A comparative study of empirical and ensemble machine learning algorithms in predicting air over-pressure in open-pit coal mine
Acta Geophysica(2020)
摘要
This study aims to take into account the feasibility of three ensemble machine learning algorithms for predicting blast-induced air over-pressure (AOp) in open-pit mine, including gradient boosting machine (GBM), random forest (RF), and Cubist. An empirical technique was also applied to predict AOp and compared with those of the ensemble models. To employ this study, 146 events of blast were investigated with 80% of the total database (approximately 118 blasting events) being used for developing the models, whereas the rest (20% ~ 28 blasts) were used to validate the models’ accuracy. RMSE, MAE, and R 2 were used as performance indices for evaluating the reliability of the models. The findings revealed that the ensemble models yielded more precise accuracy than those of the empirical model. Of the ensemble models, the Cubist model provided better performance than those of RF and GBM models with RMSE, MAE, and R 2 of 2.483, 0.976, and 0.956, respectively, whereas the RF and GBM models provided poorer accuracy with an RMSE of 2.579, 2.721; R 2 of 0.953, 0.950, and MAE of 1.103, 1.498, respectively. In contrast, the empirical model was interpreted as the poorest model with an RMSE of 4.448, R 2 of 0.872, and MAE of 3.719. In addition, other findings indicated that explosive charge capacity, spacing, stemming, monitoring distance, and air humidity were the most important inputs for the AOp predictive models using artificial intelligence.
更多查看译文
关键词
Air over-pressure,Open-pit mine,Ensemble algorithm,Random forest,Gradient boosting machine,Cubist
AI 理解论文
溯源树
样例
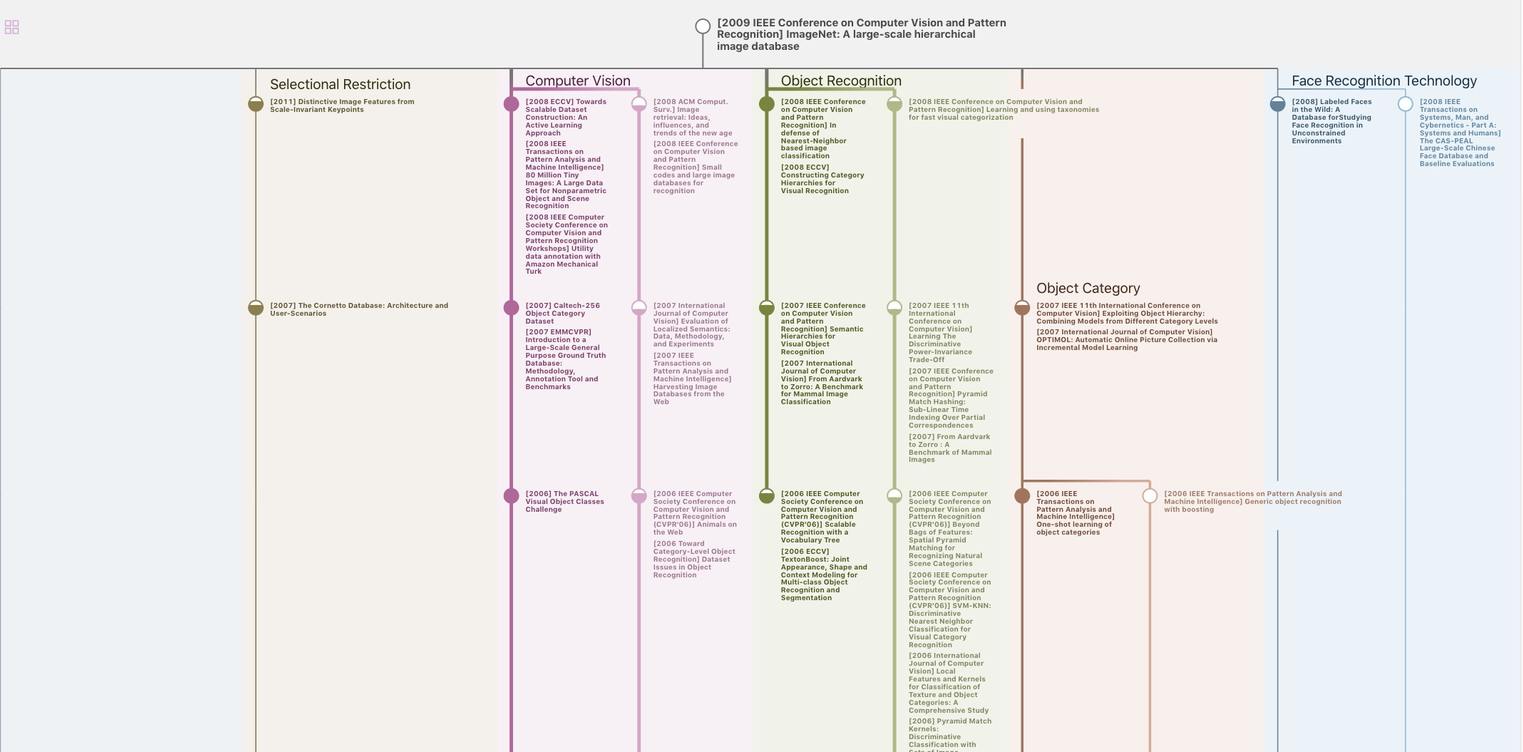
生成溯源树,研究论文发展脉络
Chat Paper
正在生成论文摘要