Study on a Low-Illumination Enhancement Method for Online Monitoring Images Considering Multiple-Exposure Image Sequence Fusion
ELECTRONICS(2023)
摘要
In order to improve the problem of low image quality caused by insufficient illumination, a low-light image enhancement method with robustness is proposed, which can effectively handle extremely dark images while achieving good results for scenes with insufficient local illumination. First, we expose the images to different degrees to form a multi-exposure image sequence; then, we introduce global-based luminance weights and contrast-based gradient weights to fuse the multi-exposure image sequence; finally, we use a bootstrap filter to suppress the noise that may occur during the image processing. We employ pertinent assessment criteria, such as the Peak Signal to Noise Ratio (PSNR), Structural Similarity (SSIM), the Average Gradient (AG), and the Figure Definition (FD), to assess how well the method enhances. Experimental results show that PSNR (31.32) and SSIM (0.74) are the highest in pretty dark scenes compared to most conventional algorithms such as MF, BIMEF, LECARM, etc. Similarly, in processing uneven illumination such as "moonlit night" images, the AG (10.21) and the FD (14.54) are at maximum. In addition, other evaluation metrics such as Shannon (SH) are optimal in the above scenarios. In addition, we apply the algorithm in this paper to the online monitoring images of electric power equipment, which can improve the image lightness while recovering the detail information. The algorithm has strong robustness in extremely dark images and natural low-light images, and the enhanced images have minimal distortion and best appearance in different low-light scenes.
更多查看译文
关键词
online monitoring images,enhancement,low-illumination,multiple-exposure
AI 理解论文
溯源树
样例
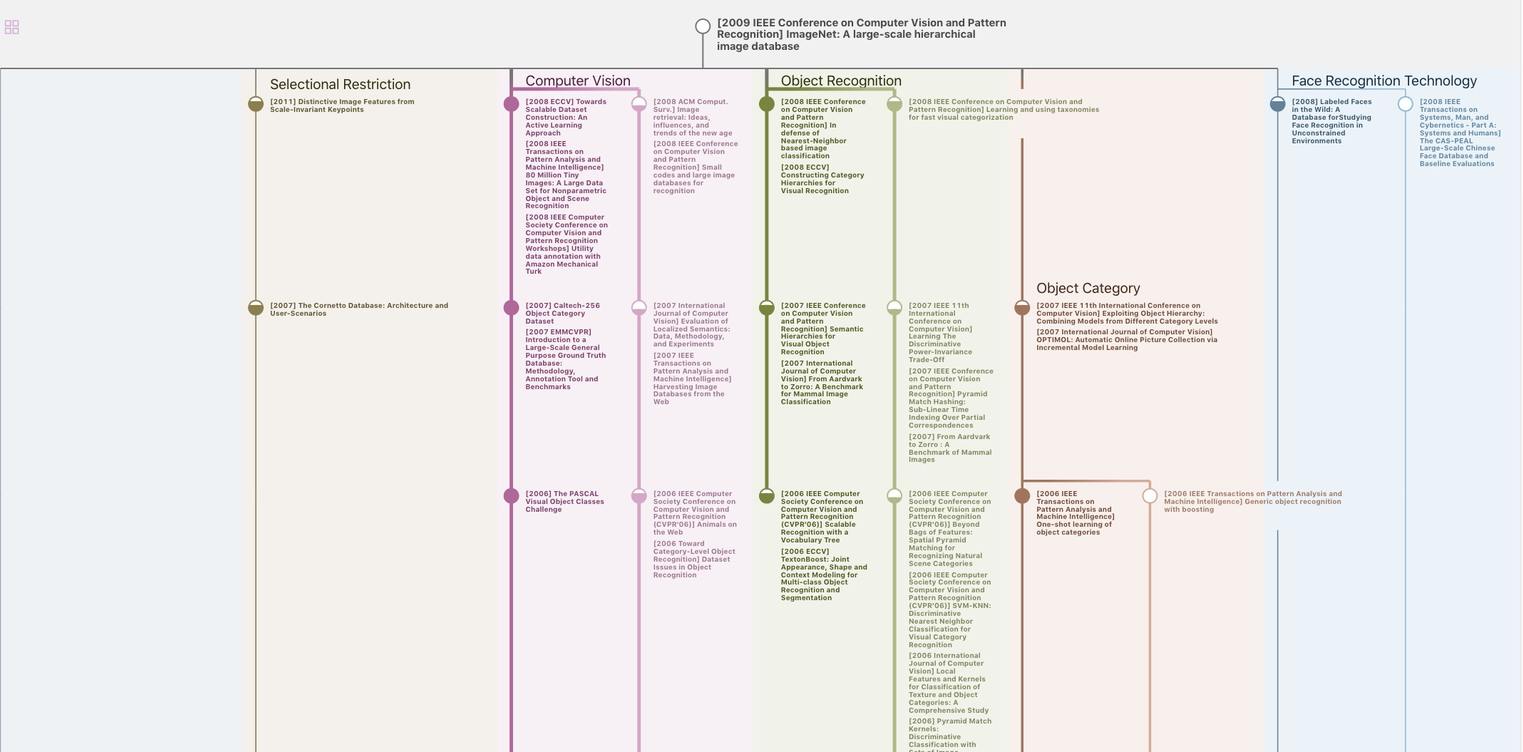
生成溯源树,研究论文发展脉络
Chat Paper
正在生成论文摘要