Deep neural network-based prediction for low-energy beam transport tuning
JOURNAL OF THE KOREAN PHYSICAL SOCIETY(2023)
摘要
Time-varying characteristics of an ion source are induced by environmental change or aging of parts inevitably, making a data-driven prediction model inaccurate. We consider non-invasively measured beam profiles as important features to represent initial beam from ion sources in real time. Beam-induced fluorescence monitor was tested to confirm change of beam properties by ion source operating conditions during a beam commissioning phase. Machine learning-based regression models were built with beam dynamics simulation datasets over a range of input parameters in the RFQ-based accelerator. Best prediction for the low-energy beam tuning was obtained by deep neural networks model. The methodology presented in the study can help develop advanced beam tuning models with non-invasive beam diagnostics in time-varying systems.
更多查看译文
关键词
RFQ-based accelerator,Beam-induced fluorescence monitor,Machine learning-based regression,Deep neural networks,Low-energy beam tuning
AI 理解论文
溯源树
样例
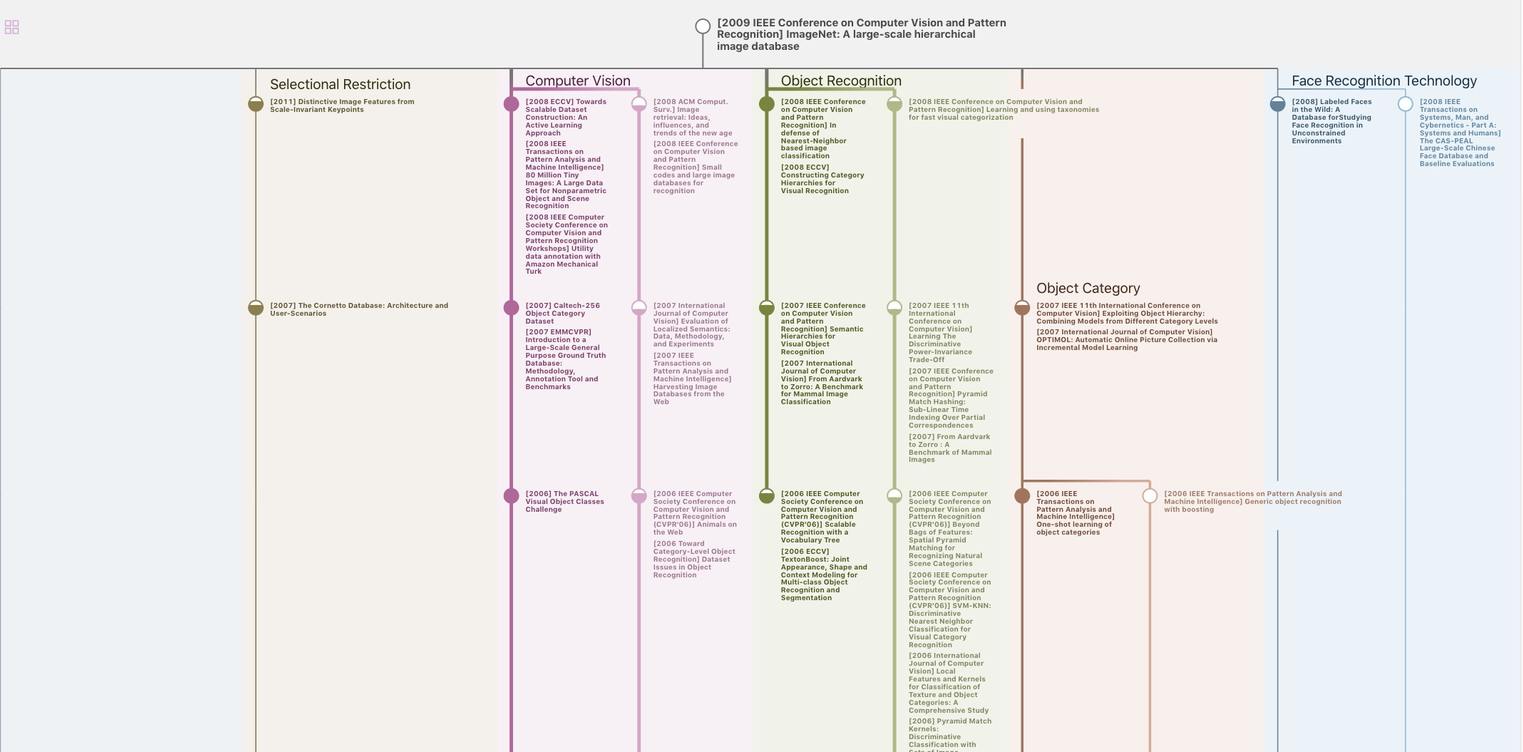
生成溯源树,研究论文发展脉络
Chat Paper
正在生成论文摘要