Quantifying the uncertainty of mobility flow predictions using Gaussian processes
TRANSPORTATION(2023)
摘要
The ability to understand and predict the flows of people in cities is crucial for the planning of transportation systems and other urban infrastructures. Deep-learning approaches are powerful since they can capture non-linear relations between geographic features and the resulting mobility flow from a given origin location to a destination location. However, existing methods are not able to quantify the uncertainty of the predictions, which limits their interpretability and thus their use for practical applications in urban infrastructure planning. To that end, we propose a Bayesian deep-learning approach that formulates deep neural networks as Gaussian processes and integrates automatic variable selection. Our method provides uncertainty estimates for the predicted origin-destination flows while also allowing to identify the most important geographic features that drive the mobility patterns. The developed machine learning approach is applied to large-scale taxi trip data from New York City.
更多查看译文
关键词
Mobility,Bayesian deep learning,Smart cities,Transportation system planning
AI 理解论文
溯源树
样例
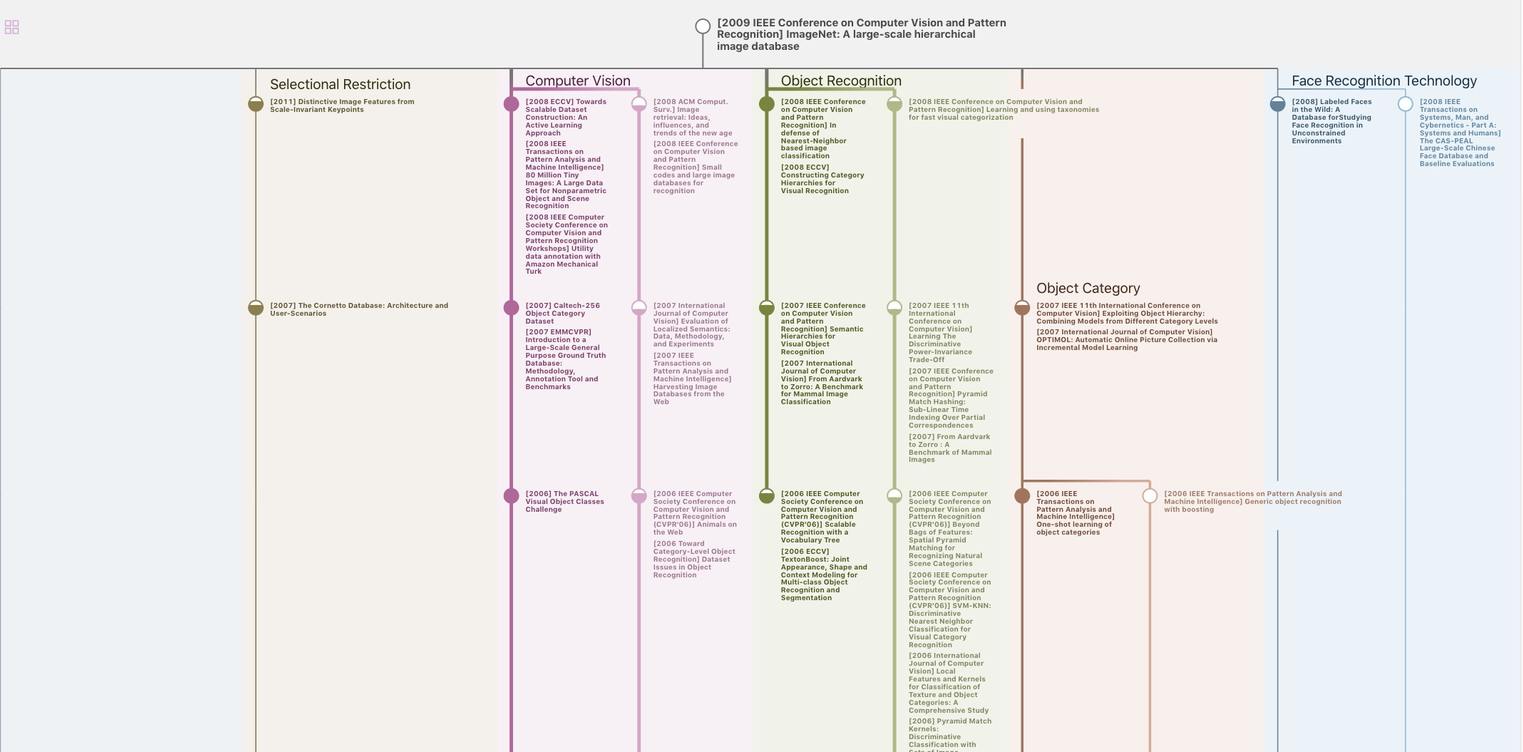
生成溯源树,研究论文发展脉络
Chat Paper
正在生成论文摘要