An Active-Set Proximal-Newton Algorithm for ℓ _1 Regularized Optimization Problems with Box Constraints
Journal of Scientific Computing(2020)
摘要
In this paper, we propose an active-set proximal-Newton algorithm for solving ℓ _1 regularized convex/nonconvex optimization problems subject to box constraints. Our algorithm first relies on the KKT error to estimate the active and free variables, and then smoothly combines the proximal gradient iteration and the Newton iteration to efficiently pursue the convergence of the active and free variables, respectively. We show the global convergence without the convexity of the objective function. For some structured convex problems, we further design a safe screening procedure that is able to identify/remove active variables, and can be integrated into the basic active-set proximal-Newton algorithm to accelerate the convergence. The algorithm is evaluated on various synthetic and real data, and the efficiency is demonstrated particularly on ℓ _1 regularized convex/nonconvex quadratic programs and logistic regression problems.
更多查看译文
关键词
Active set,Proximity operator,Newton method,ℓ _1 regularization,Box constraints
AI 理解论文
溯源树
样例
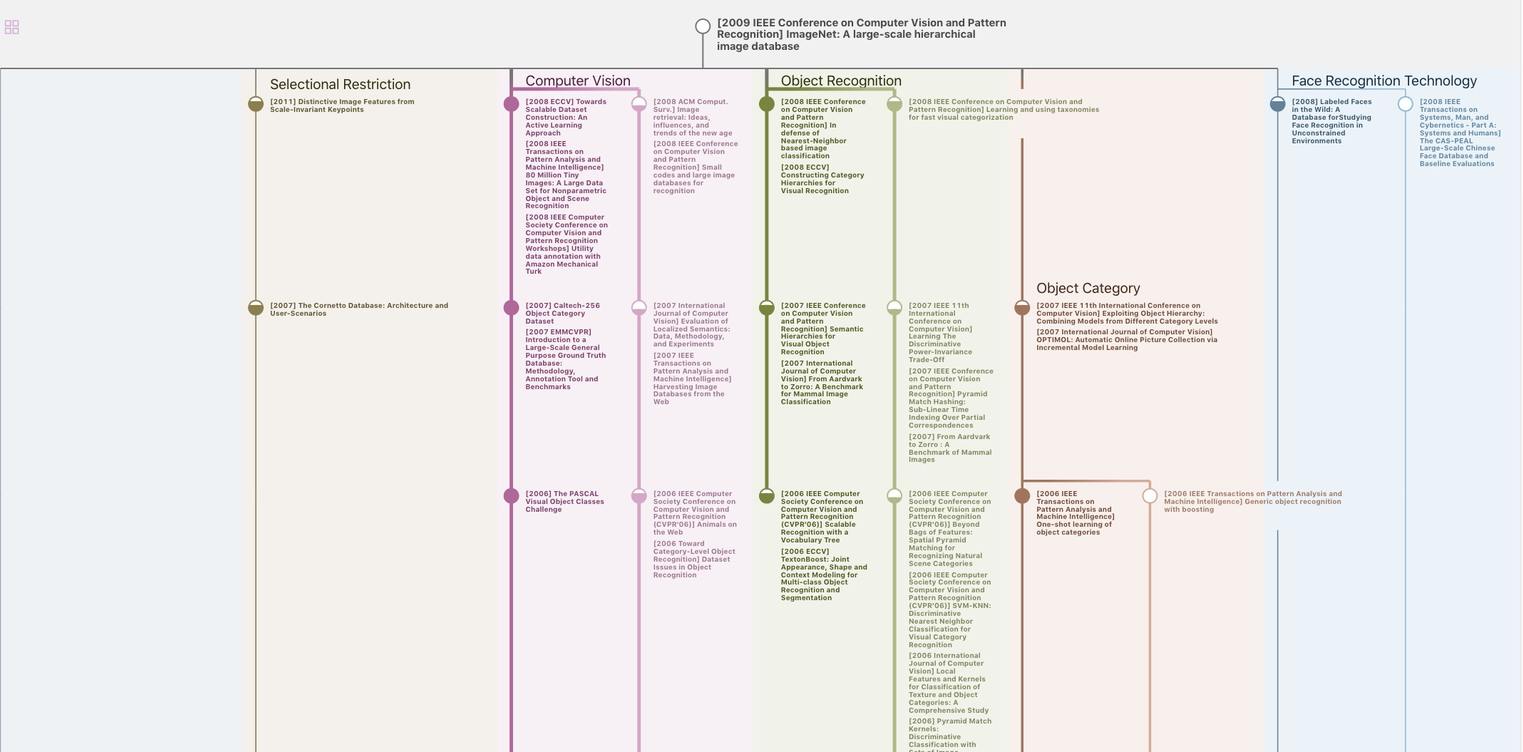
生成溯源树,研究论文发展脉络
Chat Paper
正在生成论文摘要