Convolutional neural networks tamper detection and location based on fragile watermarking
APPLIED INTELLIGENCE(2023)
摘要
With the wide application of neural network, the trained neural network model has become an important asset to provide services for users, but it also faces the risk of malicious attack or illegal tampering. Therefore, especially in safety-critical fields such as military, medical, transportation, and legal, it is crucial to provide users with an integrity authentication mechanism. In this paper, we propose a method for tamper detection and location of convolutional neural networks based on fragile watermarking, which makes it possible to recover the original model as much as possible with the help of existing intact data. Specifically, we use the HRank-based neural network pruning method and the characteristics of single precision floating-point numbers to construct the host sequence, and use the block histogram shift method to embed the watermarking information. To ensure the security of the additional information required to extract the watermarking, we encrypt it using the Combined Logistic Tent Map algorithm. At the receiving end, only the authorized owner can extract the watermarking information from the marked model, and use the characteristics of Merkle Hash Tree to achieve efficient integrity authentication and fast tamper location. To demonstrate the effectiveness of the proposed method, we conduct experiments on two datasets using multiple pre-trained models. The results show that the embedded fragile watermarking can not only realize the integrity authentication of the model, but also realize the authorization verification and tamper location of the model without affecting the classification performance of the model.
更多查看译文
关键词
Convolutional neural networks,Fast tamper location,Fragile watermarking,Authorization verification,Security
AI 理解论文
溯源树
样例
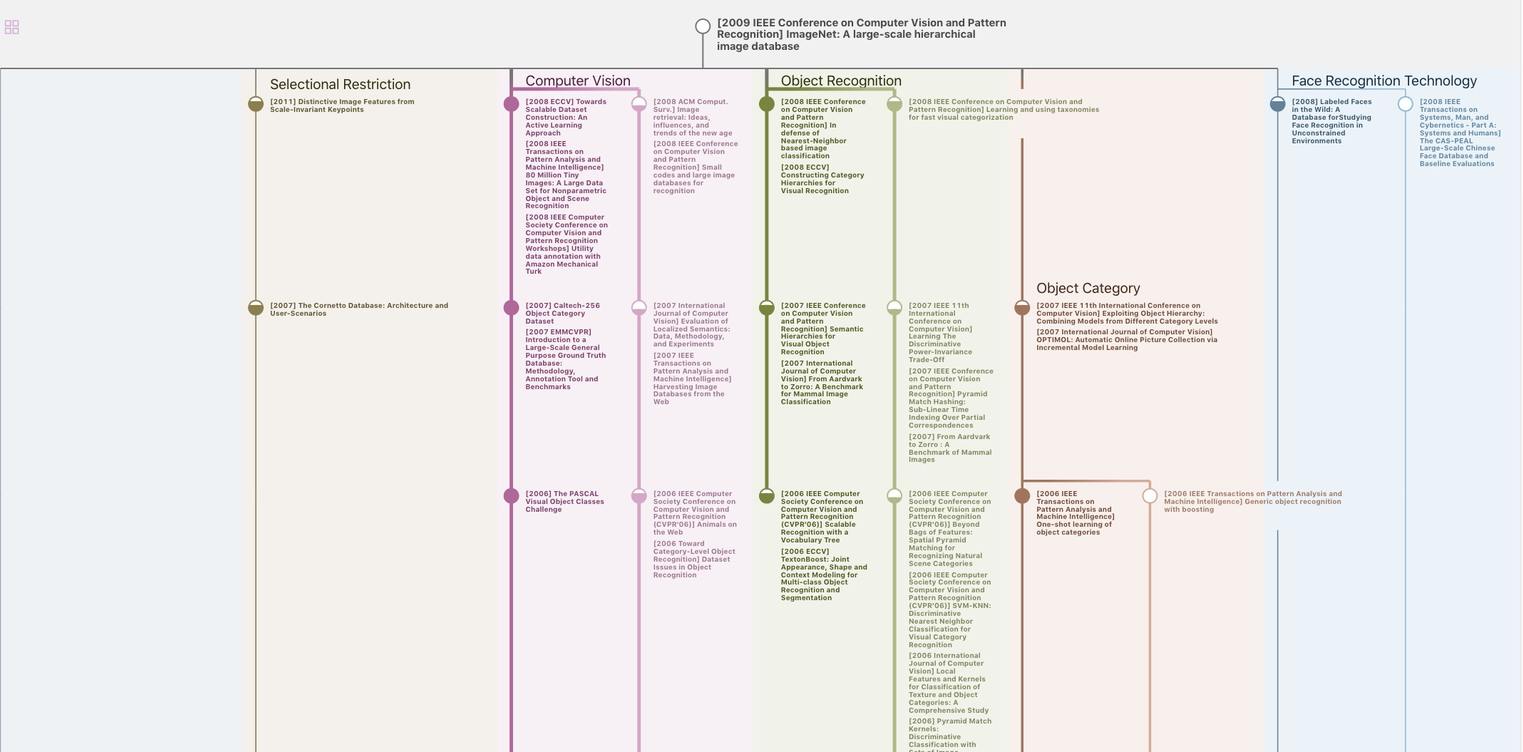
生成溯源树,研究论文发展脉络
Chat Paper
正在生成论文摘要