A study on fault diagnosis of marine engine using a neural network with dimension-reduced vibration signals
JOURNAL OF THE ACOUSTICAL SOCIETY OF KOREA(2022)
摘要
This study experimentally investigates the effect of dimensionality reduction of vibration signal on fault diagnosis of a marine engine. By using the principal component analysis, a vibration signal having the dimension of 513 is converted into a low-dimensional signal having the dimension of 1 to 15, and the variation in fault diagnosis accuracy according to the dimensionality change is observed. The vibration signal measured from a full-scale marine generator diesel engine is used, and the contribution of the dimension-reduced signal is quantitatively evaluated using two kinds of variable importance analysis algorithms which are the integrated gradients and the feature permutation methods. As a result of experimental data analysis, the accuracy of the fault diagnosis is shown to improve as the number of dimensions used increases, and when the dimension approaches 10, near-perfect fault classification accuracy is achieved. This shows that the dimension of the vibration signal can be considerably reduced without degrading fault diagnosis accuracy. In the variable importance analysis, the dimension-reduced principal components show higher contribution than the conventional statistical features, which supports the effectiveness of the dimension-reduced signals on fault diagnosis.
更多查看译文
关键词
Engine condition monitoring, Vibration analysis, Dimension reduction, Neural network, Principal component analysis, Variable importance analysis
AI 理解论文
溯源树
样例
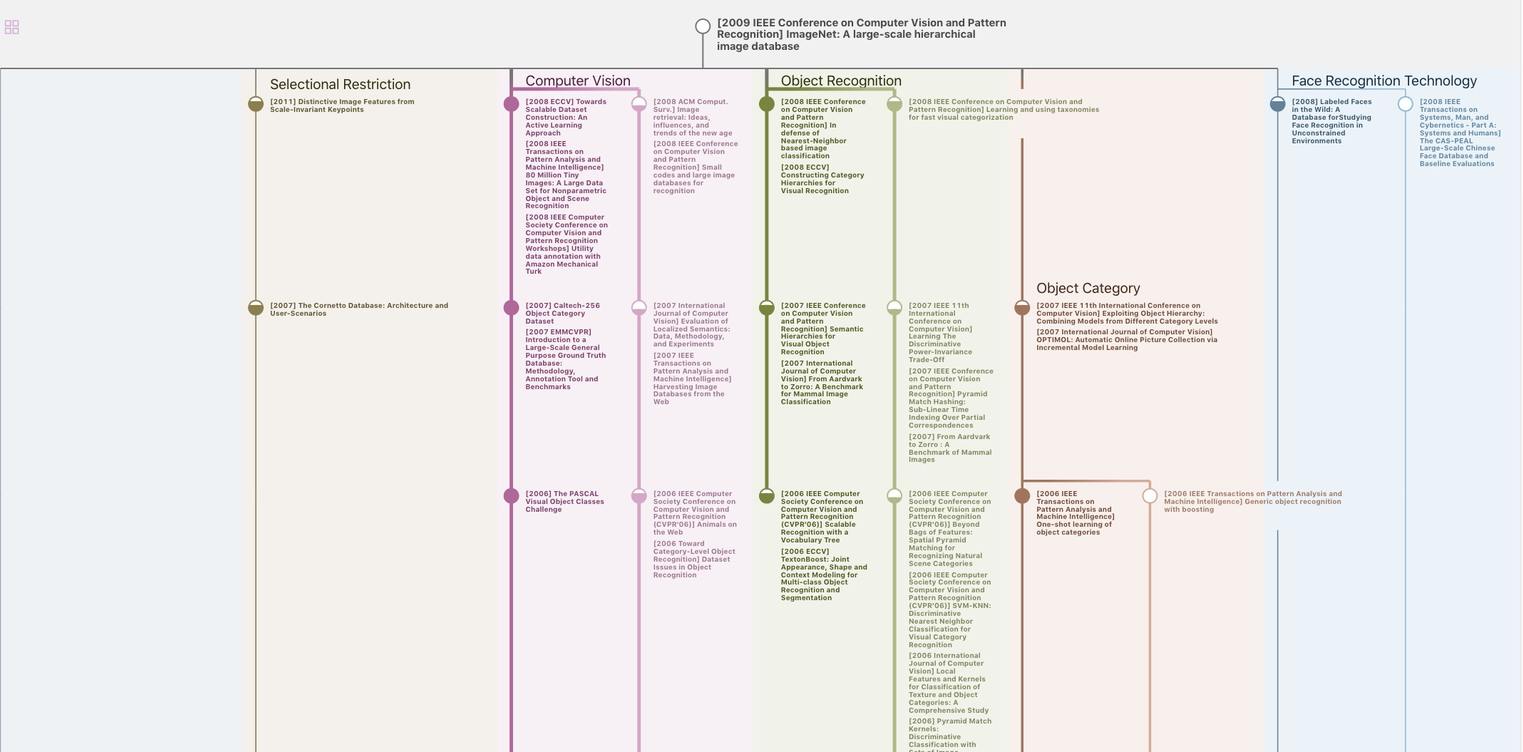
生成溯源树,研究论文发展脉络
Chat Paper
正在生成论文摘要