Improving Joint Speech-Text Representations Without Alignment
CoRR(2023)
摘要
The last year has seen astonishing progress in text-prompted image generation premised on the idea of a cross-modal representation space in which the text and image domains are represented jointly. In ASR, this idea has found application as joint speech-text encoders that can scale to the capacities of very large parameter models by being trained on both unpaired speech and text. While these methods show promise, they have required special treatment of the sequence-length mismatch inherent in speech and text, either by up-sampling heuristics or an explicit alignment model. In this work, we offer evidence that joint speech-text encoders naturally achieve consistent representations across modalities by disregarding sequence length, and argue that consistency losses could forgive length differences and simply assume the best alignment. We show that such a loss improves downstream WER in both a large-parameter monolingual and multilingual system.
更多查看译文
关键词
representations,alignment,speech-text
AI 理解论文
溯源树
样例
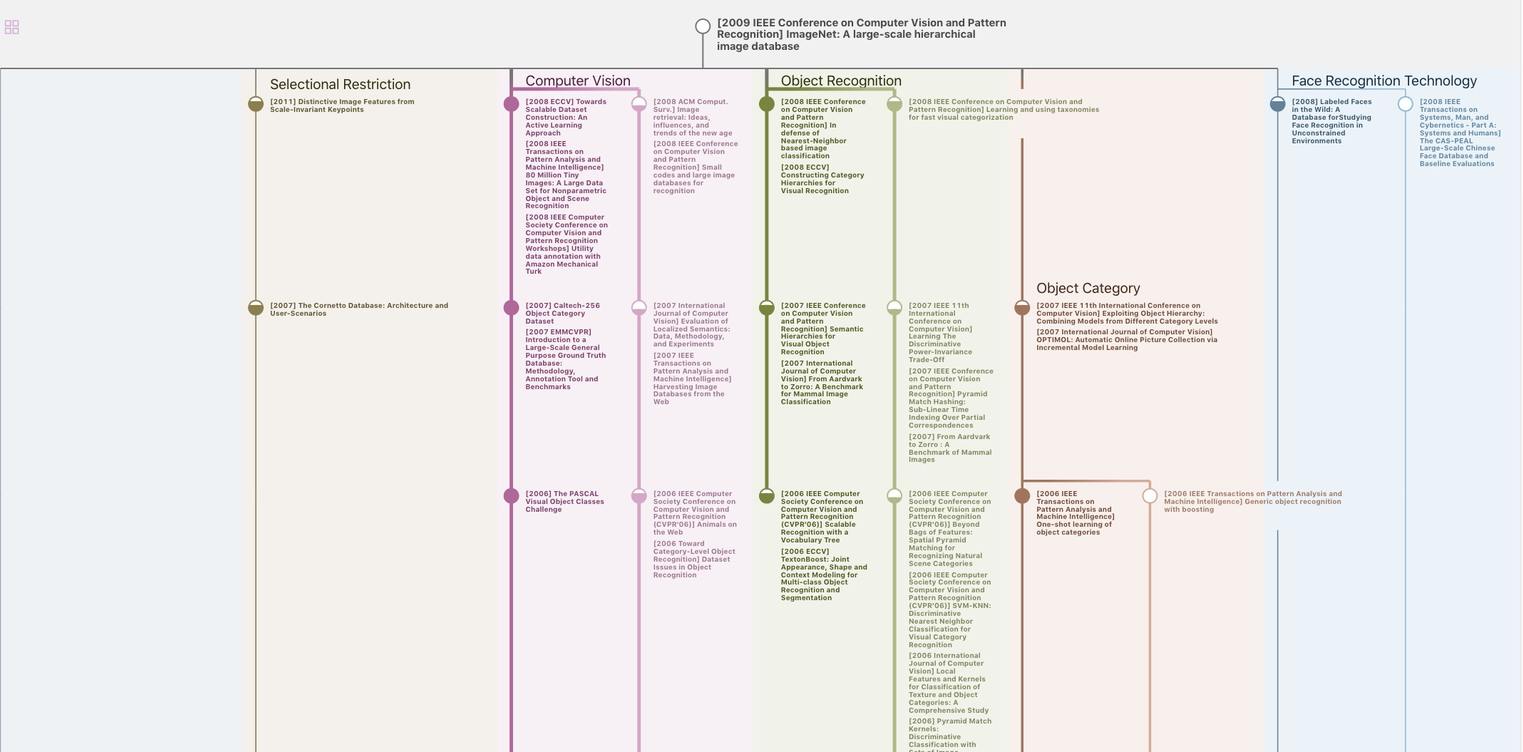
生成溯源树,研究论文发展脉络
Chat Paper
正在生成论文摘要