Radiomics in the characterization of lipid-poor adrenal adenomas at unenhanced CT: time to look beyond usual density metrics
EUROPEAN RADIOLOGY(2024)
摘要
ObjectivesIn this study, we developed a radiomic signature for the classification of benign lipid-poor adenomas, which may potentially help clinicians limit the number of unnecessary investigations in clinical practice. Indeterminate adrenal lesions of benign and malignant nature may exhibit different values of key radiomics features.MethodsPatients who had available histopathology reports and a non-contrast-enhanced CT scan were included in the study. Radiomics feature extraction was done after the adrenal lesions were contoured. The primary feature selection and prediction performance scores were calculated using the least absolute shrinkage and selection operator (LASSO). To eliminate redundancy, the best-performing features were further examined using the Pearson correlation coefficient, and new predictive models were created.ResultsThis investigation covered 50 lesions in 48 patients. After LASSO-based radiomics feature selection, the test dataset's 30 iterations of logistic regression models produced an average performance of 0.72. The model with the best performance, made up of 13 radiomics features, had an AUC of 0.99 in the training phase and 1.00 in the test phase. The number of features was lowered to 5 after performing Pearson's correlation to prevent overfitting. The final radiomic signature trained a number of machine learning classifiers, with an average AUC of 0.93.ConclusionsIncluding more radiomics features in the identification of adenomas may improve the accuracy of NECT and reduce the need for additional imaging procedures and clinical workup, according to this and other recent radiomics studies that have clear points of contact with current clinical practice.Clinical relevance statementThe study developed a radiomic signature using unenhanced CT scans for classifying lipid-poor adenomas, potentially reducing unnecessary investigations that scored a final accuracy of 93%.Key Points & BULL; Radiomics has potential for differentiating lipid-poor adenomas and avoiding unnecessary further investigations.& BULL; Quadratic mean, strength, maximum 3D diameter, volume density, and area density are promising predictors for adenomas.& BULL; Radiomics models reach high performance with average AUC of 0.95 in the training phase and 0.72 in the test phase.Key Points & BULL; Radiomics has potential for differentiating lipid-poor adenomas and avoiding unnecessary further investigations.& BULL; Quadratic mean, strength, maximum 3D diameter, volume density, and area density are promising predictors for adenomas.& BULL; Radiomics models reach high performance with average AUC of 0.95 in the training phase and 0.72 in the test phase.Key Points & BULL; Radiomics has potential for differentiating lipid-poor adenomas and avoiding unnecessary further investigations.& BULL; Quadratic mean, strength, maximum 3D diameter, volume density, and area density are promising predictors for adenomas.& BULL; Radiomics models reach high performance with average AUC of 0.95 in the training phase and 0.72 in the test phase.
更多查看译文
关键词
Abdomen,Adrenocortical adenoma,Adrenal incidentaloma,X-ray computed tomography,Artificial intelligence
AI 理解论文
溯源树
样例
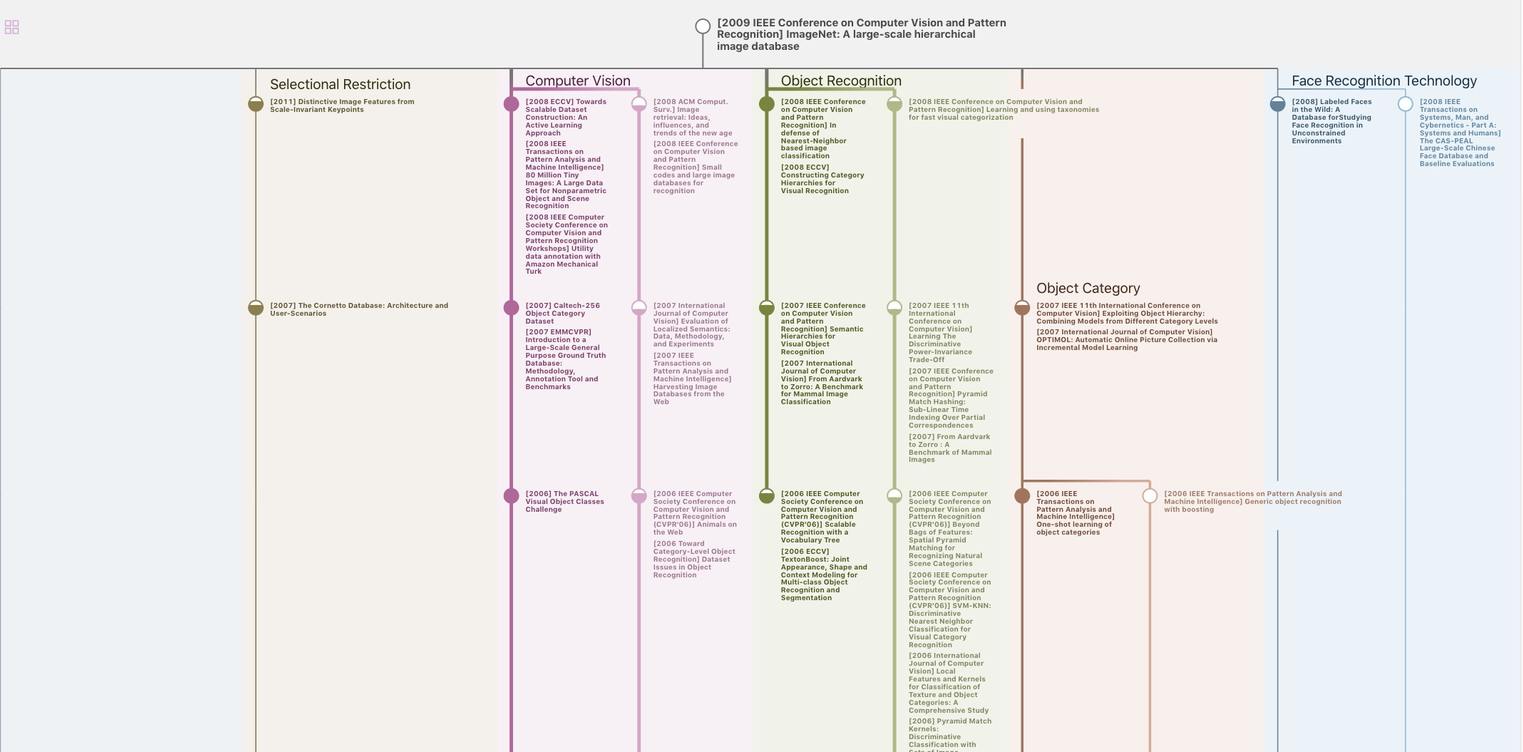
生成溯源树,研究论文发展脉络
Chat Paper
正在生成论文摘要