Online solving Nash equilibrium solution of N -player nonzero-sum differential games via recursive least squares
SOFT COMPUTING(2023)
摘要
A novel online critical neural network weight adjustment algorithm is proposed in this paper by combining policy iteration and recursive least squares (RLSs) to address the problem of optimal control of players in nonlinear systems with nonzero-sum games. The interaction between players and the nonlinearity of the system make it difficult to solve the Hamiltonian function directly. From a linear regression perspective, this paper regards any admissible control and its corresponding value function as the input and output affected by perturbations. By using RLS to process the current data and store the historical data’s covariance matrix to adjust the weights, the calculation is greatly simplified by avoiding the space waste caused by a lot of historical data storage and the time waste caused by data collection. When calculating the cumulative error, a discount factor is introduced to avoid the total error value tending to infinity and the effect of historical policy evaluation, where the covariance matrix tends to zero and loses its adjustment effect. When the error of the Hamiltonian equation is zero, the proposed adjustment law will also tend to zero. After that, the stability of the covariance matrix was analysed as well as the convergence of the weight errors was proved. Finally, two simulations are conducted to verify the effectiveness of the proposed algorithm based on the RLS method for solving the Nash equilibrium solution online.
更多查看译文
关键词
Neural network (NN),Recursive least squares (RLS),Reinforcement learning (RL),Nonzero-sum game (NZS)
AI 理解论文
溯源树
样例
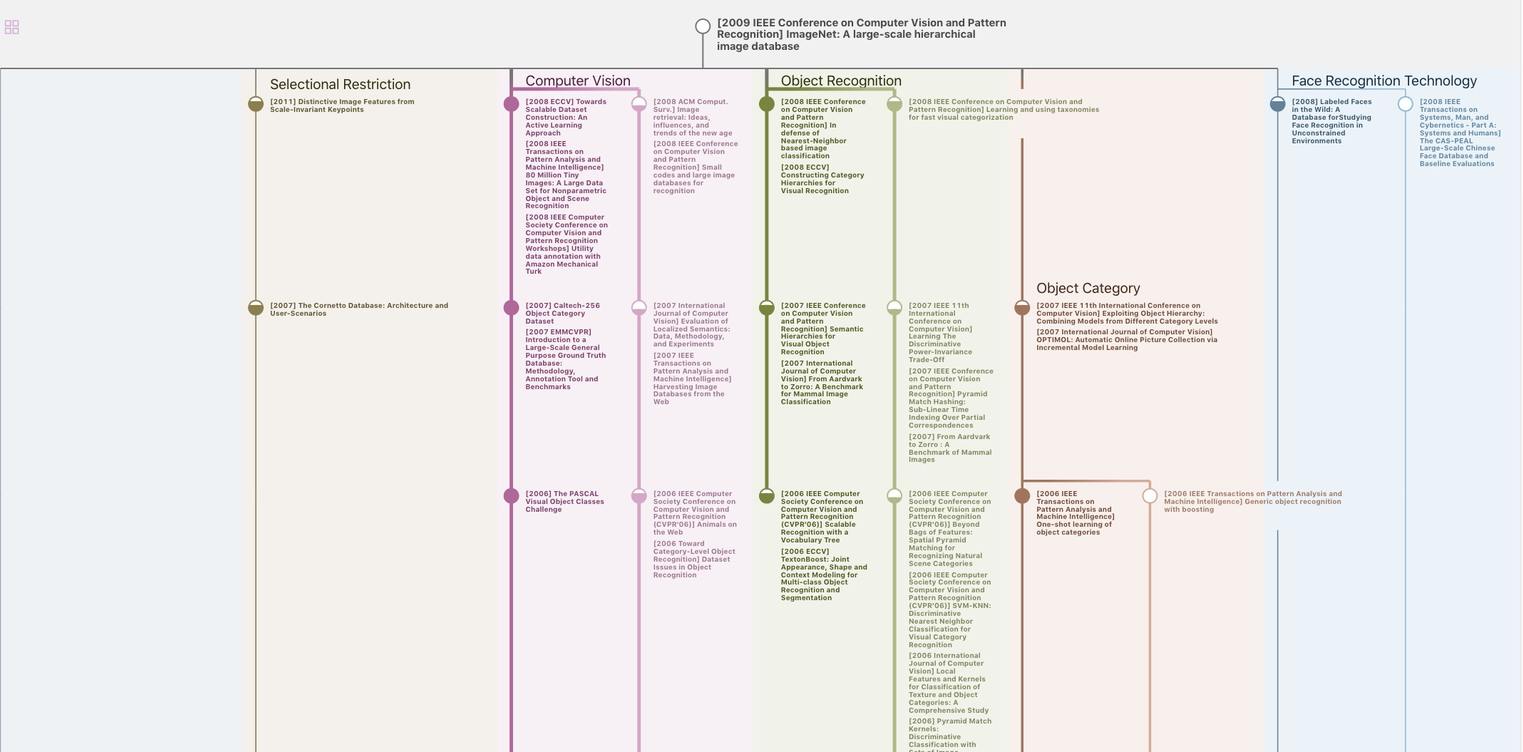
生成溯源树,研究论文发展脉络
Chat Paper
正在生成论文摘要