HSGAN: Hyperspectral Reconstruction From RGB Images With Generative Adversarial Network.
IEEE transactions on neural networks and learning systems(2023)
摘要
Hyperspectral (HS) reconstruction from RGB images denotes the recovery of whole-scene HS information, which has attracted much attention recently. State-of-the-art approaches often adopt convolutional neural networks to learn the mapping for HS reconstruction from RGB images. However, they often do not achieve high HS reconstruction performance across different scenes consistently. In addition, their performance in recovering HS images from clean and real-world noisy RGB images is not consistent. To improve the HS reconstruction accuracy and robustness across different scenes and from different input images, we present an effective HSGAN framework with a two-stage adversarial training strategy. The generator is a four-level top-down architecture that extracts and combines features on multiple scales. To generalize well to real-world noisy images, we further propose a spatial-spectral attention block (SSAB) to learn both spatial-wise and channel-wise relations. We conduct the HS reconstruction experiments from both clean and real-world noisy RGB images on five well-known HS datasets. The results demonstrate that HSGAN achieves superior performance to existing methods. Please visit https://github.com/zhaoyuzhi/HSGAN to try our codes.
更多查看译文
关键词
Generative adversarial network (GAN),hyperspectral (HS) reconstruction,spatial–spectral attention
AI 理解论文
溯源树
样例
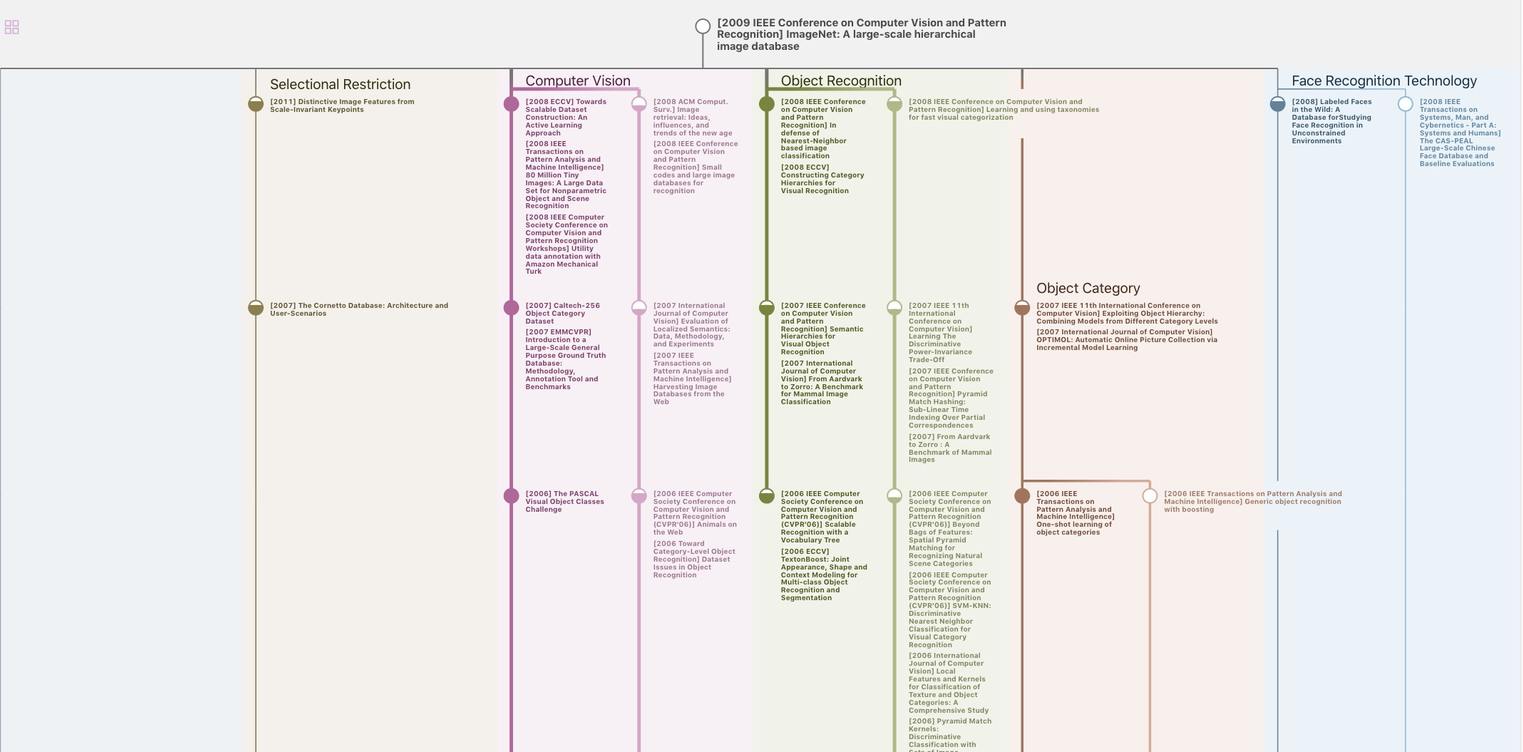
生成溯源树,研究论文发展脉络
Chat Paper
正在生成论文摘要