Blind deconvolution for multiple observed images with missing values
PACIFIC JOURNAL OF OPTIMIZATION(2023)
摘要
In this paper, we study the problem of blind deconvolution for multiple observed images with missing values. By making use of low rank tensor structure from multiple observed images, we formulate the proposed optimization model consisting of data-fitting term between the observed pixel values and blurred image pixels, low rank tensor completion for multiple observed images, and total variational regularization for the deconvoluted image. The multiple observed images form a third-order tensor where two modes are for spatial domain (under the convolution (blurring) operation) and the remaining mode is for temporal domain. Here we propose to employ the transformed tubal nuclear norm based on the transformation along temporal mode to regularize such observed low rank tensor in the model. We solve the resulting model by inexact proximal alternating minimization and show the convergence of the inexact iterations for the blind deconvolution problem. Numerical examples are reported to show the performance of the proposed model is better than that using single observed image only.
更多查看译文
关键词
tensor completion, blind deconvolution, incompleta observation, transformed tensor nuclear norm
AI 理解论文
溯源树
样例
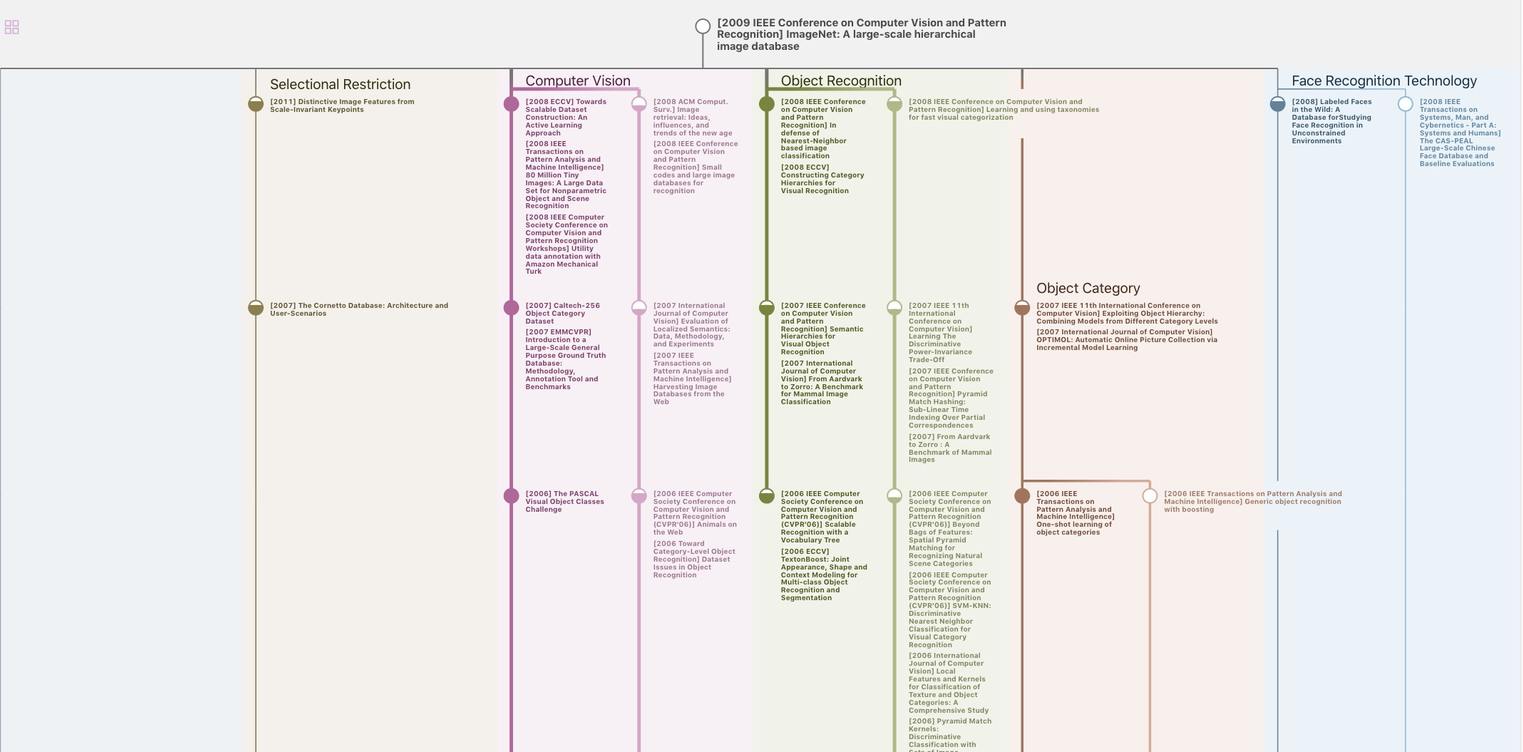
生成溯源树,研究论文发展脉络
Chat Paper
正在生成论文摘要