Ab initio quantum chemistry with neural-network wavefunctions
Nature Reviews Chemistry(2023)
Abstract
Deep learning methods outperform human capabilities in pattern recognition and data processing problems and now have an increasingly important role in scientific discovery. A key application of machine learning in molecular science is to learn potential energy surfaces or force fields from ab initio solutions of the electronic Schrödinger equation using data sets obtained with density functional theory, coupled cluster or other quantum chemistry (QC) methods. In this Review, we discuss a complementary approach using machine learning to aid the direct solution of QC problems from first principles. Specifically, we focus on quantum Monte Carlo methods that use neural-network ansatzes to solve the electronic Schrödinger equation, in first and second quantization, computing ground and excited states and generalizing over multiple nuclear configurations. Although still at their infancy, these methods can already generate virtually exact solutions of the electronic Schrödinger equation for small systems and rival advanced conventional QC methods for systems with up to a few dozen electrons.
MoreTranslated text
Key words
initio quantum chemistry,neural-network neural-network
AI Read Science
Must-Reading Tree
Example
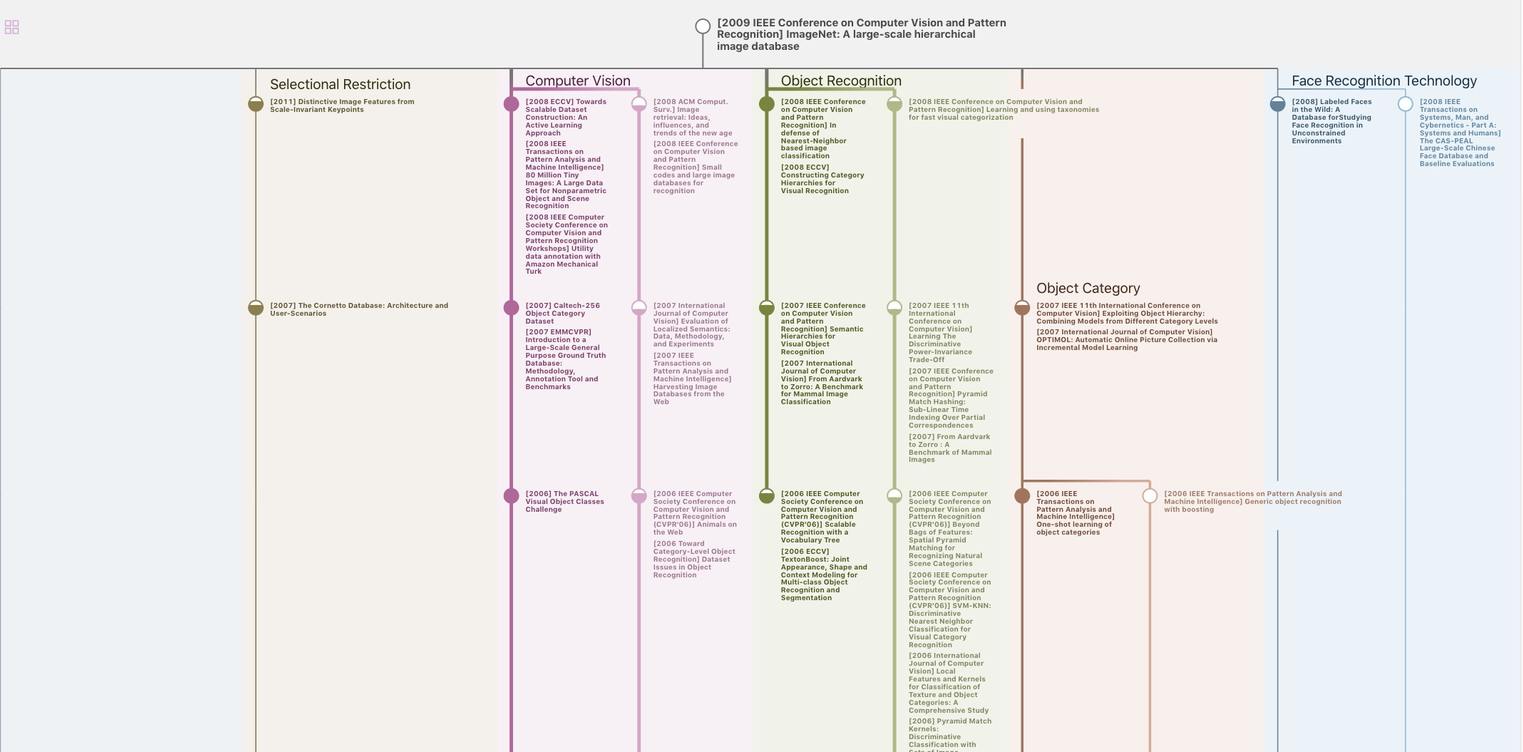
Generate MRT to find the research sequence of this paper
Chat Paper
Summary is being generated by the instructions you defined