Flow-dependent wind extraction in strong-constraint 4D-Var
QUARTERLY JOURNAL OF THE ROYAL METEOROLOGICAL SOCIETY(2023)
摘要
In the process of data assimilation for numerical weather prediction, biases in the model and observations can induce spurious analysis increments, which degrade the quality of the analyses. For this reason, the feedback of atmospheric composition (e.g., ozone and aerosols) observations on winds through dynamical adjustment is typically disabled in operational 4D-Var assimilation. This study investigates whether an increasing number of tracer observations could be exploited to constrain winds better in 4D-Var. For an idealized case study using analytical strong-constraint 4D-Var with the 1D advection model of Allen et al. and accurate background tracer field, it is shown that coupled wind-tracer assimilation always improves the tracer analysis. It also improves the wind analysis, but only if the magnitude of the error associated with unrepresented or misrepresented tracer physical forcings is smaller than the magnitude of the difference between the nature-run advection and the background-tracer advection. In other words, the model needs to be a good approximation of the truth for successful wind extraction. Based on this criterion, a method for flow-dependent 4D-Var wind extraction is developed, which selectively activates the coupling between winds and tracers locally and temporally by altering the tangent-linear tracer equation and the adjoint wind equation. The new method is implemented in the intermediate-complexity incremental 4D-Var model Moist Atmosphere Dynamics Data Assimilation Model (MADDAM) of Zaplotnik et al. Numerical experiments with MADDAM show that the new approach diminishes the occurrence of spurious wind-analysis increments and can improve the accuracy of wind analyses.
更多查看译文
关键词
4D-Var,data assimilation,ensemble,flow-dependent,model error,wind extraction,wind tracing
AI 理解论文
溯源树
样例
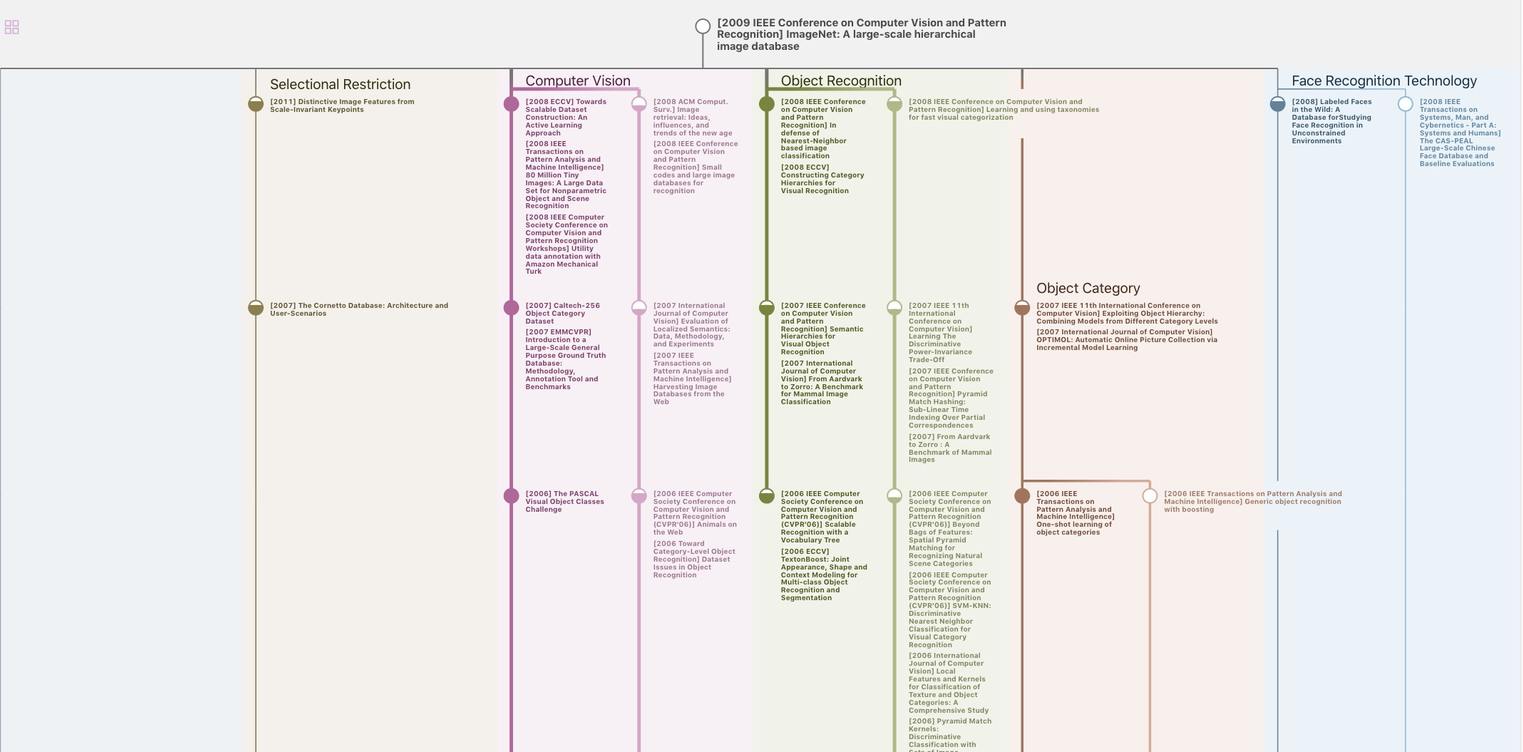
生成溯源树,研究论文发展脉络
Chat Paper
正在生成论文摘要