Attribute-based document image retrieval
International Journal on Document Analysis and Recognition (IJDAR)(2024)
摘要
This paper explores the use of attributes for document image querying and retrieval. Existing document image retrieval techniques present several drawbacks: textual searches are limited to text, query-by-example searches require a sample query document on hand, and layout-based searches rigidly assign documents to one of several preset classes. Attributes have yet to be fully exploited in document image analysis. We describe document images based on attributes and utilize those descriptions to form a new querying paradigm for document image retrieval that addresses the above limitations: attribute-based document image retrieval (ABDIR). We create attribute-based descriptions of the documents using an expandable set of individual, independent attribute classifiers built on convolutional neural network architectures. We combine the descriptions to form queries of variable complexity which retrieve a ranked list of document images. ABDIR allows users to search for documents based on memorable visual features of their contents in a flexible way, with queries like “Find documents that have a one-column layout, are table dominant, and are colorful”, or “Find historical documents that are illuminated and have see-through artifacts”. Experiments on the recent PubLayNet and HisIR19 datasets demonstrate the system’s ability to extract various document image attributes with high accuracy, with Darknet-53 performing best, and show very promising results for document image retrieval. ABDIR is scalable and versatile: it is easy to change, add, and remove attributes, and easy to adapt queries to new domains. It provides for document image retrieval capabilities that are not possible or are impractical with other paradigms.
更多查看译文
关键词
Document image retrieval,Document querying,Visual attributes,Convolutional neural networks,Document classification
AI 理解论文
溯源树
样例
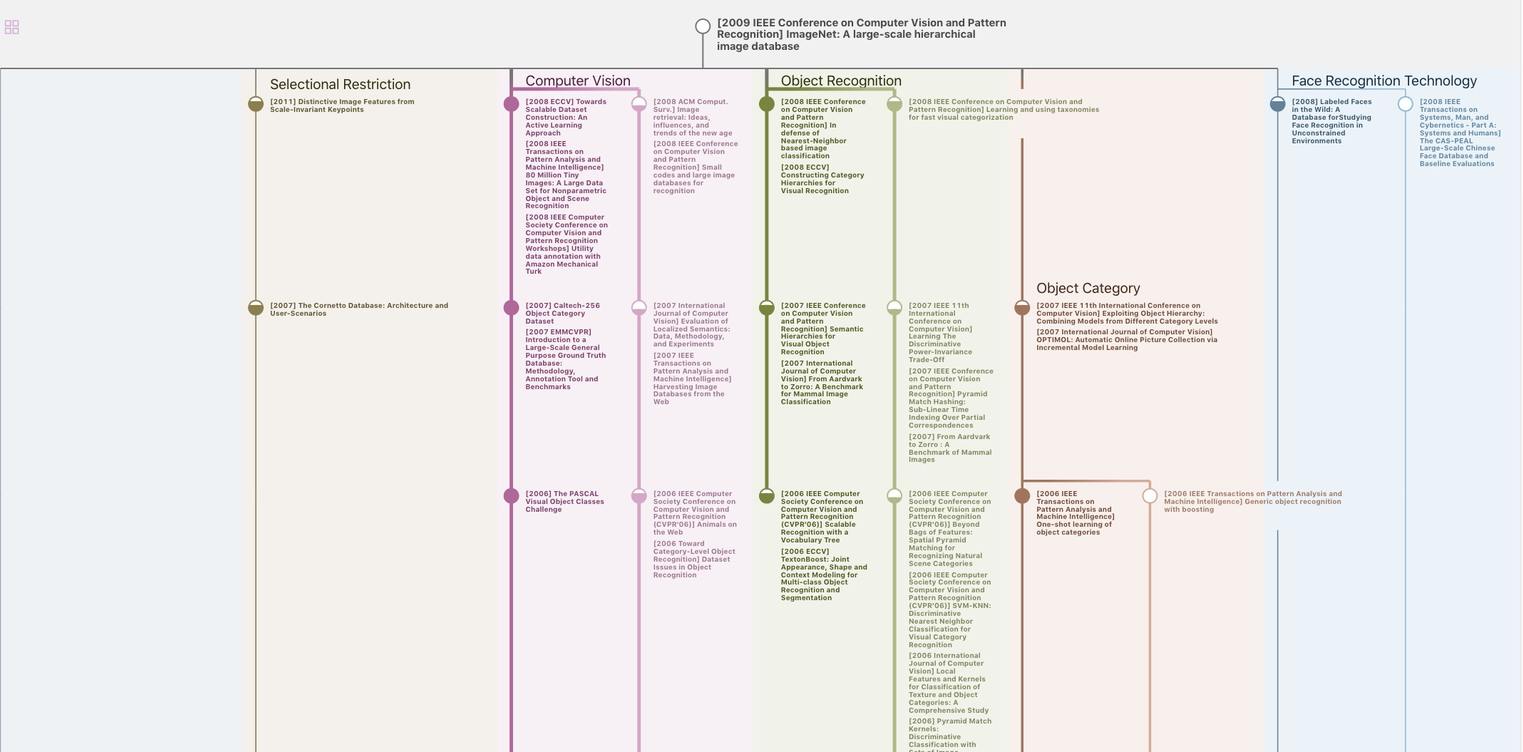
生成溯源树,研究论文发展脉络
Chat Paper
正在生成论文摘要