Optimize to generalize in Gaussian processes: An alternative objective based on the Renyi divergence
IISE TRANSACTIONS(2024)
摘要
We introduce an alternative closed-form objective function a-ELBO for improved parameter estimation in the Gaussian process (GP) based on the Renyi a-divergence. We use a decreasing temperature parameter a to iteratively deform the objective function during optimization. Ultimately, our objective function converges to the exact log-marginal likelihood function of GP. At early optimization stages, a-ELBO can be viewed as a regularizer that smoothes some unwanted critical points. At late stages, a-ELBO recovers the exact log-marginal likelihood function that guides the optimizer to solutions that best explain the observed data. Theoretically, we derive an upper bound of the Renyi divergence under the proposed objective and derive convergence rates for a class of smooth and non-smooth kernels. Case studies on a wide range of real-life engineering applications demonstrate that our proposed objective is a practical alternative that offers improved prediction performance over several state-of-the-art inference techniques.
更多查看译文
关键词
Gaussian process,Renyi divergence,convergence,engineering applications
AI 理解论文
溯源树
样例
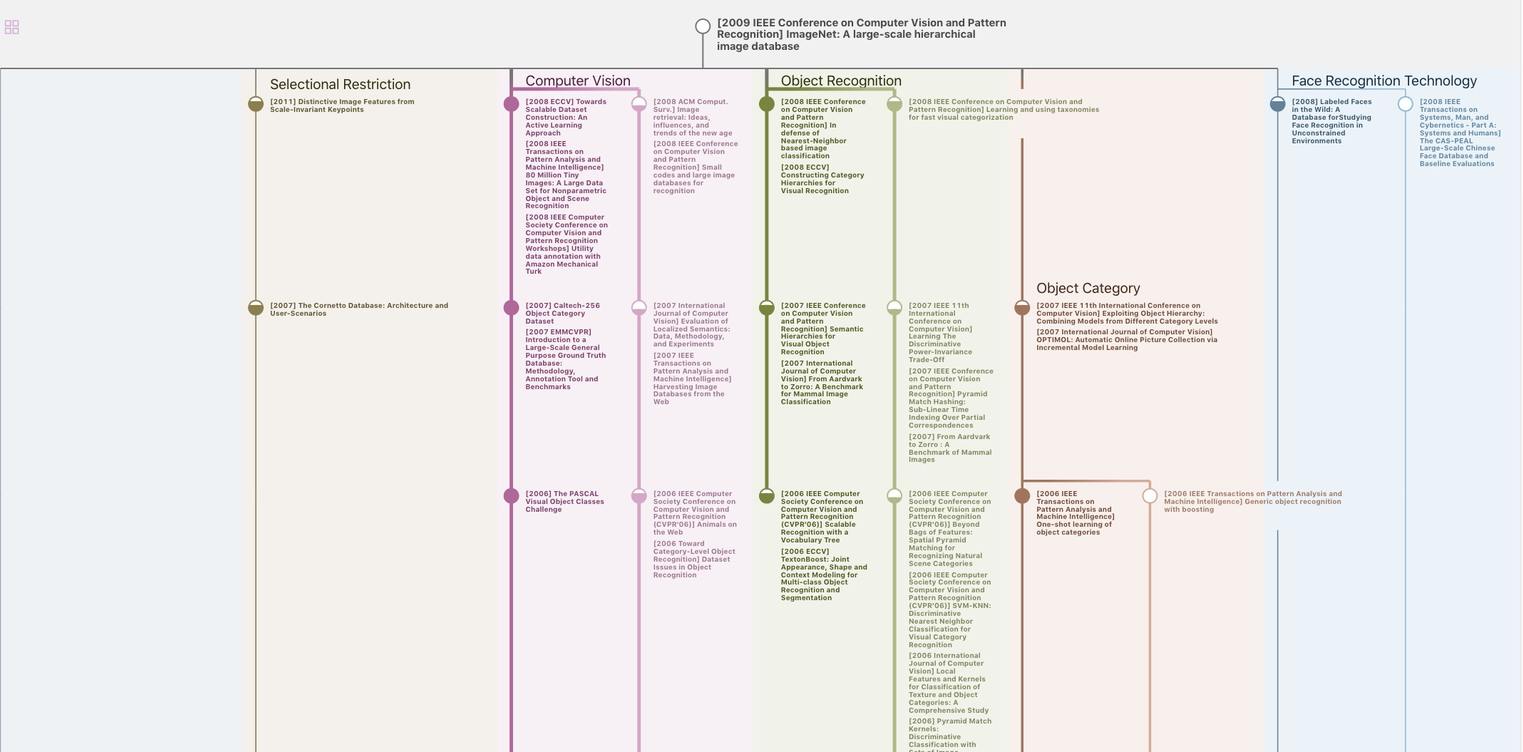
生成溯源树,研究论文发展脉络
Chat Paper
正在生成论文摘要