Soil friction coefficient estimation using CNN included in an assistive system for walking in urban areas
J. Ambient Intell. Humaniz. Comput.(2023)
摘要
We present a smartphone-based solution to help visually impaired people autonomously navigate in urban environments. Contrary to previous works in the literature, the newly proposed system in this paper aims to determine the coefficient of friction (COF) of a soil for aiding in the safe movement of blind and visually impaired people (BVIP). Through convolutional neural networks (CNNs) trained to output this measure, our new investigation then offers the possibility of predicting a risk of falling in their next step by determining the maximum static friction force of the ground. Indeed, a commercial smartphone’s camera captures the video of the ground and sends frame as inputs to the CNN model for image segmentation and COF computation. We validated our proposed model in real experiments carried out on 8 types of soils, while also experimenting different CNN models and different optimizers. The proposed ResNet50 CNN-based system provides an accuracy of 96% in the classification of soil type, enabling to guide visually impaired persons. Combined with the associated COF of the soil type, it’s possible to estimate a risk of fall (stick or slip) for the next step in the front of the user from the past measured interaction force (using an instrumented insole) between the soil and the sole. Traditional navigation approaches do not consider the soil characteristics such as COF to guide blind and visually impaired person.
更多查看译文
关键词
Smartphone,Risk of fall,Deep learning,Computer vision
AI 理解论文
溯源树
样例
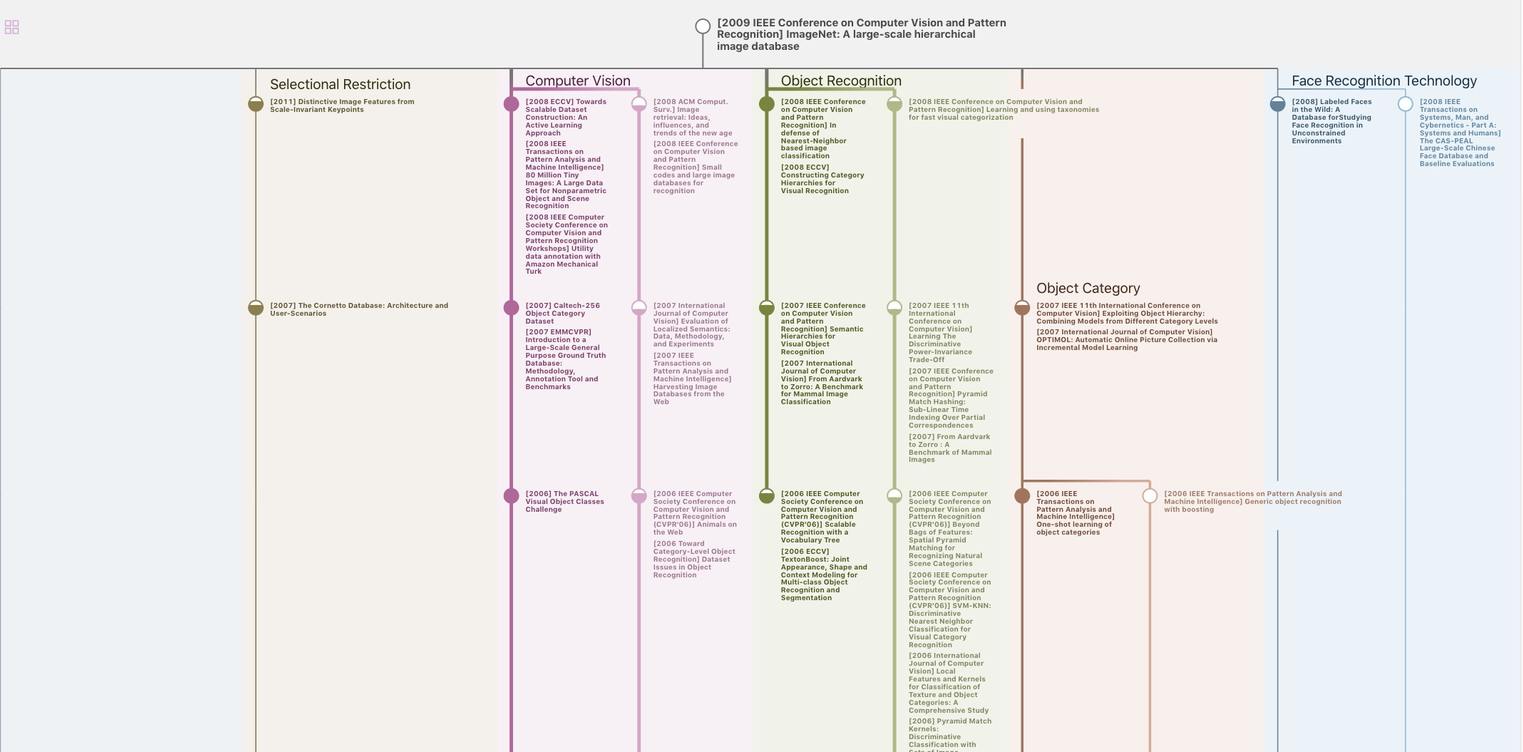
生成溯源树,研究论文发展脉络
Chat Paper
正在生成论文摘要