Nondestructive Technology for Real-Time Monitoring and Prediction of Soybean Quality Using Machine Learning for a Bulk Transport Simulation
IEEE SENSORS JOURNAL(2023)
摘要
Grain moisture content and shipping time can interfere with postharvest logistics on soybean quality. Thus, the study aimed to evaluate the use of a nondestructive technology, equipment including a mechanical-portable sampler with a hardware device and sensors for real-time monitoring of temperature, relative humidity (RH), and intergranular carbon dioxide (CO2) to predict the quality of soybean in the function of different moisture contents (11%, 14%, and 18% w.b.), sampling positions in the grain mass profile, and shipping time (0, 60, 480, and 1440 min). The monitoring of indirect quality measurement variables associated with the application of machine-learning (ML) models satisfactorily predicted the physical quality of the grain mass, over the shipping time, for the different conditions tested. The moisture content associated with time was the factor with the greatest influence on the quality of transported grains. The shipping time is the determining factor for controlling the quality of grains. Lots of soybeans harvested from crops with moisture content between 14% and 18% must not exceed 120 min of shipping time to maintain quality. It is recommended that the shipping time of grains shipped from storage units to processing industries, with moisture contents between 11% and 14%, should not exceed 840 min. The M5P and reduced error pruning tree (REPTree) algorithms were the ones that best performed the prediction of grain quality. The monitoring system and grain quality prediction has application in grain-producing farms, grain storage, the grain-processing industry, and companies providing services in grain transport. The technology contributes to grain loss reduction and conservation in the context of logistics, sustainability, and food security.
更多查看译文
关键词
soybean quality,nondestructive technology,machine learning,real-time
AI 理解论文
溯源树
样例
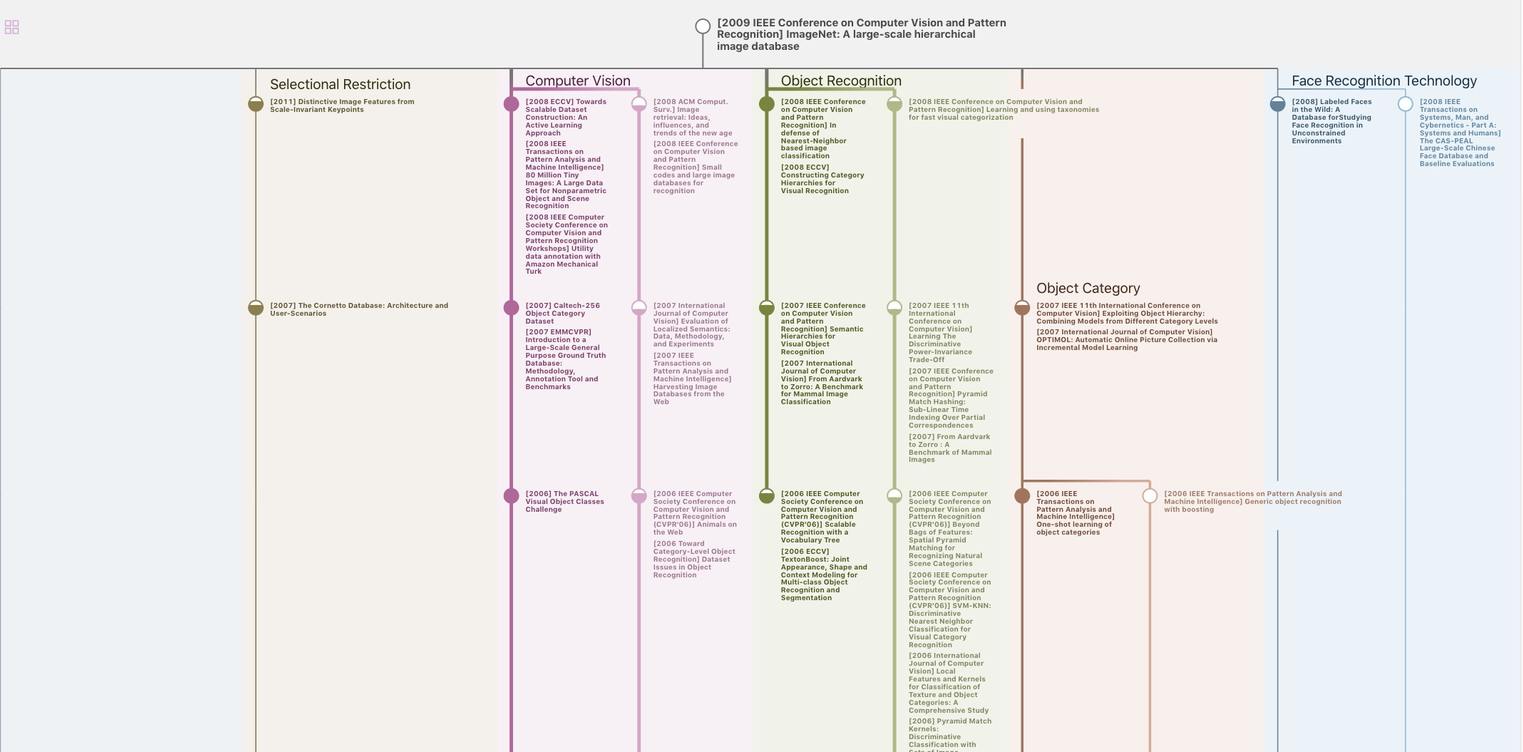
生成溯源树,研究论文发展脉络
Chat Paper
正在生成论文摘要