A dynamic programming-based data-adaptive information granulation approach and its distributed implementation
APPLIED INTELLIGENCE(2023)
摘要
Information granules are fundamental and effective constructs in human cognitive and decision-making activities. It is unavoidable to automatically describe and extract some valuable information from large-scale datasets. In this study, we suggest a data-adaptive information granulation method to reinforce the data description mechanisms. First, a dynamic programming-based attribute-value clustering method is proposed to adaptively partition the numeric attribute values to several optimal data fragments for different condition attributes. Then, some interval information granules are constructed from the clustered data fragments by the principle of justifiable granularity for some specific tasks in machine learning. Furthermore, we introduce the distributed implementation in the framework of Map-Reduce to overcome some restrictions in large-scale datasets. The experiments on several benchmark datasets are provided to demonstrate the adaptivity on different datasets and the feasibility in machine learning tasks. Furthermore, the distributed performance are tested on some artificial datasets. Graphical Abstract (left).figa
更多查看译文
关键词
Information granules,Big data,Machine learning,Map-reduce
AI 理解论文
溯源树
样例
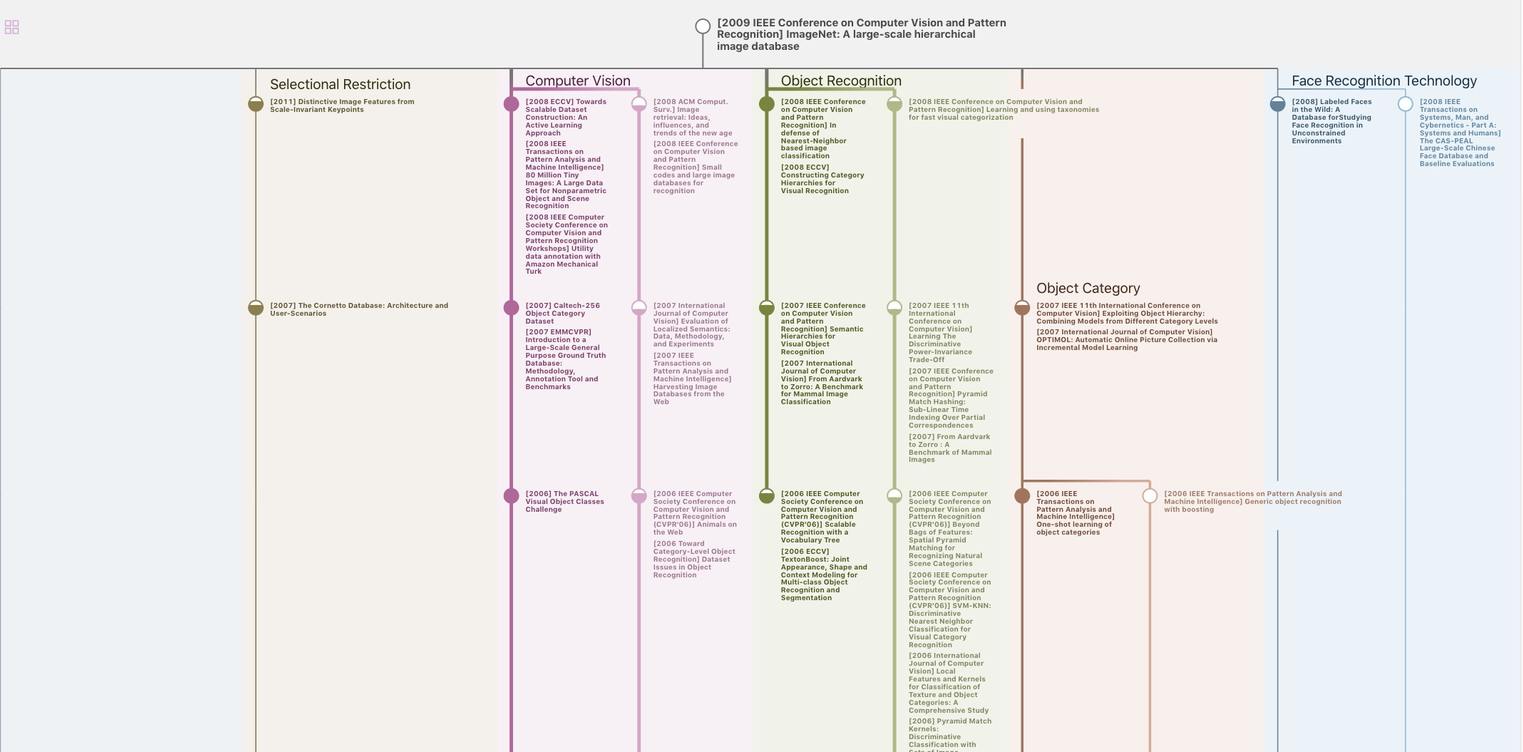
生成溯源树,研究论文发展脉络
Chat Paper
正在生成论文摘要