On-line evolutionary identification technology for milling chatter of thin walled parts based on the incremental-sparse K-means and the online sequential extreme learning machine
INTERNATIONAL JOURNAL OF ADVANCED MANUFACTURING TECHNOLOGY(2023)
Abstract
In the milling process of thin-walled parts, chatter is very easy to occur, which has a very adverse impact on the surface quality and machining efficiency of the workpiece. In order to solve the problem of low accuracy of milling state identification caused by few initial samples and dynamic changes in the milling process, a hybrid online evolutionary chatter identification model combining unsupervised learning and supervised learning was proposed. First of all, aiming at the problem that traditional K-means algorithm is difficult to adapt to online dynamic clustering of milling chatter, an online incremental-sparse K-means algorithm (ISK-Means) was proposed, and the dynamic incremental-sparse strategy of K-means was designed. Second, aiming at the problem that the online sequential extreme learning machine (OS-ELM) algorithm directly adds its predicted samples to the training sample set during the incremental learning process, and the pseudo-samples in the training sample set would lead to the degradation of the OS-ELM model, a hybrid online evolutionary chatter identification model combining the ISK-means and the OS-ELM was proposed, and the online identification and evolution strategy was designed. Finally, the experimental results show that the ISK-means algorithm can greatly improve the clustering efficiency and is suitable for milling chatter online dynamic clustering. Meanwhile, compared with the existing model, the recognition accuracy of the hybrid online evolutionary chatter recognition model combined with ISK-means algorithm and OS-ELM algorithm is improved by 1.31%. This is of great significance for the online control of subsequent chatter.
MoreTranslated text
Key words
Milling chatter,Milling state identification,Incremental-sparse K-means,Online sequential extreme learning machine,Online evolutionary model
AI Read Science
Must-Reading Tree
Example
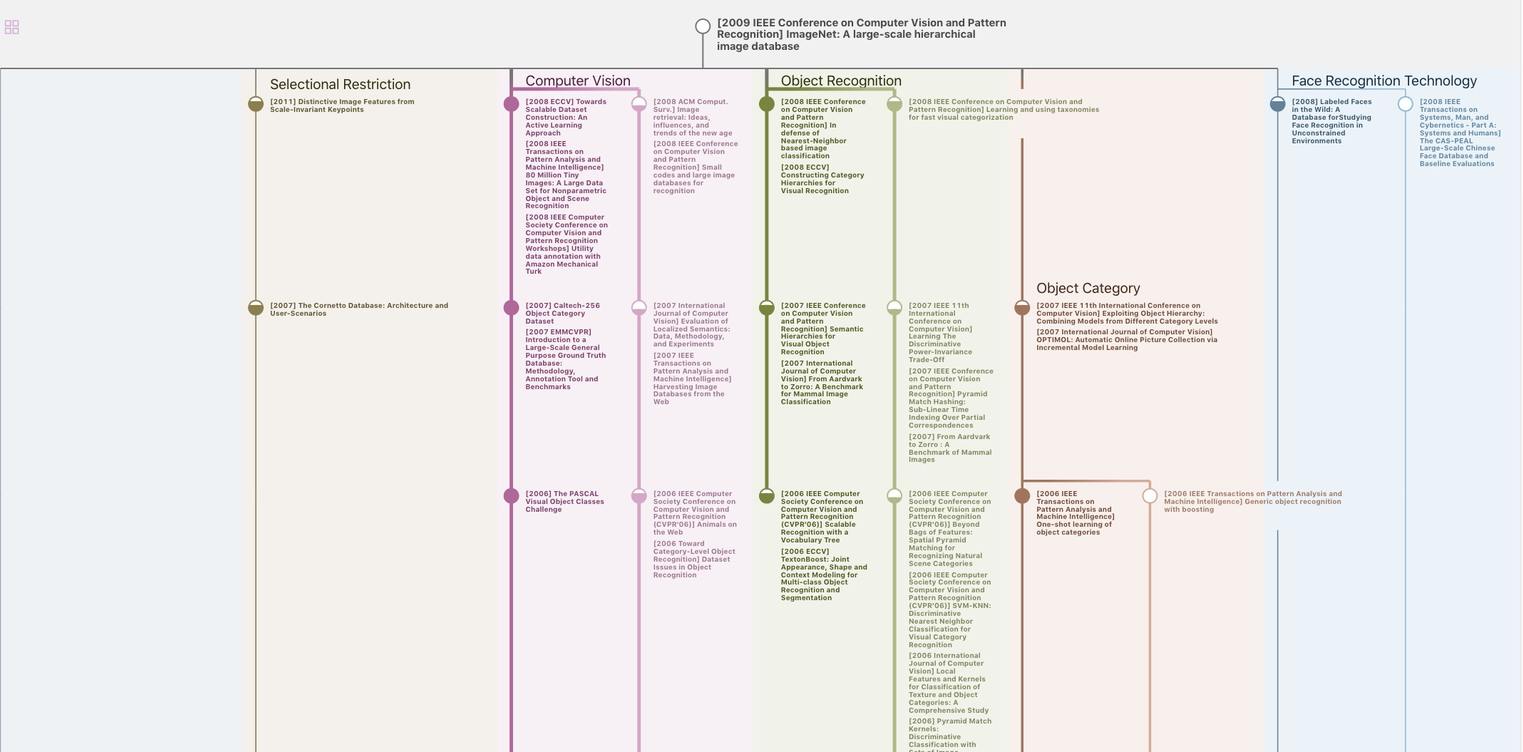
Generate MRT to find the research sequence of this paper
Chat Paper
Summary is being generated by the instructions you defined