Explainable active learning in investigating structure–stability of SmFe 12-α-β X α Y β structures X, Y {Mo, Zn, Co, Cu, Ti, Al, Ga}
MRS Bulletin(2022)
Abstract
In this article, we propose a query-and-learn active learning approach combined with first-principles calculations to rapidly search for potentially stable crystal structure via elemental substitution, to clarify their stabilization mechanism, and integrate this approach to SmFe _12 -based compounds with ThMn _12 structure, which exhibits prominent magnetic properties. The proposed method aims to (1) accurately estimate formation energies with limited first-principles calculation data, (2) visually monitor the progress of the structure search process, (3) extract correlations between structures and formation energies, and (4) recommend the most beneficial candidates of SmFe _12 -substituted structures for the subsequent first-principles calculations. The structures of SmFe _12- -𝖷_𝖸_ before optimization are prepared by substituting 𝖷, 𝖸 elements—Mo, Zn, Co, Cu, Ti, Al, Ga—in the region of + <4 into iron sites of the original SmFe _12 structures. Using the optimized structures and formation energies obtained from the first-principles calculations after each active learning cycle, we construct an embedded two-dimensional space to rationally visualize the set of all the calculated and not-yet-calculated structures for monitoring the progress of the search. Our machine learning model with an embedding representation attained a prediction error for the formation energy of 1.25× 10^-2 (eV/atom) and required only one-sixth of the training data compared to other learning methods. Moreover, the time required to recall most potentially stable structures was nearly four times faster than the random search. The formation energy landscape visualized using the embedding representation revealed that the substitutions of Al and Ga have the highest potential to stabilize the SmFe _12 structure. In particular, SmFe _9 [Al/Ga] _2 Ti showed the highest stability among the investigated structures. Finally, by quantitatively measuring the change in the structures before and after optimization using OFM descriptors, the correlations between the coordination number of substitution sites and the resulting formation energy are revealed. The negative-formation-energy-family SmFe _12- - [Al/Ga] _𝖸_ structures show a common trend of increasing coordination number at substituted sites, whereas structures with positive formation energy show a corresponding decreasing trend. Impact statement Seeking the next generation of high-performance magnets is a crucial demand for replacing the widely accepted Nd-Fe-B magnets developed in the middle 80s. The iron-rich compounds with the original tetragonal ThMn 12 structure appear as the most potential candidates except for the hard synthesizing it in nature due to its high energy of formation. Stabilization for this material system is expected by substituting new elements, but the vast number of possible structures makes the exploration difficult even for theoretical calculations. This article proposes an integration of first-principles calculations and explainable active learning to efficiently explore the crystal structure space of this material system. In particular, the explored crystal structure space can be rationally visualized, on which the relationship between substitution elements, substitution sites, and crystal structure stabilization can be intuitively interpreted.
MoreTranslated text
Key words
explainable active learning,structures,structure–stability
AI Read Science
Must-Reading Tree
Example
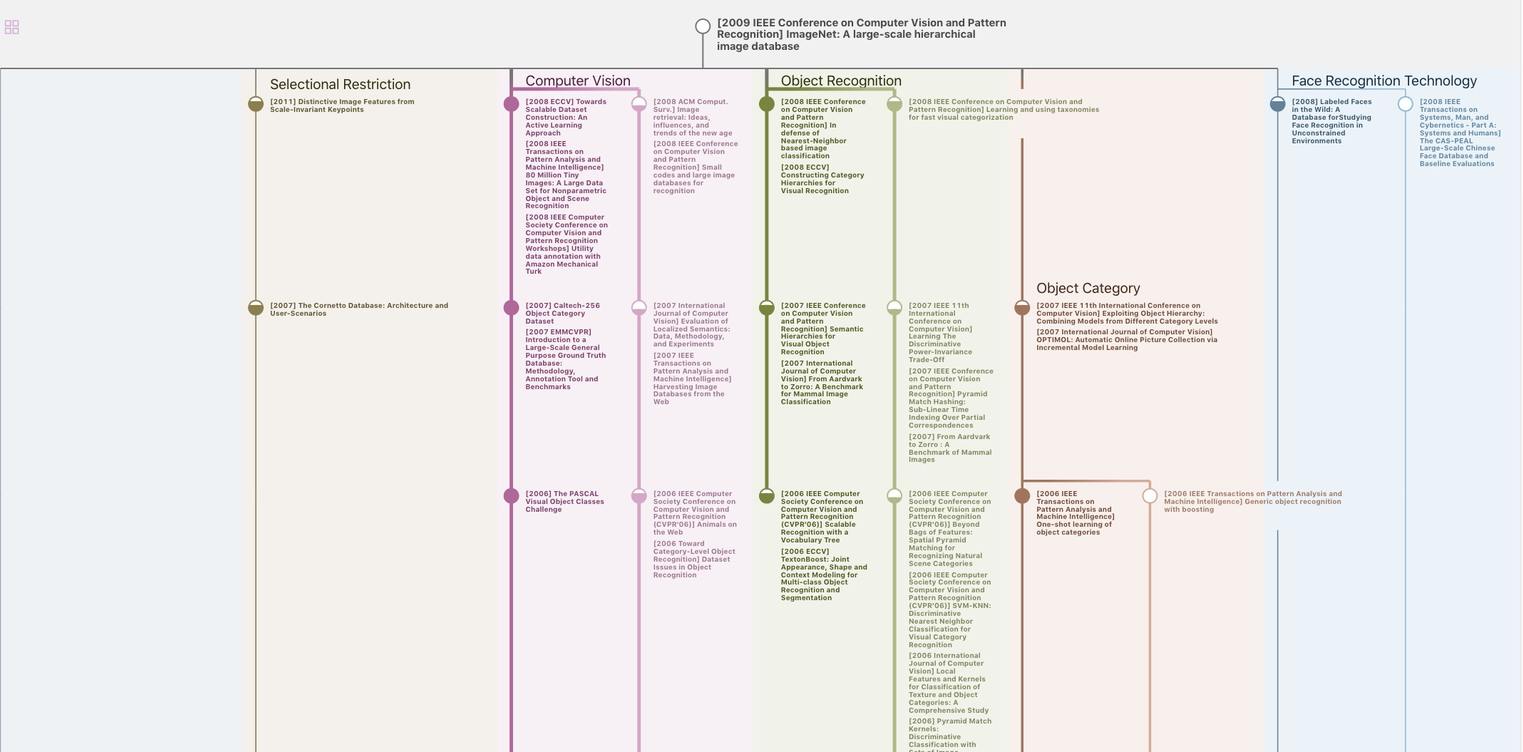
Generate MRT to find the research sequence of this paper
Chat Paper
Summary is being generated by the instructions you defined