MSARN: A Multi-scale Attention Residual Network for End-to-End Environmental Sound Classification
NEURAL PROCESSING LETTERS(2023)
摘要
In current end-to-end environmental sound classification model, fixed-size filters are difficult to balance the time-frequency resolution while the weight setting of each scale is hard to reflect their importances when using multi-scale filters. Therefore, an end-to-end environmental sound classification method based on multi-scale attention residual network is proposed in this paper, which make full use of attention mechanism, muti-scale fusion and residual network structure. A weighted fusion of features at different scales by attention mechanism is utilized for better feature representation. Meanwhile, the residual structure, instead of the normal one-dimensional convolution layer, is taken into account, which alleviates the problems of gradient explosion and gradient disappearance, and accelerates the model training process. Experiments on the environmental sound datasets ESC-10, ESC-50 and UrbanSound8k show that our MSARN model achieves a classification accuracy of 91.9, 79.4 and 95.4%, respectively, which is better than other mainstream end-to-end methods. Compared with the single scale model, the accuracy is improved from 8.9 to 15.2%.
更多查看译文
关键词
Environmental sound classification, Convolutional neural network, Multi-scale, End to end
AI 理解论文
溯源树
样例
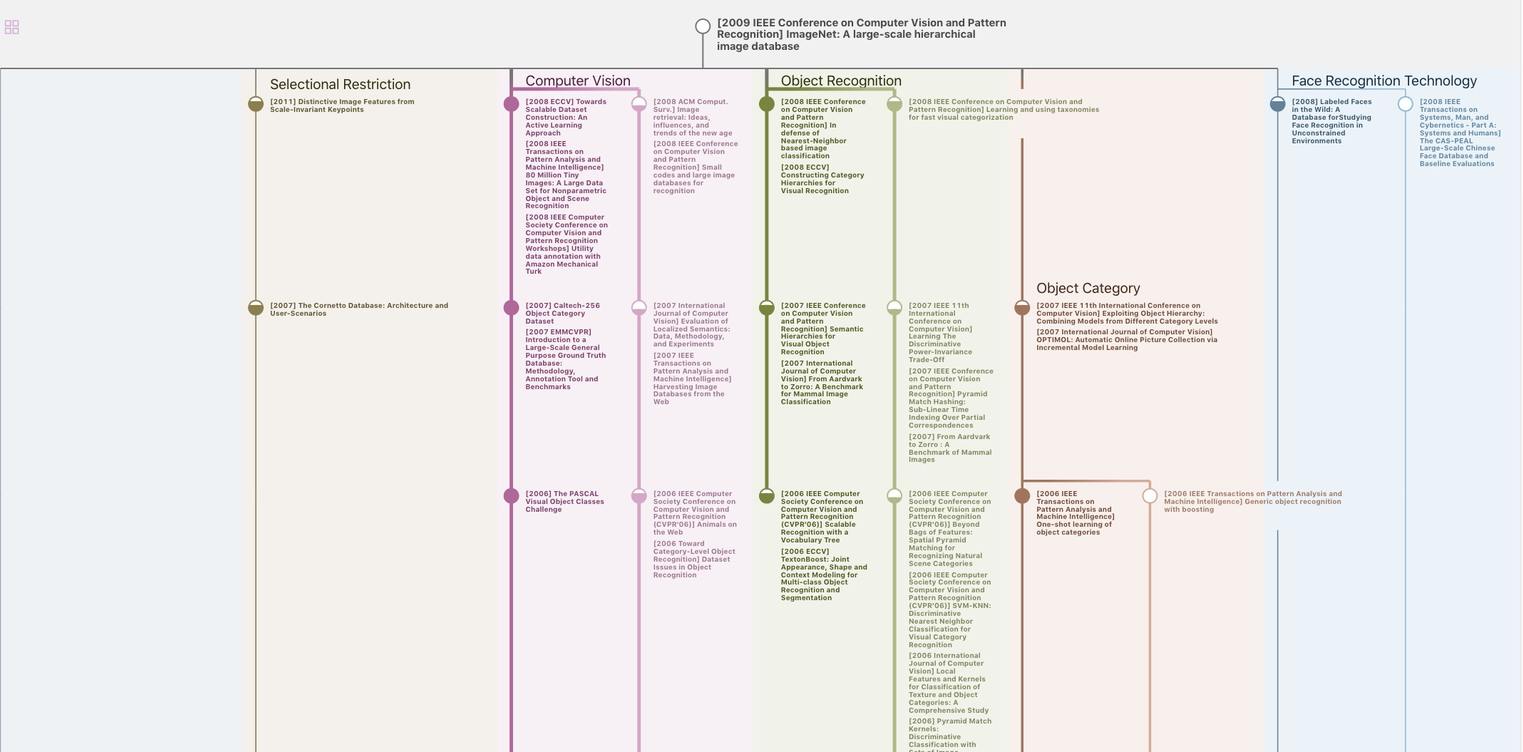
生成溯源树,研究论文发展脉络
Chat Paper
正在生成论文摘要