A pseudo-parabolic diffusion model to enhance deep neural texture features
Multimedia Tools and Applications(2024)
摘要
In this work, we propose a methodology for texture recognition. The combination of deep learning with texture encoding techniques has demonstrated to be a powerful strategy to solve this problem. However, one of such encoding classically used in texture images, which are PDE operators, has not been explored in deep learning frameworks. Based on that, here we introduce a pseudo-parabolic diffusion operator to the pipeline of a convolutional neural network (CNN). The method is divided into 4 major stages: 1) application of the pseudo-parabolic operator to the image; 2) use of the resulting image as input to a pre-trained CNN; 3) extraction of local features from the last convolutional layer and pooling by Fisher vectors; 4) classification of the pooled features. Our approach is compared in a texture classification task and outperforms, in terms of classification accuracy, several state-of-the-art solutions. For example, in the challenging benchmark datasets KTH2b and FMD, the proposed method achieves classification accuracy of 79% and 81.8%, respectively. From the numerical viewpoint, we also highlight the key approximation and algorithmic aspects of our computational pseudo-parabolic diffusion modeling into the pipeline of a CNN to enhance deep neural texture features. In particular, the advantage over the plain CNN architecture is substantial. Such interesting performance can be justified by the capacity of the pseudo-parabolic operator to remove spurious noise while preserving important discontinuity information on the texture. The results also suggest the potential of our approach in real-world application, as attested on a practical application to the identification of plant species. In this specific task, our method achieves an accuracy of 94% and outperforms the state-of-the-art results. This is especially the case when we do not have access to a large amount of data for training and when the computational resources are limited, as our method do not involve any fine tuning.
更多查看译文
关键词
Texture recognition,Partial Differential Equation (PDE),Convolutional neural networks,Image descriptors,PDE image/texture process
AI 理解论文
溯源树
样例
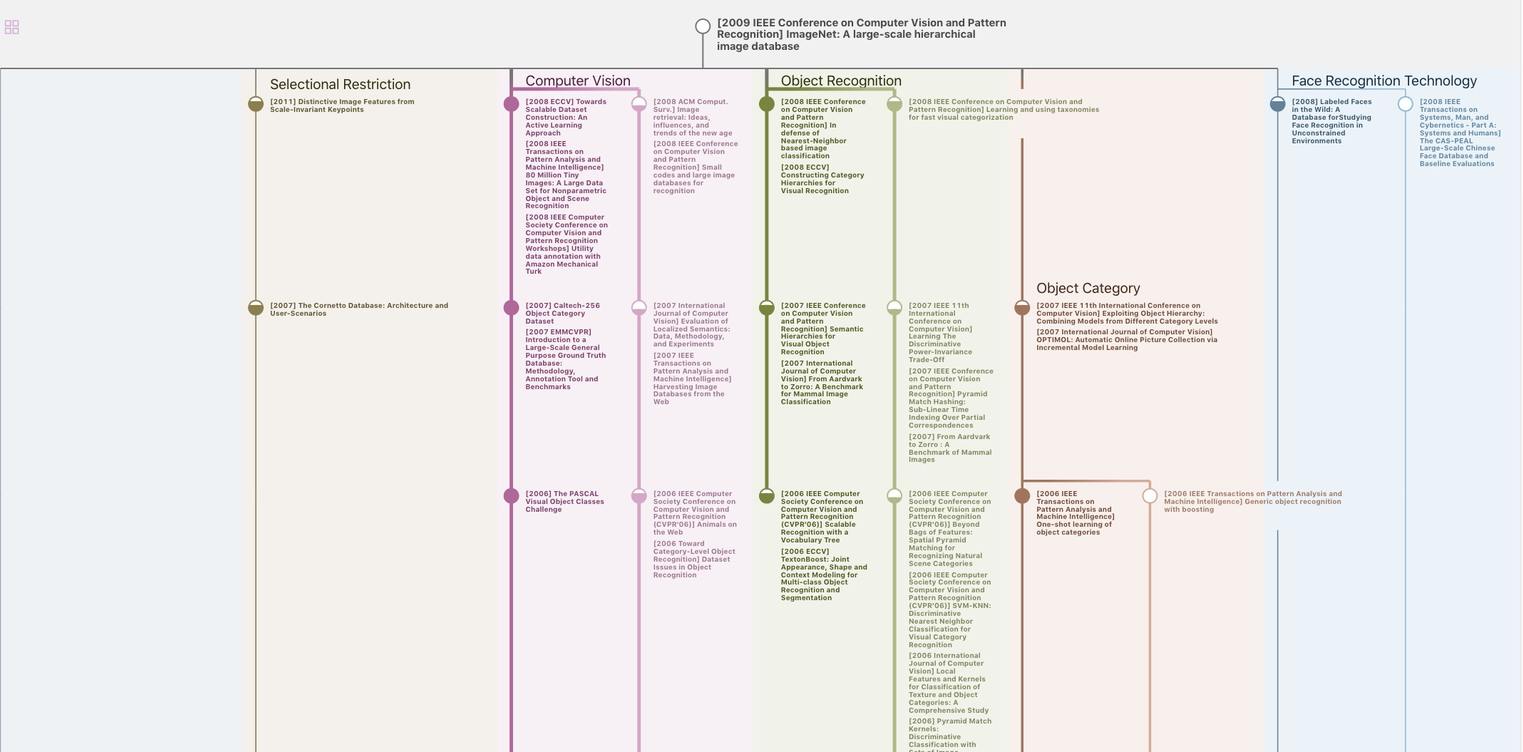
生成溯源树,研究论文发展脉络
Chat Paper
正在生成论文摘要