Adaptive remaining useful life prediction framework with stochastic failure threshold for experimental bearings with different lifetimes under contaminated condition
International Journal of System Assurance Engineering and Management(2023)
摘要
Deterioration modelling and remaining useful life (RUL) prediction of roller bearings is critical to ensure a safe, reliable, and efficient operation of rotating machinery. RUL prediction models in model-based approaches are often based on constant failure threshold and time-domain features for bearings’ failure prognosis. Due to nonlinearity of the acceleration signals, noises, and measurement errors, the time-domain features used as condition indicators are unable to track bearings’ degradation successfully and they are mostly utilized for fault diagnosis, especially in the fault classification field using machine learning algorithms. This paper proposes an adaptive RUL prediction framework with a stochastic failure threshold which comprises of two main phases of feature extraction and RUL prediction using laboratory-acquired accelerated life test data obtained from contaminated bearings. The first phase is to decompose the empirical input signals into different frequency bands using some time–frequency transformation functions and extract several condition indicators for the second phase. The second phase is based on a stochastic Wiener process while the key parameters of the model are updated iteratively using a Bayesian approach, and RUL at different degradation datapoints is computed numerically. The experimental results showed the good performance of the developed framework. Some factors affecting RUL prediction such as the length of bearing samples, and degradation mechanism are highlighted in the result. The results of this paper can be further used for an effective maintenance optimization, determining an optimal maintenance alarm threshold, improving the reliability and safety of rotating machinery, and reducing the downtime cost.
更多查看译文
关键词
Remaining useful life,Bearing,Prognosis,Stochastic modeling,Bayesian inference,Accelerated life tests,Rotating equipment
AI 理解论文
溯源树
样例
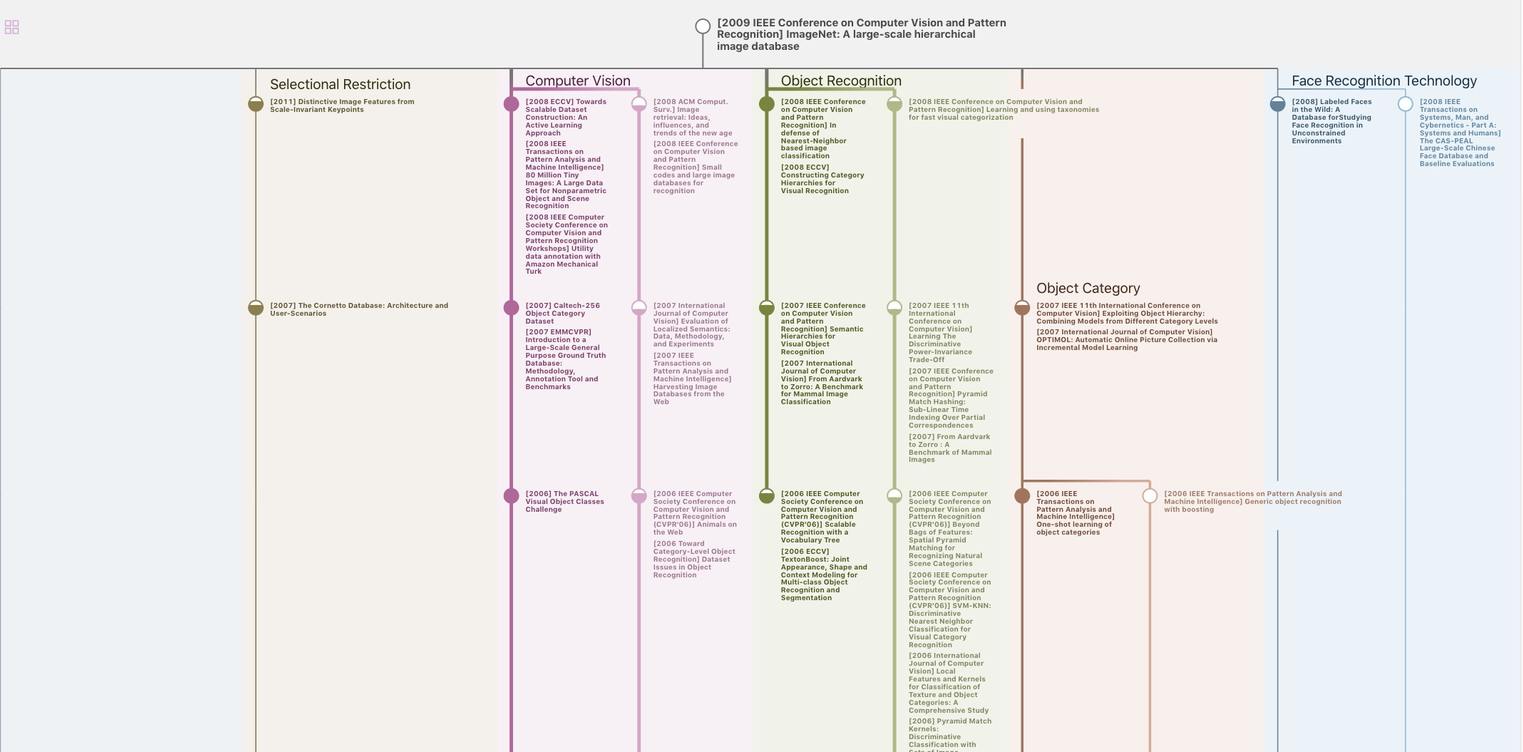
生成溯源树,研究论文发展脉络
Chat Paper
正在生成论文摘要