Digital twin-assisted enhanced meta-transfer learning for rolling bearing fault diagnosis
MECHANICAL SYSTEMS AND SIGNAL PROCESSING(2023)
摘要
Fault diagnosis of bearing under variable working conditions is widely required in practice, and the combination of working conditions and fault fluctuations increases the complexity of addressing its related problems. By developing a virtual simulation model, a digital twin (DT) can obtain the same or even more information than the physical object at a lower cost. Furthermore, it has great potential in the application of bearing fault diagnosis. In this paper, the DT model of the bearing test rig is robustly established, and the fault diagnosis bearing between the simulation and physical object is realized using the proposed enhanced meta -transfer learning (EMTL). First, the DT model is established through parameter identification and modal testing, and the modeling accuracy of DT model reaching 95.685%. The bearing simulation and experimental data are then collected under the same conditions using the DT model and bearing test rig, and the simulation data with little deviation from the experimental data is obtained. Finally, an attention mechanism and domain adaptation are introduced into the EMTL, with the average accuracy of fault diagnosis of bearing reaching 95.18% with few-label target domain data. The proposed strategy is both theoretically significant and practically useful. The experiment results demonstrate that our method outperforms a series of state-of-the-art methods on the bearing fault diagnosis across various limited data conditions. The proposed strategy effectively solves the few-shot problem, which is both theoretically significant and practically useful.
更多查看译文
关键词
Digital twin, Meta-transfer learning, Few-shot learning, Finite element model updating, Bearing fault diagnosis
AI 理解论文
溯源树
样例
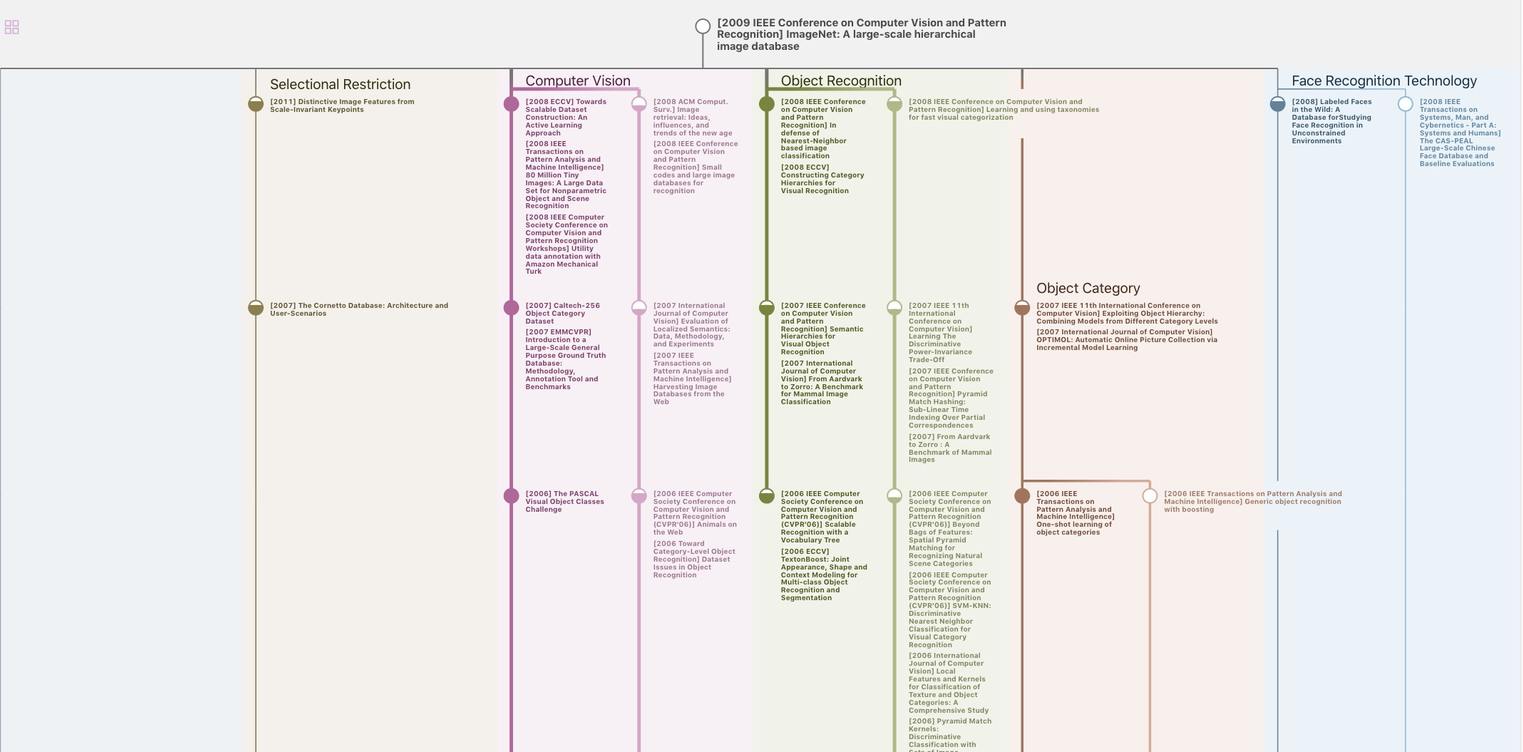
生成溯源树,研究论文发展脉络
Chat Paper
正在生成论文摘要