Variance reduced moving balls approximation method for smooth constrained minimization problems
Optimization Letters(2023)
摘要
In this paper, we consider the problem of minimizing the sum of a large number of smooth convex functions subject to a complicated constraint set defined by a smooth convex function. Such a problem has wide applications in many areas, such as machine learning and signal processing. By utilizing variance reduction and moving balls approximation techniques, we propose a new variance reduced moving balls approximation method. Compared with existing convergence rates of moving balls approximation-type methods that require the strong convexity of the objective function, a notable advantage of the proposed method is that the linear and sublinear convergence rates can be guaranteed under the quadratic gradient growth property and convexity condition, respectively. To demonstrate its effectiveness, numerical experiments for solving the smooth regularized logistic regression problem and the Neyman-Pearson classification problem are presented.
更多查看译文
关键词
Smooth constrained minimization,Variance reduction,Moving balls approximation,Relaxed strong convexity condition,Convergence rate
AI 理解论文
溯源树
样例
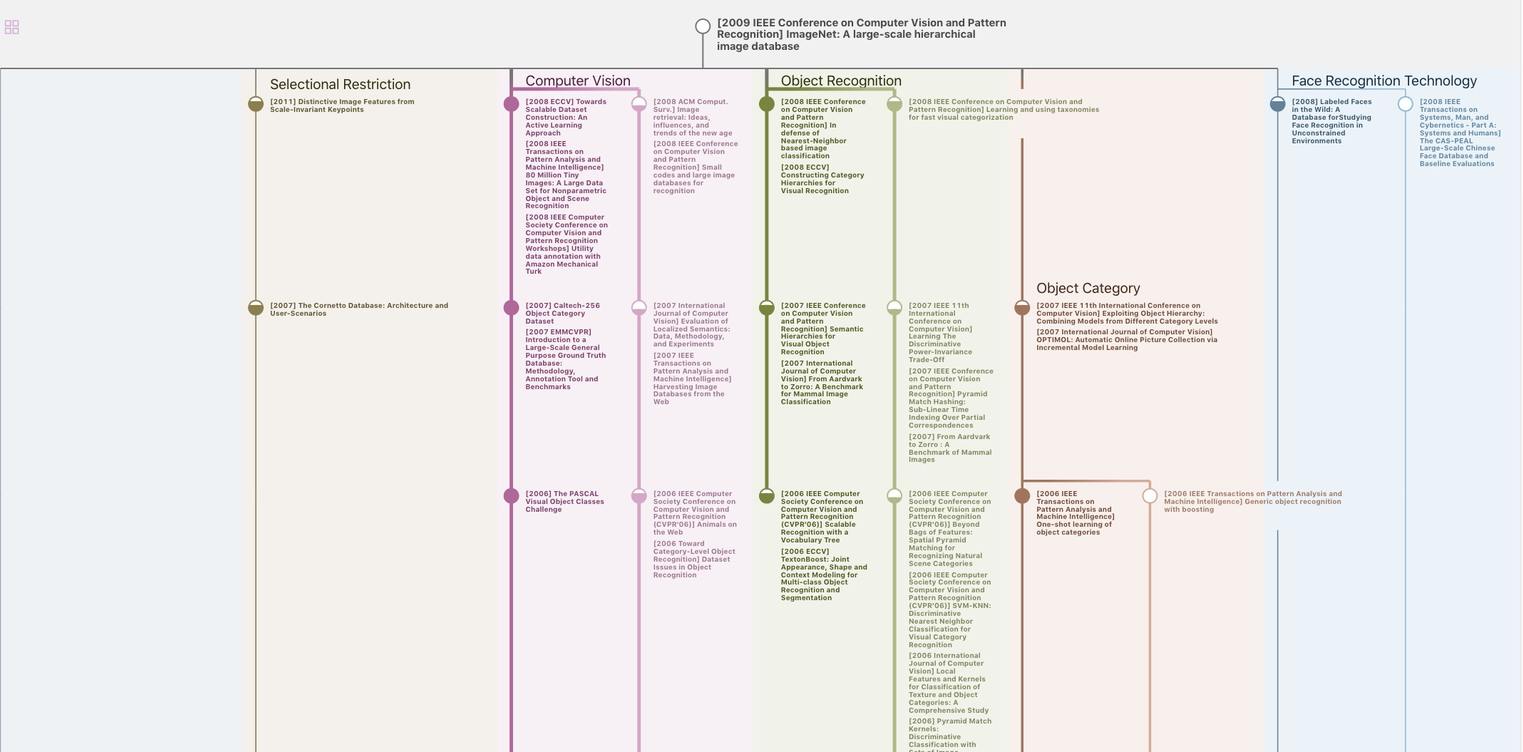
生成溯源树,研究论文发展脉络
Chat Paper
正在生成论文摘要