DeepAUP: A Deep Neural Network Framework for Abnormal Underground Heat Transport Pipelines
IEEE TRANSACTIONS ON AUTOMATION SCIENCE AND ENGINEERING(2023)
摘要
Pipeline inspection methods are continuously being developed, given that the safe maintenance and operation of heat transport pipelines is one of the most important issues in Korea. However, owing to the limitations of traditional inspection methods (e.g., excavation work), new solutions for underground heat-transport pipelines are required. Therefore, we propose DeepAUP, which is a deep neural network framework for detecting abnormalities in underground heat-transport pipelines. With datasets collected from actual environments using two sensors, DeepAUP effectively identified abnormal underground heat-transport pipelines, with more than 99% accuracy. Based on the experimental findings, several practical implications, as well as notable limitations, are examined. Note to Practitioners-This study was motivated by the several issues related to checking and maintaining underground heat-transport pipelines. Although there are several cornerstones for addressing this issue, they involve notable limitations, including economic and environmental concerns. Most existing studies indicate that expensive additional facilities, such as hoop strain sensors, are required to explore underground pipeline abnormalities. Thus, this study suggests a unique approach using deep learning called DeepAUP, which is expected to significantly reduce the effort required from practitioners. In future studies, we plan to employ a more efficient framework and a real-time detection system in the urban areas of South Korea.
更多查看译文
关键词
Pipeline,signal data,abnormal,MFCC,DeepAUP
AI 理解论文
溯源树
样例
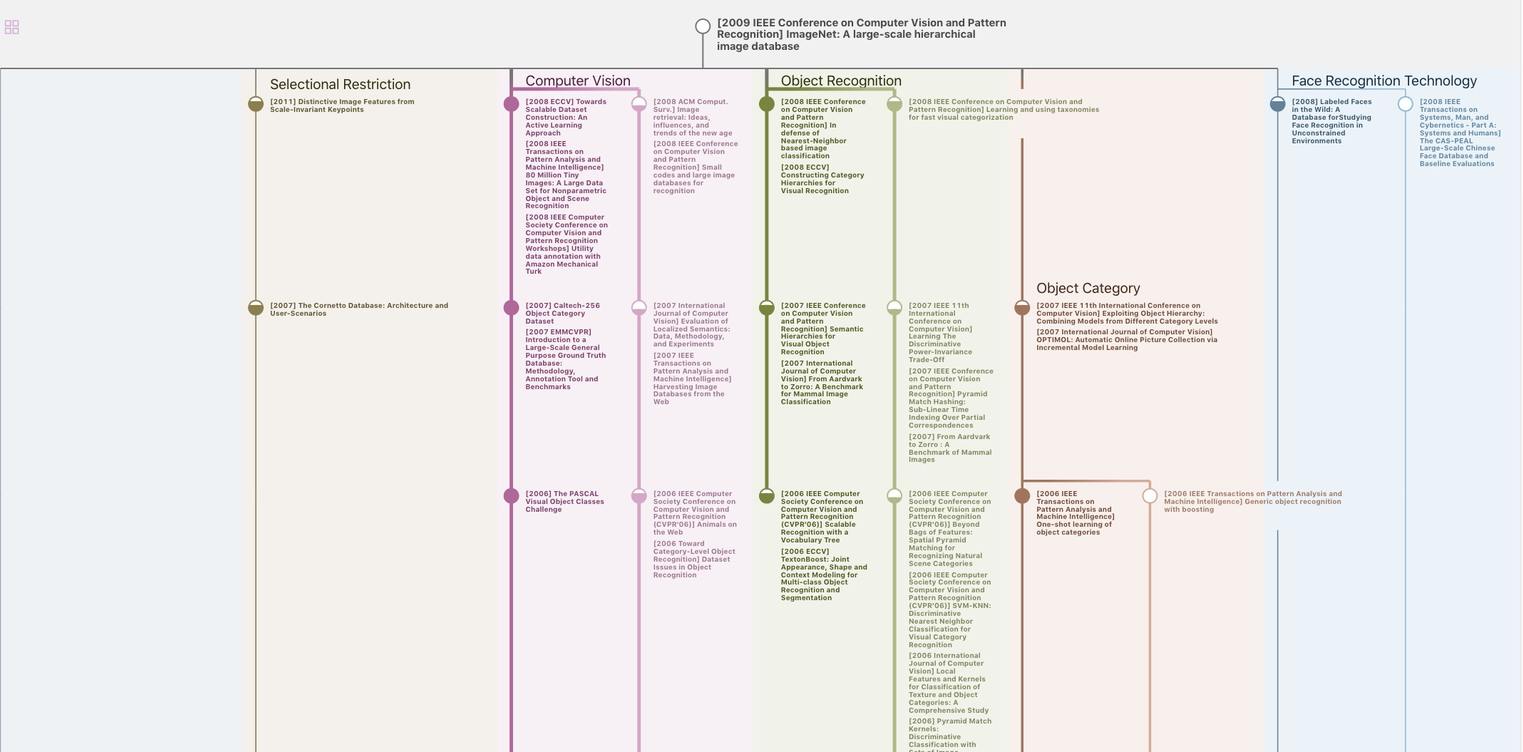
生成溯源树,研究论文发展脉络
Chat Paper
正在生成论文摘要