A comparative study of TOPSIS-based GCMs selection and multi-model ensemble
INTERNATIONAL JOURNAL OF CLIMATOLOGY(2023)
摘要
General circulation models (GCMs) are developed to simulate the past climate and generate future climate predictions. In the context of global warming, their important roles in identifying possible solutions to water resources planning/management are recognized by the world. However, in actual and regional implementation, due to many factors like initial and boundary conditions, parameters and model structures and so forth, there are great variabilities and uncertainties across the future climate projections of GCMs outputs, which has attracted criticism from water resources planners. Thus, the GCMs usually must be evaluated for assessing their performances in simulating the historical observations. Currently, there are many different conclusions and opinions as to whether the optimal individual model is more advantageous or whether the combined consideration of MME works better. The purpose of this paper is to compare the advantages and disadvantages between the selection of the optimal single GCM and multi-model ensemble (MME) based on one of the more objective selection methods called TOPSIS. The results show that the performances of GCMs in simulating precipitation and temperature in different climate subregions over China are not identical. For CMIP6 GCMs simulations, the optimal precipitation GCM is CMCC-CM2-SR5 for EC, MIROC6 for SC, SWC and NWC, CESM2-WACCM for NC and NEC, and FGOALS-g3 for QTP. As for temperature, only NESM3 and BCC-ESM1 are the optimal GCM for QTP and NWC, respectively; in other subregions, MME is better than single GCM. In general, a simple arithmetic averaging approach employed to generate the MME model is not superior to the optimal GCM, although the error metric with the observed data is reduced, at the cost of a severe compression of the interannual variability.
更多查看译文
关键词
ensemble,general circulation model,ranking,selection,TOPSIS
AI 理解论文
溯源树
样例
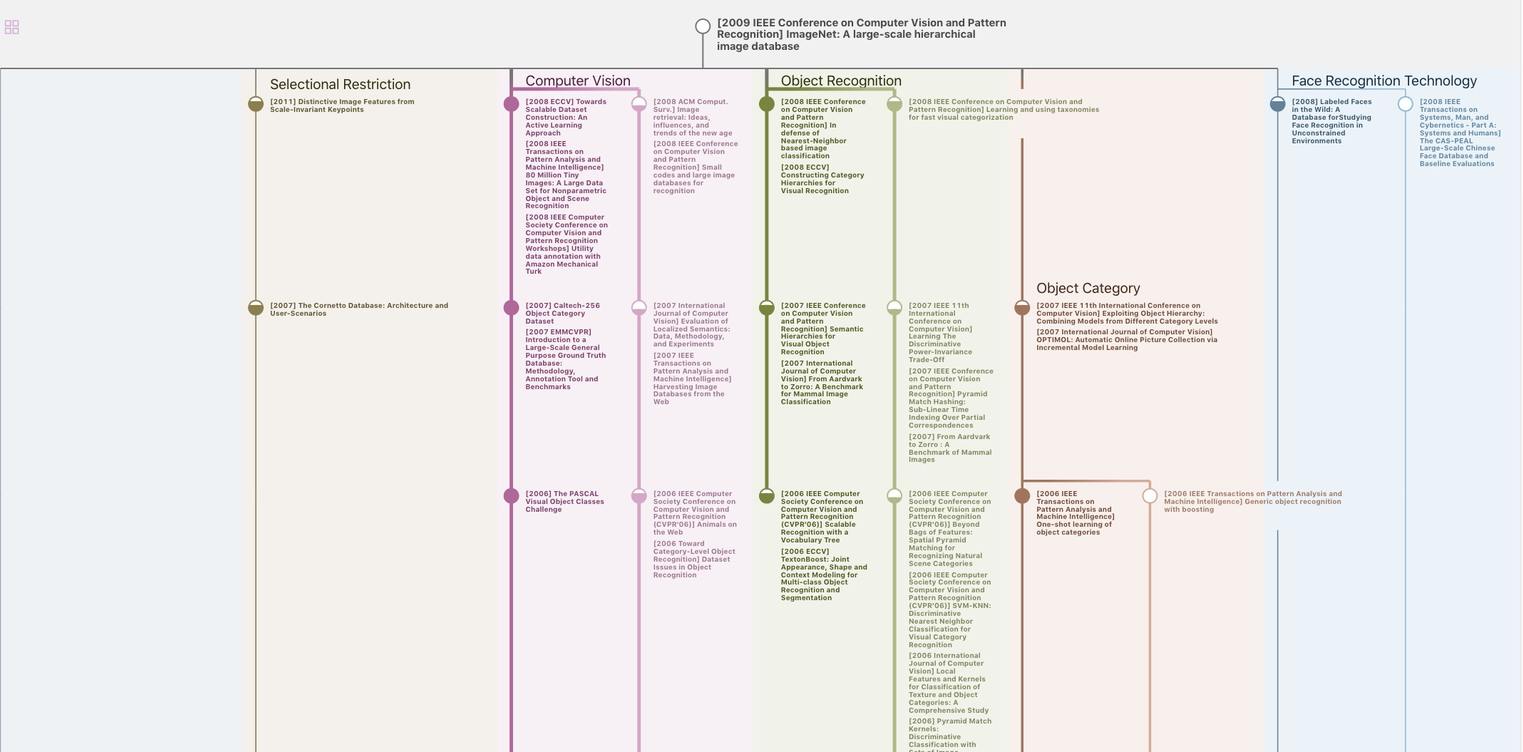
生成溯源树,研究论文发展脉络
Chat Paper
正在生成论文摘要