State estimation of hydraulic quadruped robots using invariant-EKF and kinematics with neural networks
NEURAL COMPUTING & APPLICATIONS(2024)
Abstract
The research on state estimation for quadruped robots is critical. Its result passed to motion controller makes the robot navigate autonomously and adjust the gait to a more stable motion. The current research depends on a multi-sensor fusion of cameras, lidars or other proprioceptive sensors, such as Inertial Measurement Unit (IMU) and encoders. The high-frequency data are generally derived from body sensors, which is to be fused with data from external sensors directly, or preprocessed with EKF first. Due to its unguaranteed convergence and robustness of tracking state mutations, EKF is insufficient. Therefore, we study state estimation for hydraulic quadruped robot based on the fusion of IMU measurement and leg odometry in this paper, and Invariant Extended Kalman Filter (IEKF) is successfully applied to quadruped robots by using this method. Besides, neural networks are utilized to train the weight functions of foot force and the state of leg odometry, and our trained functions improve the accuracy of observation compared with common weight average methods. Finally, our experiments of accuracy show that the root mean square error of our method is significantly reduced and the absolute trajectory error is reduced by 30% compared to traditional IEKF. The algorithm achieves the drift per distance travelled below 4 cm/m. Moreover, it has good robustness.
MoreTranslated text
Key words
Invariant-EKF,Neural networks,Quadruped robot,Multi-sensor,IMU measurement
AI Read Science
Must-Reading Tree
Example
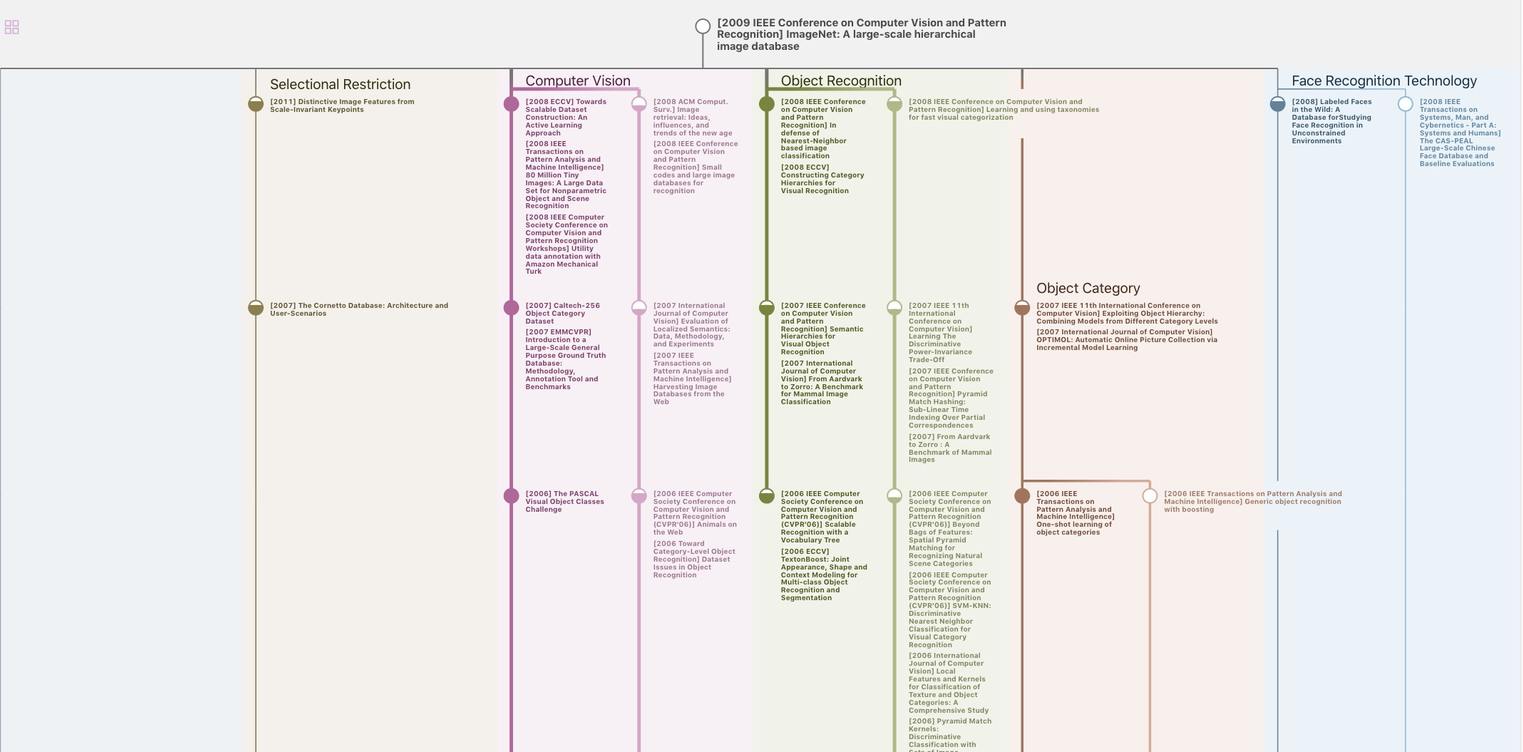
Generate MRT to find the research sequence of this paper
Chat Paper
Summary is being generated by the instructions you defined