Evaluation of response to neoadjuvant chemotherapy in osteosarcoma using dynamic contrast-enhanced MRI: development and external validation of a model
Skeletal Radiology(2024)
摘要
Objective To identify which dynamic contrast-enhanced (DCE-)MRI features best predict histological response to neoadjuvant chemotherapy in patients with an osteosarcoma. Methods Patients with osteosarcoma who underwent DCE-MRI before and after neoadjuvant chemotherapy prior to resection were retrospectively included at two different centers. Data from the center with the larger cohort (training cohort) was used to identify which method for region-of-interest selection (whole slab or focal area method) and which change in DCE-MRI features (time to enhancement, wash-in rate, maximum relative enhancement and area under the curve) gave the most accurate prediction of histological response. Models were created using logistic regression and cross-validated. The most accurate model was then externally validated using data from the other center (test cohort). Results Fifty-five (27 poor response) and 30 (19 poor response) patients were included in training and test cohorts, respectively. Intraclass correlation coefficient of relative DCE-MRI features ranged 0.81–0.97 with the whole slab and 0.57–0.85 with the focal area segmentation method. Poor histological response was best predicted with the whole slab segmentation method using a single feature threshold, relative wash-in rate <2.3. Mean accuracy was 0.85 (95%CI: 0.75–0.95), and area under the receiver operating characteristic curve (AUC-index) was 0.93 (95%CI: 0.86–1.00). In external validation, accuracy and AUC-index were 0.80 and 0.80. Conclusion In this study, a relative wash-in rate of <2.3 determined with the whole slab segmentation method predicted histological response to neoadjuvant chemotherapy in osteosarcoma. Consistent performance was observed in an external test cohort.
更多查看译文
关键词
osteosarcoma,neoadjuvant chemotherapy,mri,contrast-enhanced
AI 理解论文
溯源树
样例
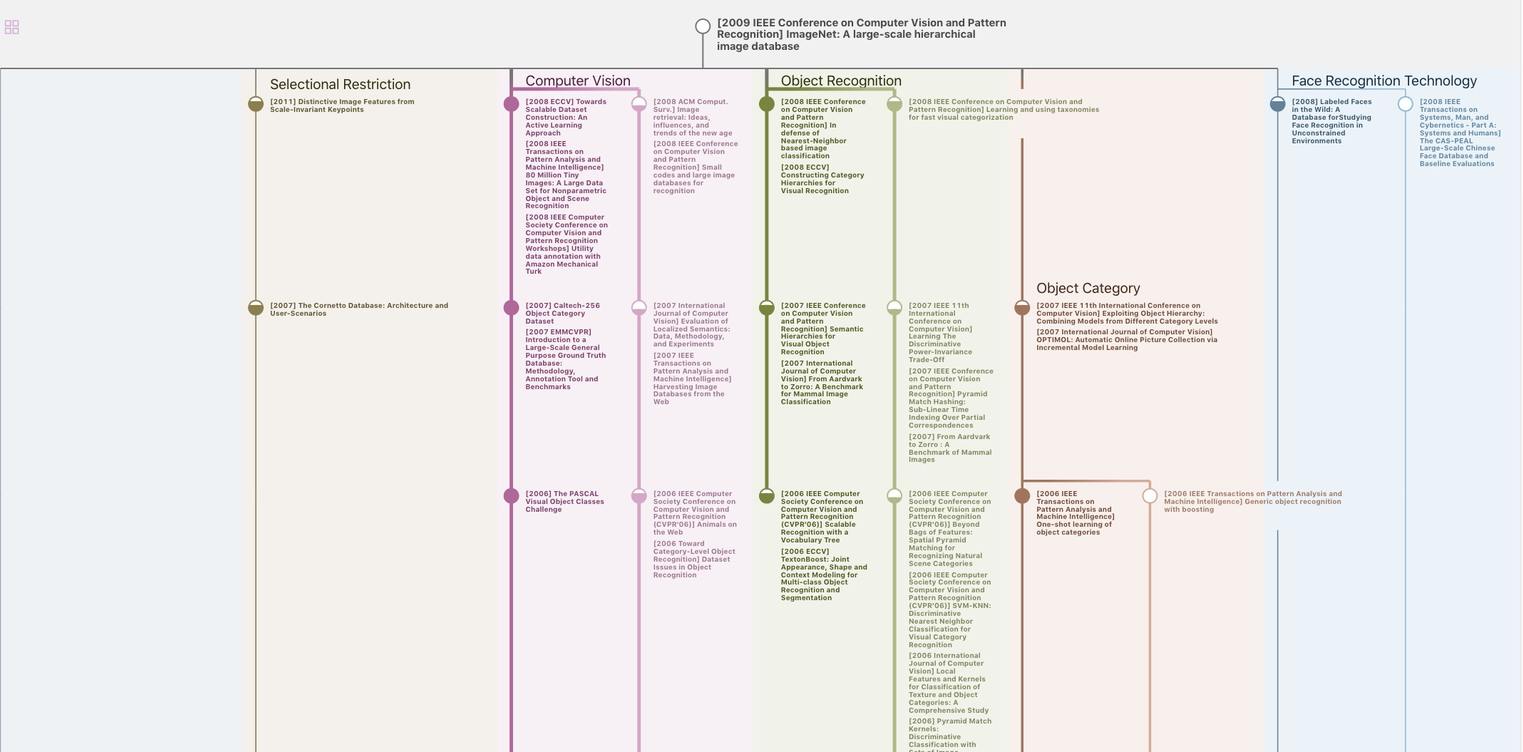
生成溯源树,研究论文发展脉络
Chat Paper
正在生成论文摘要