Deep learning discrimination of rheumatoid arthritis from osteoarthritis on hand radiography
SKELETAL RADIOLOGY(2024)
摘要
Purpose To develop a deep learning model to distinguish rheumatoid arthritis (RA) from osteoarthritis (OA) using hand radiographs and to evaluate the effects of changing pretraining and training parameters on model performance. Materials and methods A convolutional neural network was retrospectively trained on 9714 hand radiograph exams from 8387 patients obtained from 2017 to 2021 at seven hospitals within an integrated healthcare network. Performance was assessed using an independent test set of 250 exams from 146 patients. Binary discriminatory capacity (no arthritis versus arthritis; RA versus not RA) and three-way classification (no arthritis versus OA versus RA) were evaluated. The effects of additional pretraining using musculoskeletal radiographs, using all views as opposed to only the posteroanterior view, and varying image resolution on model performance were also investigated. Area under the receiver operating characteristic curve (AUC) and Cohen’s kappa coefficient were used to evaluate diagnostic performance. Results For no arthritis versus arthritis, the model achieved an AUC of 0.975 (95% CI: 0.957, 0.989). For RA versus not RA, the model achieved an AUC of 0.955 (95% CI: 0.919, 0.983). For three-way classification, the model achieved a kappa of 0.806 (95% CI: 0.742, 0.866) and accuracy of 87.2% (95% CI: 83.2%, 91.2%) on the test set. Increasing image resolution increased performance up to 1024 × 1024 pixels. Additional pretraining on musculoskeletal radiographs and using all views did not significantly affect performance. Conclusion A deep learning model can be used to distinguish no arthritis, OA, and RA on hand radiographs with high performance.
更多查看译文
关键词
Deep learning,Hand radiography,Osteoarthritis,Rheumatoid arthritis,Model parameters
AI 理解论文
溯源树
样例
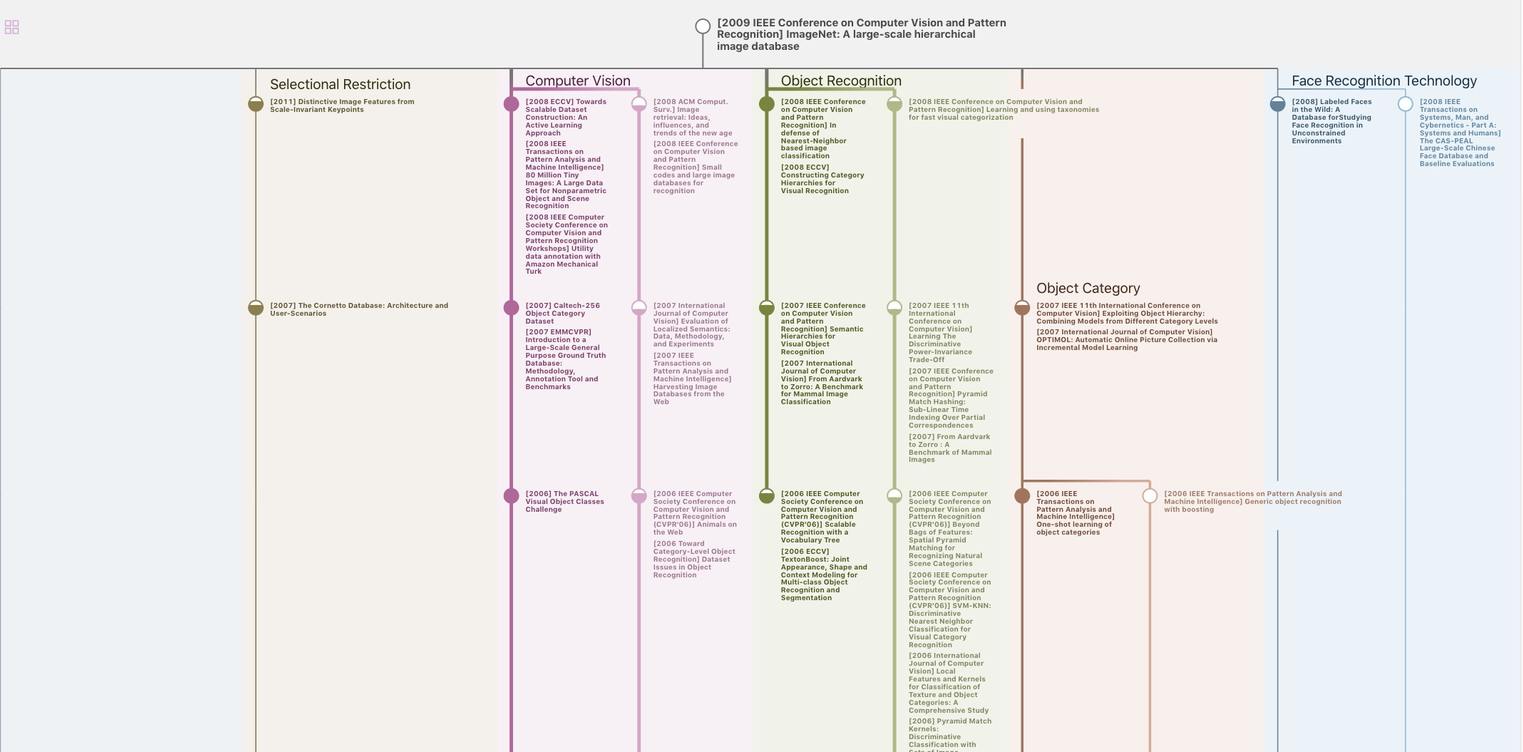
生成溯源树,研究论文发展脉络
Chat Paper
正在生成论文摘要