Language model-accelerated deep symbolic optimization
NEURAL COMPUTING & APPLICATIONS(2023)
摘要
Symbolic optimization methods have been used to solve varied challenging and relevant problems such as symbolic regression and neural architecture search. However, the current state of the art typically learns each problem from scratch and is unable to leverage pre-existing knowledge and datasets that are available for many applications. Inspired by the similarity between sequence representations learned in natural language processing and the formulation of symbolic optimization as a discrete sequence optimization problem, we propose language model-accelerated deep symbolic optimization (LA-DSO), a method that leverages language models to learn symbolic optimization solutions more efficiently. We demonstrate LA-DSO in two tasks: symbolic regression, which allows us to perform extensive experimentation due to its low computation requirements, and computational antibody optimization, which shows that our proposal accelerates learning in challenging real-world problems.
更多查看译文
关键词
Transfer learning,Reinforcement learning,Discrete optimization,BERT
AI 理解论文
溯源树
样例
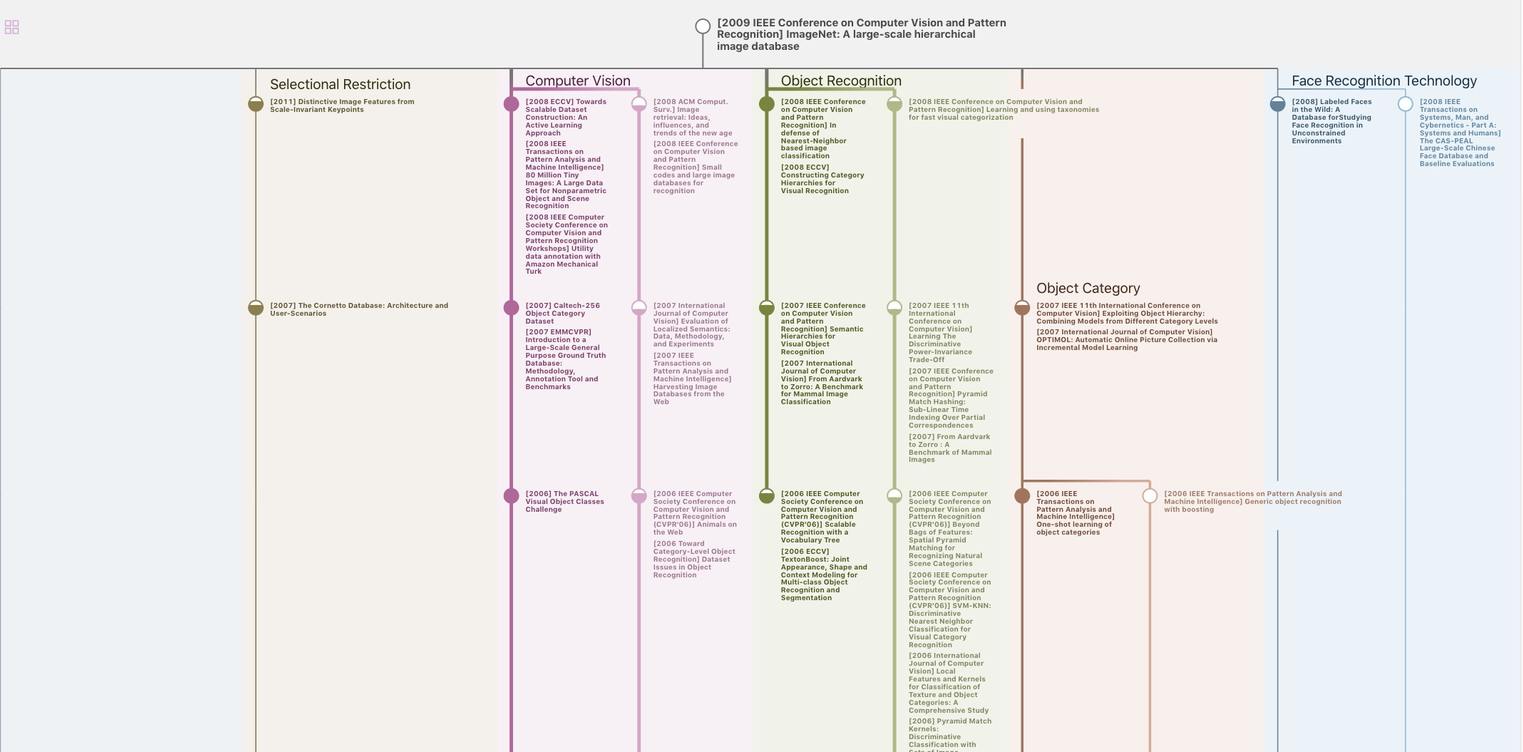
生成溯源树,研究论文发展脉络
Chat Paper
正在生成论文摘要