DGCC-Fruit: a lightweight fine-grained fruit recognition network
JOURNAL OF FOOD MEASUREMENT AND CHARACTERIZATION(2023)
摘要
In recent years, image recognition technology based on deep learning has become a research hotspot in smart agriculture. Aiming at the problem of dataset insufficient in fine-grained fruit object detection, a class mixed fine-grained fruit image object detection dataset ZFruit is constructed covering clean, natural and complex backgrounds. At the same time, in view of the current fruit image detection and recognition algorithm with high complexity, large parameters, and difficulty in high precision and lightweight detection of fine-grained fruits in different environments, this paper proposes a lightweight fruit recognition network model DGCC-Fruit based on YOLOv5. Firstly, a GC-based low-cost feature extraction network is proposed by integrating the GhostBottleneck module and the coordinate attention mechanism (CA), which enhances the fine-grained feature extraction capability; secondly, a new feature fusion network is constructed by introducing CARAFE content-aware upsampling operator to make full use of deep semantic information to improve the detection performance of fine-grained fruit images; finally, the model is further optimized by the knowledge distillation strategy. Taking the smallest-scale model as an example, the experimental results on the self-made dataset ZFruit and the public dataset VOC2007 show that our DGCC n -Fruit network has better performance than the original YOLOv5n ( ZFruit : + 2.1% mAP@.5, + 1.9% mAP@.5:.95; VOC2007 : + 5.4% mAP@.5, + 5.5% mAP@.5:.95), with a reduction of about 14% in the parameters and 11% in the model size.
更多查看译文
关键词
Fruit detection dataset,Lightweight network,Fine-grained fruit recognition,YOLOv5
AI 理解论文
溯源树
样例
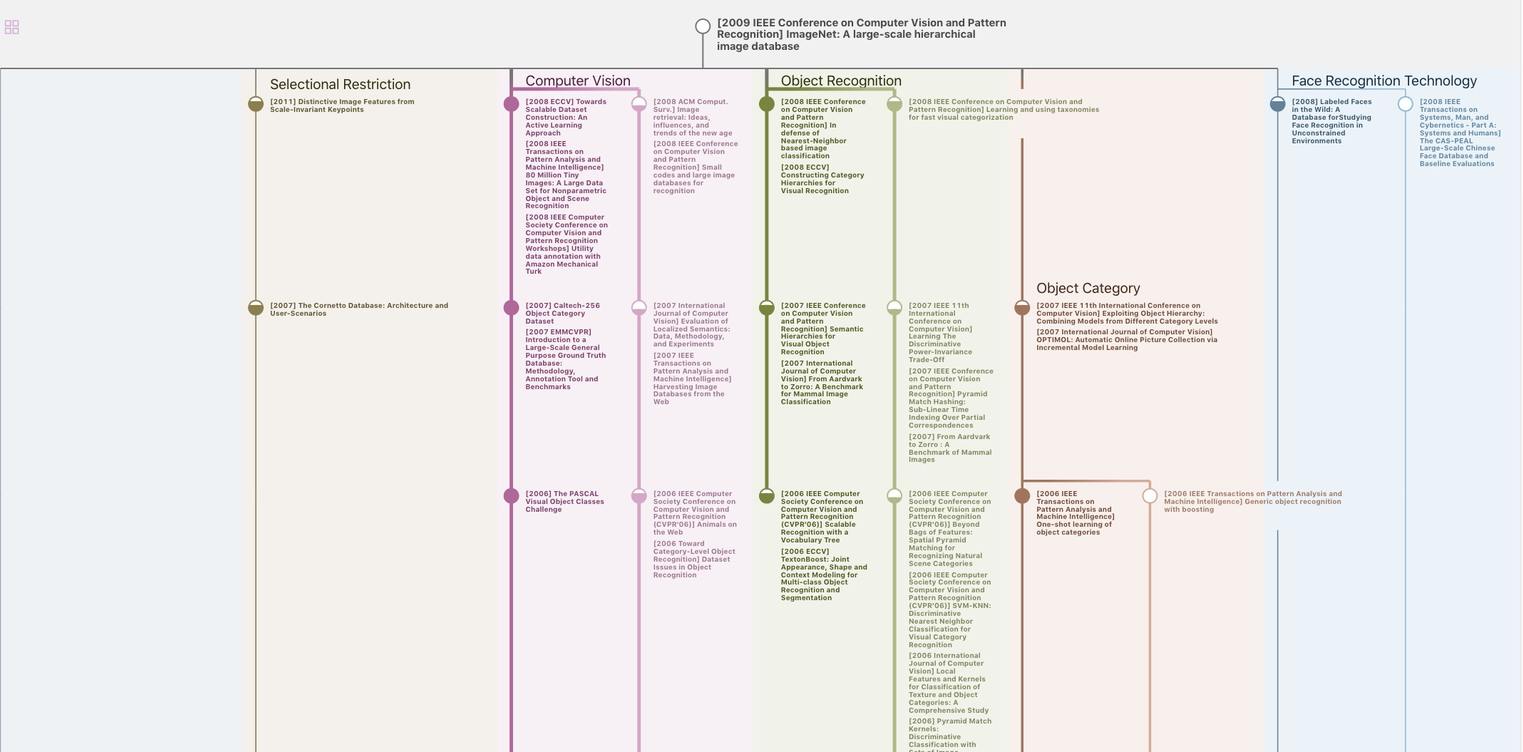
生成溯源树,研究论文发展脉络
Chat Paper
正在生成论文摘要