Block size, parallelism and predictive performance: finding the sweet spot in distributed learning
INTERNATIONAL JOURNAL OF PARALLEL EMERGENT AND DISTRIBUTED SYSTEMS(2024)
摘要
As distributed and multi-organization Machine Learning emerges, new challenges must be solved, such as diverse and low-quality data or real-time delivery. In this paper, we use a distributed learning environment to analyze the relationship between block size, parallelism, and predictor quality. Specifically, the goal is to find the optimum block size and the best heuristic to create distributed Ensembles. We evaluated three different heuristics and five block sizes on four publicly available datasets. Results show that using fewer but better base models matches or outperforms a standard Random Forest, and that 32 MB is the best block size.
更多查看译文
关键词
Distributed machine learning,distributed file system,hadoop,machine learning
AI 理解论文
溯源树
样例
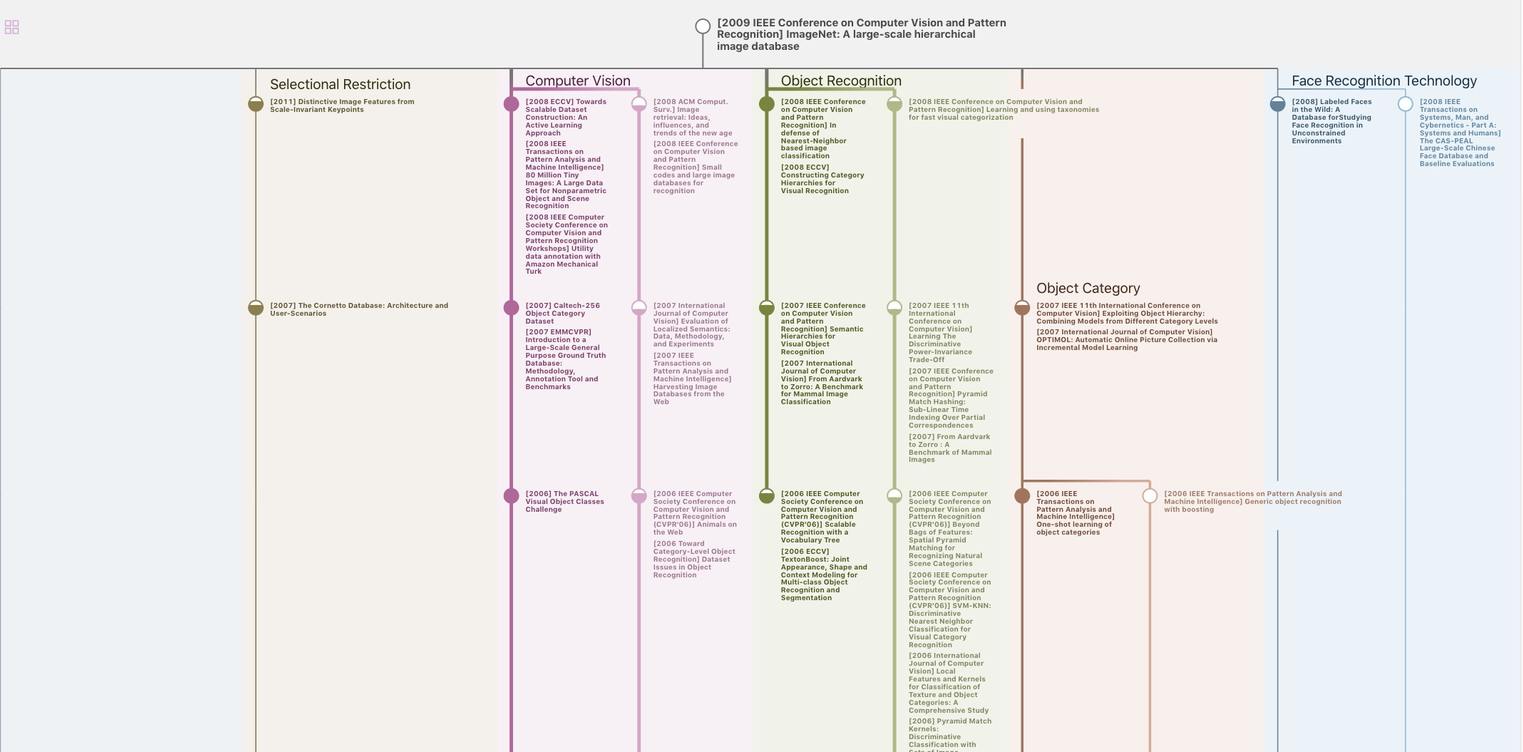
生成溯源树,研究论文发展脉络
Chat Paper
正在生成论文摘要