Assessing the simulation of streamflow with the LSTM model across the continental United States using the MOPEX dataset
Neural Comput. Appl.(2023)
摘要
This study aims to assess the spatiotemporal performance of Machine Learning-based techniques for simulating streamflow on a continental scale using Long-Sort Term Memory (LSTM) models. The dataset employed is derived from the Model Parameter Estimation Experiment (MOPEX), encompassing 438 watersheds across the US. MOPEX has the longest data record (55 years) compared to other datasets which makes it very suitable for LSTM training. The impact of incorporating vegetation Greenness Fraction (GF) in the LSTM GF model was assessed. To gauge the models’ performance, temporally and spatially, a range of assessment metrics were employed. Upon the integration of GF, the LSTM models either maintained or enhanced streamflow simulation across the US, contingent upon the watershed location and seasonal variations. Notably, the overall median KGE and Percent Bias (PB) values with the inclusion of GF were 0.723 and 4.09, in contrast to 0.717 and 4.94 without the incorporation of GF. In addition, the results indicated that LSTM GF had superior performance compared to LSTM in areas where there was significant seasonal variation in vegetation cover. Results show that using extensive data record (MOPEX) bolstered the performance of LSTM with a Kling-Gupta Efficiency (KGE) reaching up to 0.97 at certain stations compared to only 0.86 when 25 years are used for the training as it is the case of the Catchment Attributes and Meteorology for Large-sample Studies (CAMELS) dataset. These findings corroborate the potential for integrating LSTM models into continental scale hydrological models such as the NOAA NextGen National Water Model.
更多查看译文
关键词
LSTM,Drainage basin,Streamflow,MOPEX,Greenness fraction,Regionalization
AI 理解论文
溯源树
样例
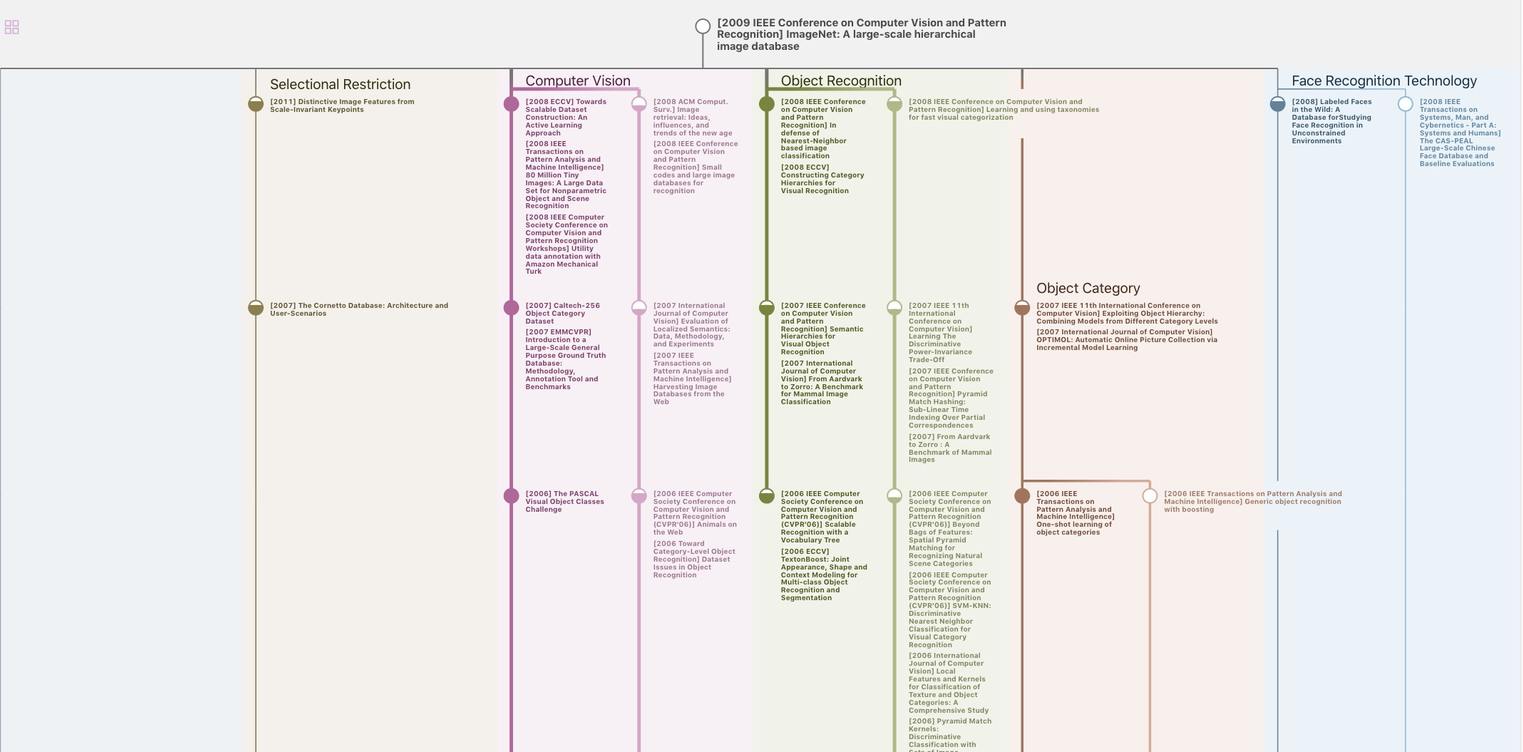
生成溯源树,研究论文发展脉络
Chat Paper
正在生成论文摘要