Toward a Better Understanding of Loss Functions for Collaborative Filtering
PROCEEDINGS OF THE 32ND ACM INTERNATIONAL CONFERENCE ON INFORMATION AND KNOWLEDGE MANAGEMENT, CIKM 2023(2023)
Abstract
Collaborative filtering (CF) is a pivotal technique in modern recommender systems. The learning process of CF models typically consists of three components: interaction encoder, loss function, and negative sampling. Although many existing studies have proposed various CF models to design sophisticated interaction encoders, recent work shows that simply reformulating the loss functions can achieve significant performance gains. This paper delves into analyzing the relationship among existing loss functions. Our mathematical analysis reveals that the previous loss functions can be interpreted as alignment and uniformity functions: (i) the alignment matches user and item representations, and (ii) the uniformity disperses user and item distributions. Inspired by this analysis, we propose a novel loss function that improves the design of alignment and uniformity considering the unique patterns of datasets called Margin-aware Alignment and Weighted Uniformity (MAWU). The key novelty of MAWU is two-fold: (i) margin-aware alignment (MA) mitigates user/item-specific popularity biases, and (ii) weighted uniformity (WU) adjusts the significance between user and item uniformities to reflect the inherent characteristics of datasets. Extensive experimental results show that MF and LightGCN equipped with MAWU are comparable or superior to state-of-the-art CF models with various loss functions on three public datasets.
MoreTranslated text
Key words
Collaborative filtering,loss function,theoretical analysis,alignment and uniformity
AI Read Science
Must-Reading Tree
Example
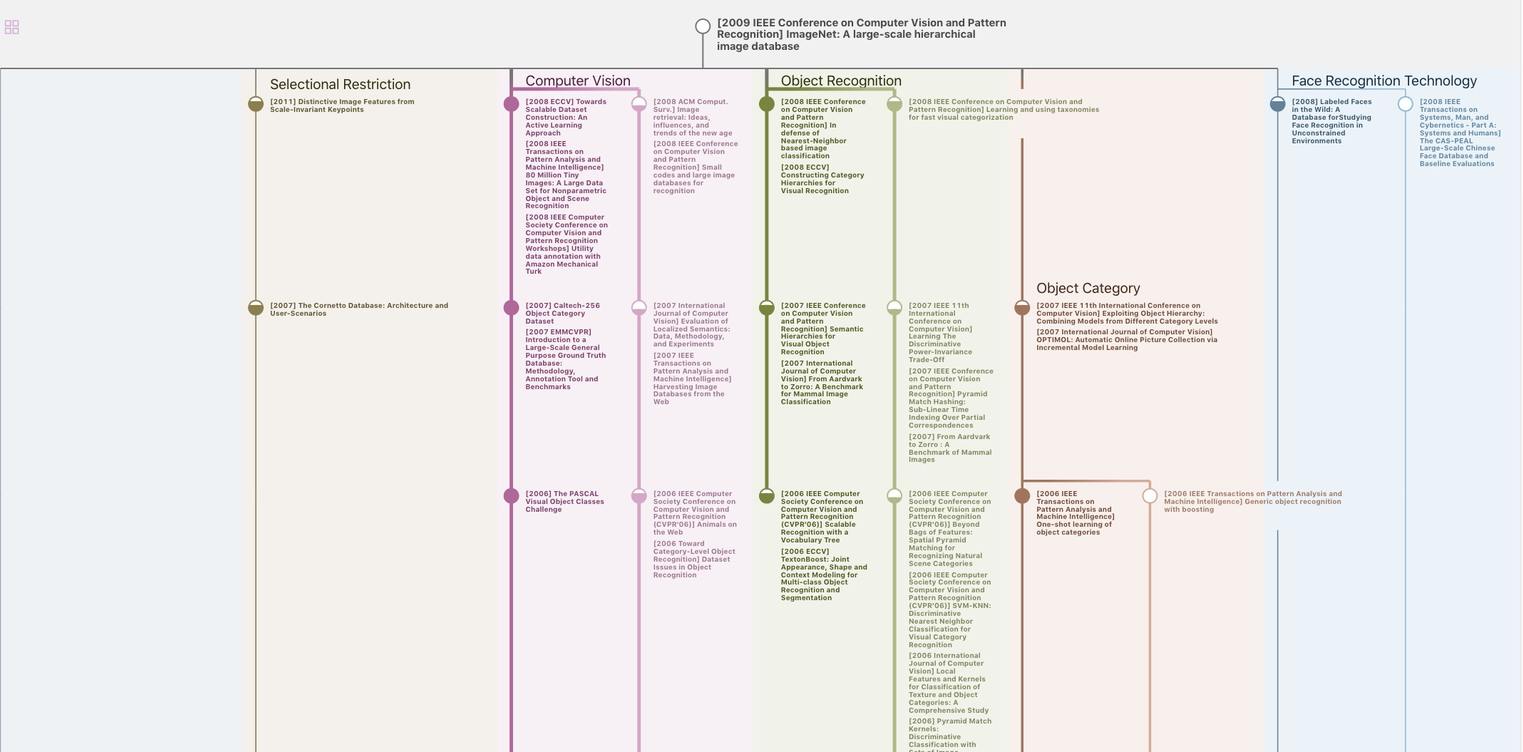
Generate MRT to find the research sequence of this paper
Chat Paper
Summary is being generated by the instructions you defined