Robust, Fair, and Trustworthy Artificial Reasoning Systems via Quantitative Causal Learning and Explainability
ARTIFICIAL INTELLIGENCE AND MACHINE LEARNING FOR MULTI-DOMAIN OPERATIONS APPLICATIONS V(2023)
Abstract
A major concern for artificial reasoning systems to achieve robustness and trustworthiness is causal learning where better explanations are needed to support the underlying tasks. Explanations for observational datasets without ground truth presents a unique challenge. This paper aims to provide a new perspective on explainability and causality by combining the two together. We propose a model which extracts quantitative knowledge from observational data via treatment effect estimation to create better explanations through comparison and validation of the causal features with results from correlation-based feature relevance explanations. Average treatment effect (ATE) estimation is calculated to provide a quantitative comparison of the causal features to the relevant features from explainable AI (XAI). This approach provides a comprehensive approach to generate robust and trustworthy explanations via validations from both causality and XAI to ensure trustworthiness, fairness and bias detection within the data, as well as the AI/ML models for artificial reasoning systems.
MoreTranslated text
AI Read Science
Must-Reading Tree
Example
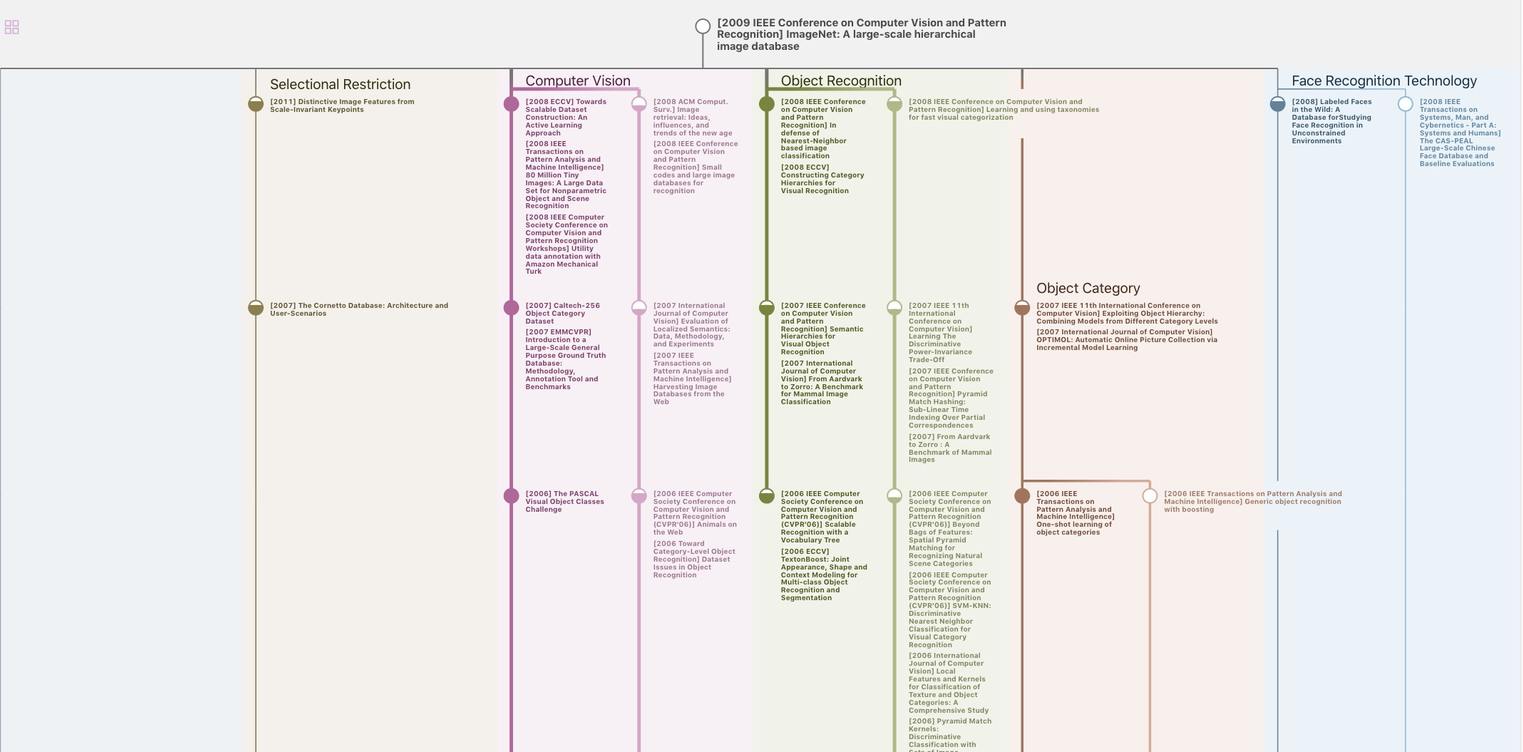
Generate MRT to find the research sequence of this paper
Chat Paper
Summary is being generated by the instructions you defined