Against Personalised Learning
INTERNATIONAL JOURNAL OF ARTIFICIAL INTELLIGENCE IN EDUCATION(2024)
摘要
Federated learning (FL) enables distributed joint training of machine learning (ML) models without the need to share local data. FL is, however, not immune to privacy threats such as model inversion (MI) attacks. The conventional FL paradigm often uses privacy-preserving techniques, and this could lead to a considerable loss in the model’s utility and consequently compromised by MI attackers. Seeking to address this limitation, this paper proposes a robust variational encoder-based personalised FL (RVE-PFL) approach that mitigates MI attacks, preserves model utility, and ensures data privacy. RVE-PFL comprises an innovative personalised variational encoder architecture and a trustworthy threat model-integrated FL method to autonomously preserve data privacy, and mitigate MI attacks. The proposed architecture seamlessly trains heterogeneous data at every client, while the proposed approach aggregates data at the server side and effectively discriminates against adversarial settings (i.e., MI); thus, achieving robustness and trustworthiness in real-time. RVE-PFL is evaluated on three benchmark datasets, namely: MNIST, Fashion-MNIST, and Cifar-10. The experimental results revealed that RVE-PFL achieves high accuracy level while preserving data and tuning adversarial settings. It outperforms Noising before Model Aggregation FL (NbAFL) with significant accuracy improvements of 8%, 20%, and 59% on MNIST, Fashion-MNIST, and Cifar-10, respectively. These findings reinforce the effectiveness of RVE-PFL in protecting against MI attacks while maintaining the model’s utility. The source code for RVE-PFL can be found on GitHub 1.
更多查看译文
关键词
Personalisation,Personalised learning,Educational philosophy,Critical studies of AI
AI 理解论文
溯源树
样例
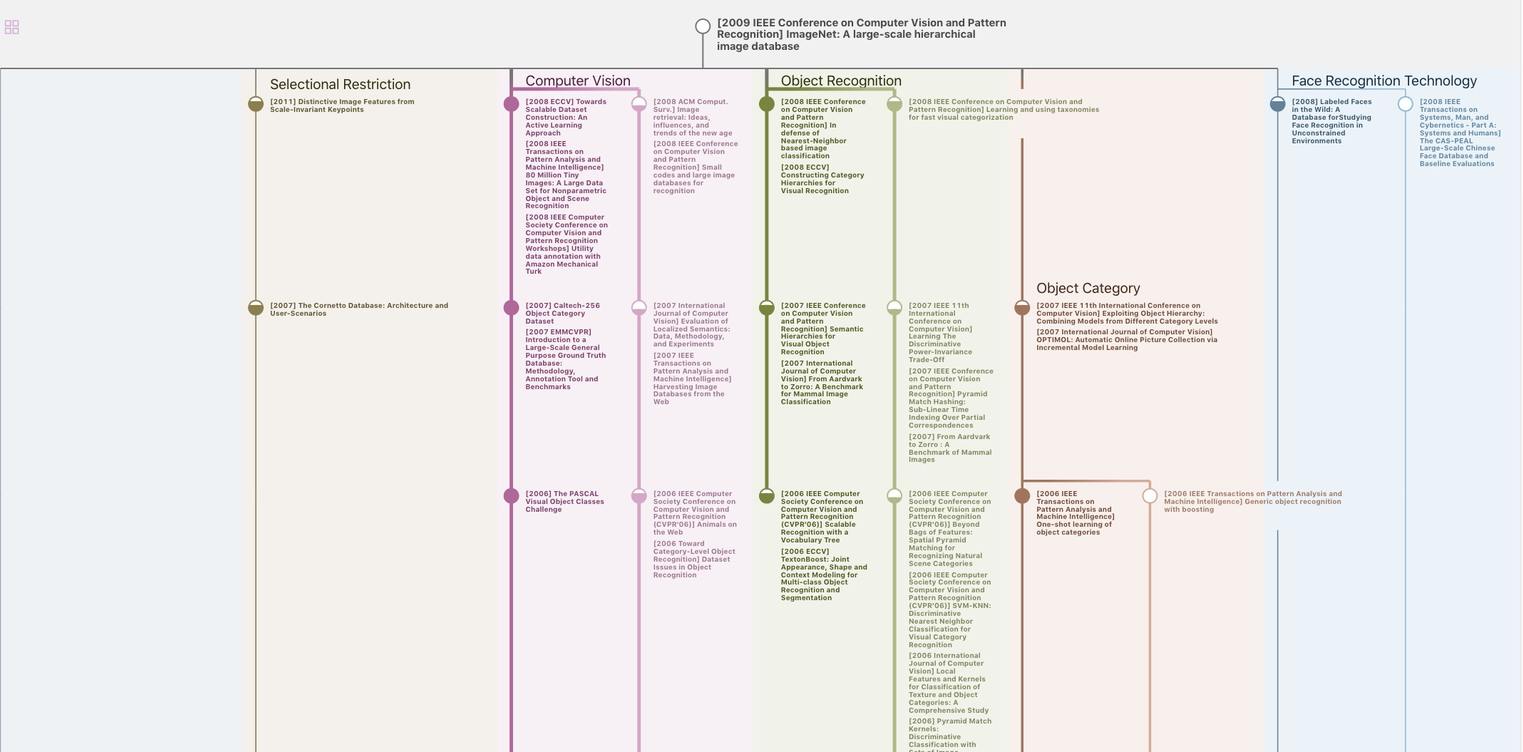
生成溯源树,研究论文发展脉络
Chat Paper
正在生成论文摘要