An improved ID3 algorithm based on variable precision neighborhood rough sets
APPLIED INTELLIGENCE(2023)
摘要
The classical ID3 decision tree algorithm cannot directly handle continuous data and has a poor classification effect. Moreover, most of the existing approaches use a single mechanism for node measurement, which is unfavorable for the construction of decision trees. In order to solve the above problems, we propose an improved ID3 algorithm (called DIGGI) based on variable precision neighborhood rough sets. First, we introduce the notions of variable precision neighborhood (VPN) equivalence relation and VPN equivalence granule, and probe some basic properties of these notions. Second, we give the model of VPN rough sets and propose two extended measures: the VPN information gain and the VPN Gini index. Finally, we construct a hybrid measure by using the VPN dependence to combine the above two extended measures, and adopt the VPN equivalence granule as the splitting rule of DIGGI. Experimental results show that DIGGI is effective and its accuracy is greatly improved compared with three traditional decision tree algorithms, the neighborhood decision tree (NDT) and variable precision neighborhood decision tree (VPNDT) proposed in the latest literature.
更多查看译文
关键词
improved id3 algorithm,rough sets,variable precision neighborhood
AI 理解论文
溯源树
样例
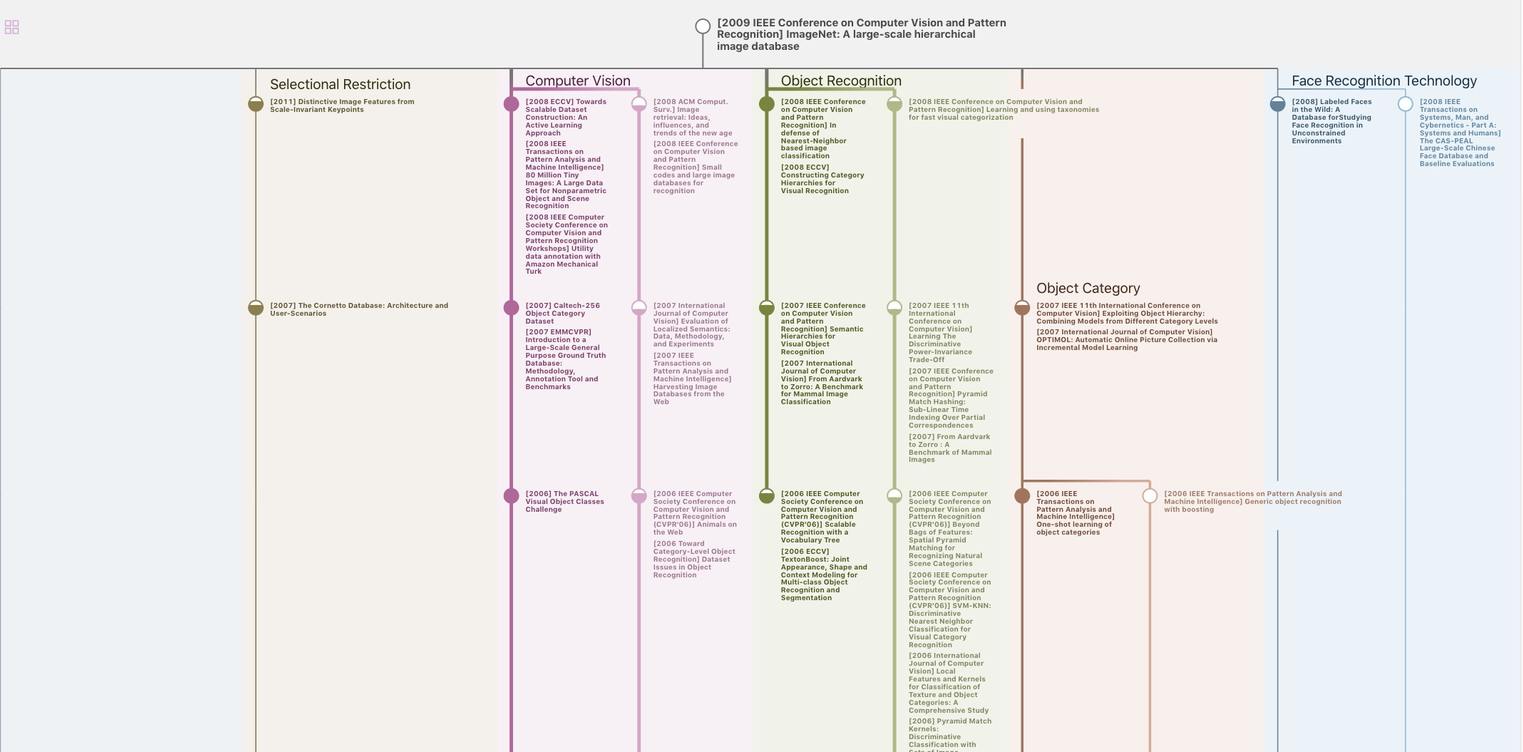
生成溯源树,研究论文发展脉络
Chat Paper
正在生成论文摘要