Hierarchical-Based Binary Moth Flame Optimization for Feature Extraction in Biomedical Application
MACHINE LEARNING, IMAGE PROCESSING, NETWORK SECURITY AND DATA SCIENCES, MIND 2022, PT I(2022)
Abstract
Feature extraction is a key challenging task to find the optimal features by alleviating irrelevant features to improve the classification accuracy. The brute force methods yield complete feature space and employ exhaustive search that makes the feature selection a non-deterministic polynomial problem. The meta-heuristic algorithm offers a better optimal solution through random search rather than a complete search. However, the MFO falls with local optima and poor convergence. In this paper, a novel methodology based on the Hierarchical Binary Moth Flame Optimization (HBMFO) with a K-Nearest Neighbor (KNN) classifier is proposed for feature extraction. The motive of this work is to reduce high dimensionality for large datasets through feature extraction. Here, the adaptive hierarchical method has been proposed to update the moth position towards the optimal solution pertaining to searching the space. The simulation results are accomplished on UCI Repository datasets to validate the superiority of the suggested optimization algorithm over existing techniques and feature selection can be carried out with respect to improving stability and accuracy.
MoreTranslated text
Key words
Feature extraction,Moth Flame Optimization,Classification
AI Read Science
Must-Reading Tree
Example
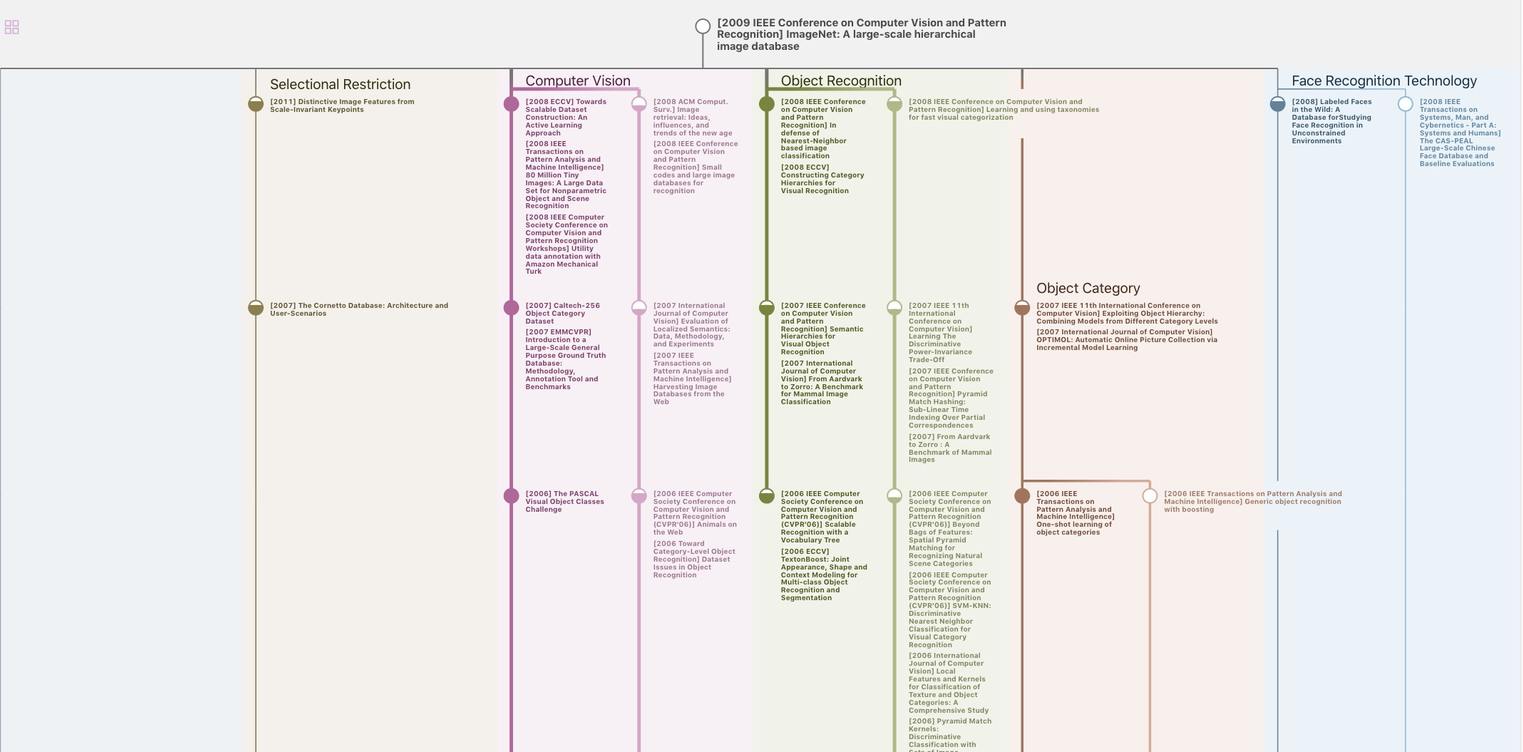
Generate MRT to find the research sequence of this paper
Chat Paper
Summary is being generated by the instructions you defined