NRI-FGSM: An Efficient Transferable Adversarial Attack Method for Speaker Recognition System
INTERSPEECH 2022(2022)
摘要
Deep neural network (DNN), though widely applied in Speaker Recognition Systems (SRS), is vulnerable to adversarial attacks which are hard to detect by humans. The black-box model vulnerability against adversarial attacks is crucial for the robustness of SRS, especially for latest models such as x-vector and ECAPA. The state-of-the-art transferable adversarial attack methods start with generating the adversarial audio from white-box SRS, then utilize this audio to attack the black-box SRS. However, these methods often have a lower success rate in SRS than in the image processing domain. To improve the attack performance on SRS, we propose an efficient Nesterov accelerate and RMSProp optimization based Iterative-Fast Gradient Sign Method (NRI-FGSM), which integrates the Nesterov Accelerated Gradient method and the Root Mean Squared Propagation optimization method with adaptive step size. Through extensive experiments on both close-set speaker recognition (CSR) and open-set speaker recognition (OSR) tasks, our method achieves higher attack success rates of 97.8% for CSR and 61.9% for OSR tasks than others, and meanwhile maintains a lower perturbation rate with signal-to-noise ratio (SNR) and perceptual evaluation of speech quality (PESQ) metrics. It is worth mentioning that our work is the first to attack the ECAPA SRS model successfully.
更多查看译文
关键词
speaker recognition, transferable attack, nesterov accelerated gradient, root mean squared propagation
AI 理解论文
溯源树
样例
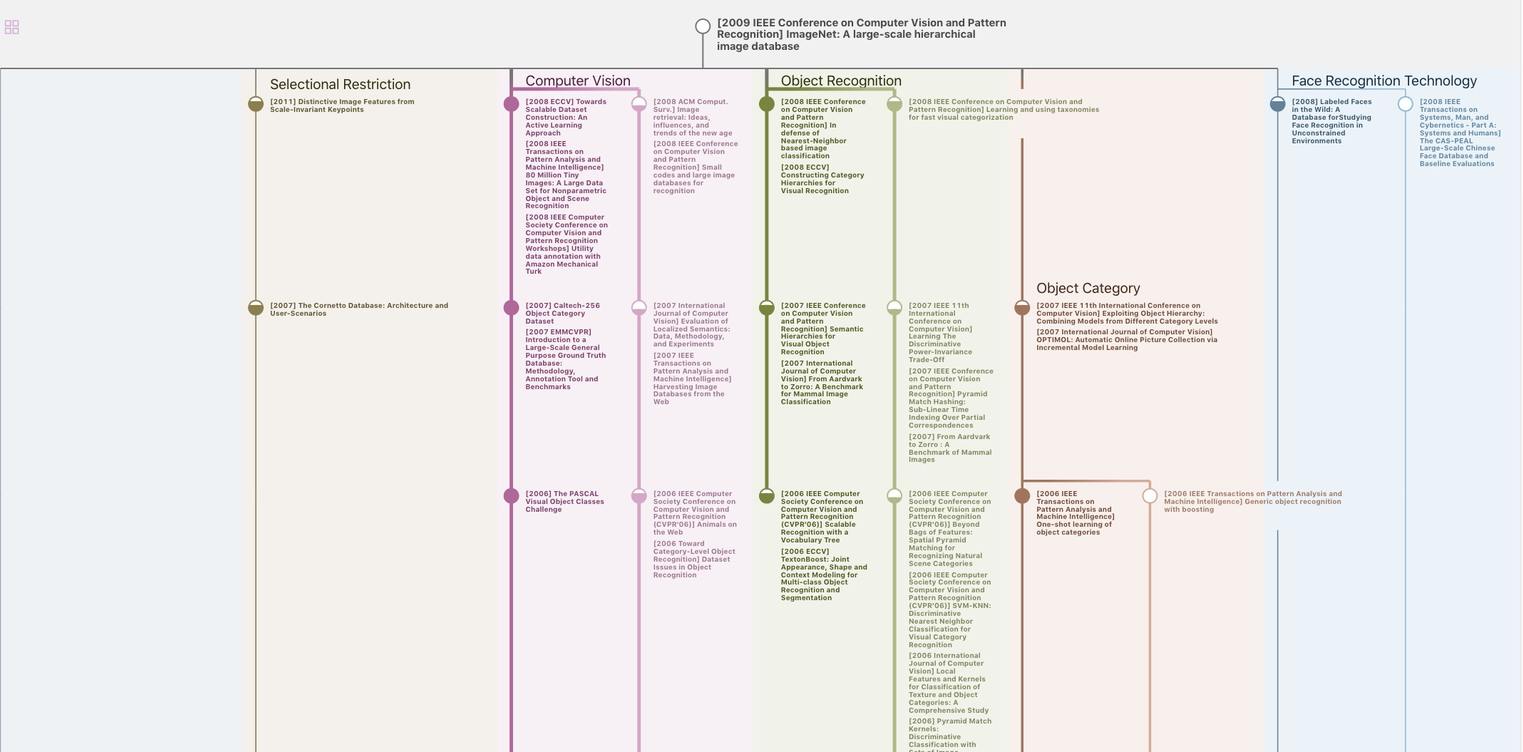
生成溯源树,研究论文发展脉络
Chat Paper
正在生成论文摘要