Converting hyperparameter gamma in distance-based loss functions to normal parameter for knowledge graph completion
APPLIED INTELLIGENCE(2023)
摘要
The parameter gamma, which is used in distance-based knowledge graph embedding to distinguish positive and negative samples, plays an important role in model performance. Usually, gamma is considered a hyperparameter and taken from a discrete set. However, as the boundary of positive and negative samples, gamma may have a continuous property and be complementary to other parameters. The high consistency between gamma and the learnable parameter with respect to their attributes means that choosing gamma as a hyperparameter is probably not a good choice. To better explore the characteristics of gamma, we propose a multi-iterated parameterized scheme to convert gamma from a hyperparameter to a normal parameter. Two concrete implementation methods, the macro parametrized method and the micro parameterized method, are provided to achieve this conversion. The conversion for gamma can reduce dependency on expert knowledge in the gamma setting and can allow successors to become involved in the recent framework quickly. Experimental results on TransE, RotatE and LineaRE show that the proposed scheme and methods can achieve approximate or better results when compared with those of the original method. A series of experimental analyses further explore the characteristics and illustrate the availability of the proposed parameterized scheme and methods.
更多查看译文
关键词
Knowledge graph embedding,Knowledge graph completion,Hyperparameter conversion,Parameter learning
AI 理解论文
溯源树
样例
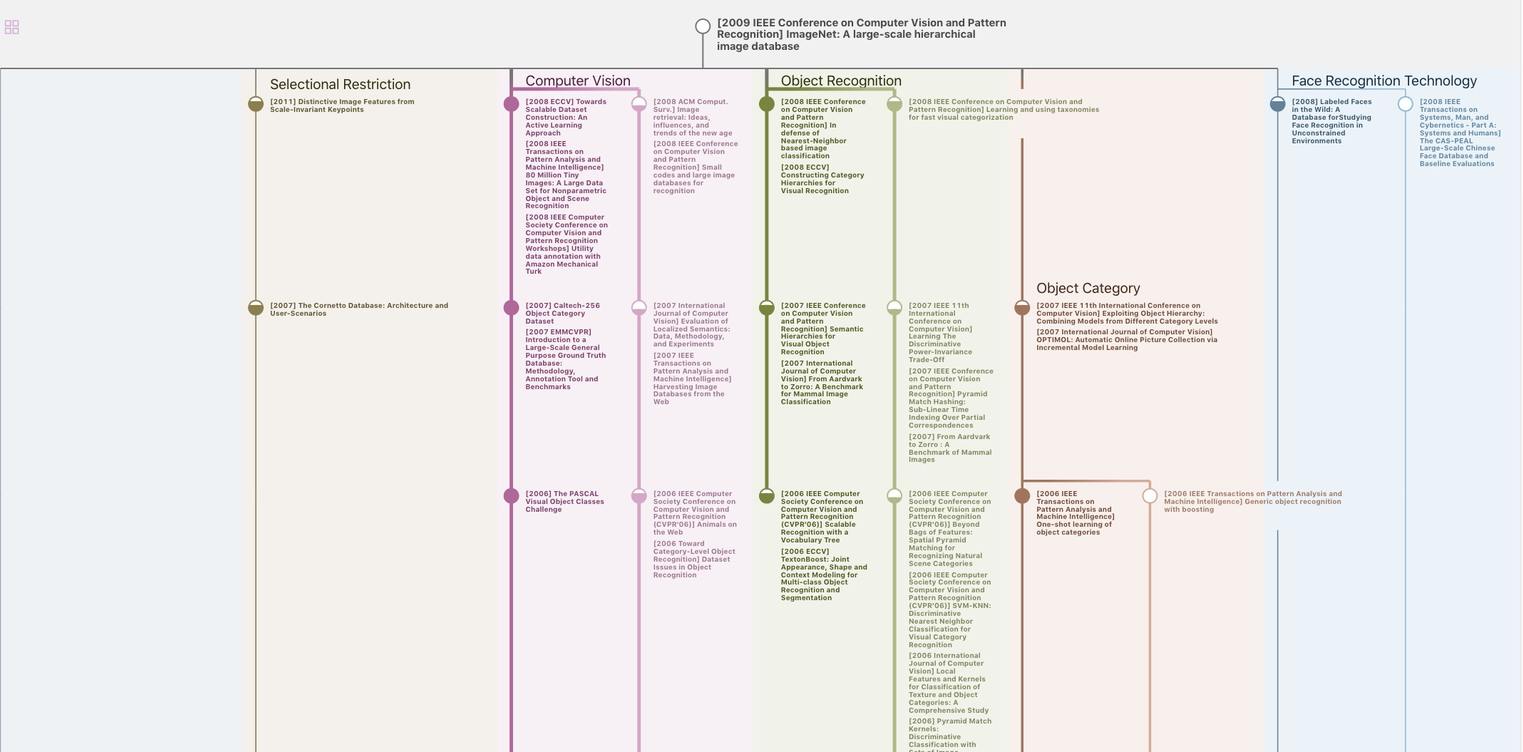
生成溯源树,研究论文发展脉络
Chat Paper
正在生成论文摘要