Defect detection and classification of offshore wind turbine rotor blades
NONDESTRUCTIVE TESTING AND EVALUATION(2023)
摘要
With increasing demand for electricity, wind turbines have gained significant attention from the public. Offshore wind power generation has emerged as a popular choice due to its potential to reduce power transmission losses and minimal impact on humans and organisms. However, it also presents challenges in detecting defects in Wind Turbine Rotor Blades (WTRB) and repairing them. To address this issue, this paper proposes an improved wavelet-based S-U-Net network to denoise WTRB images followed by a weakly supervised CNN method for removing background parts that could affect defective feature extraction. Defective features are then extracted using VGG16 network on a deep learning server platform, while an enhanced Particle Swarm Optimization (PSO) algorithm combined with K-means is used to classify defect features of WTRBs. Experimental results demonstrate that classification accuracy of unlabelled blade defect datasets improved significantly from 62.6% using only Kmeans clustering method up to 96.4% using our proposed algorithm approach. This study applies improved PSO and Kmeans algorithms towards offshore Wind Turbine Rotor Blade condition monitoring with precise detection test results enabling early-stage detection of Wind Turbine Rotor Blades defects leading to timely repairs.
更多查看译文
关键词
Wind turbine rotor blades,S-U-net,VGG16 network,deep learning,PSO algorithms,K-means
AI 理解论文
溯源树
样例
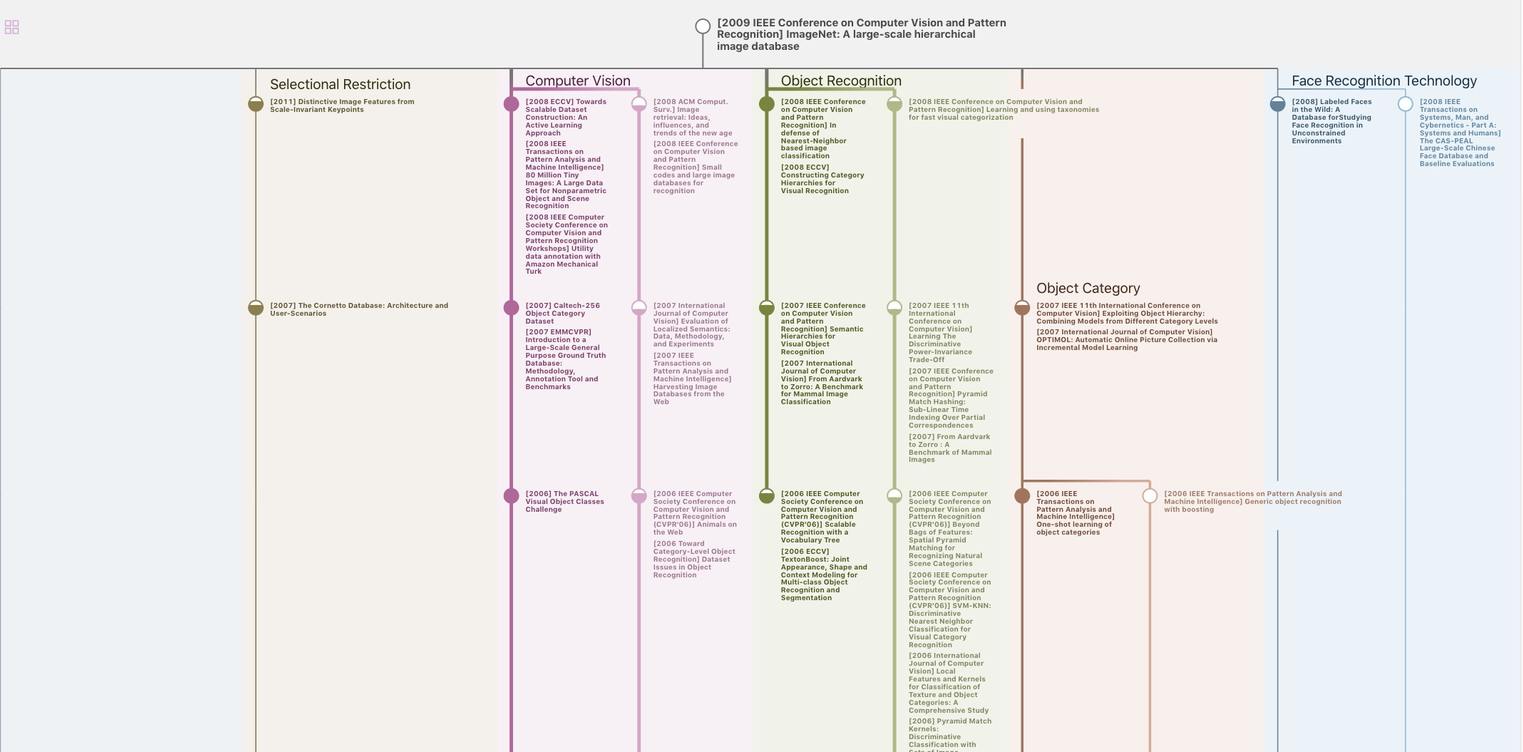
生成溯源树,研究论文发展脉络
Chat Paper
正在生成论文摘要