STMT: Spatio-temporal memory transformer for multi-object tracking
APPLIED INTELLIGENCE(2023)
摘要
Typically, modern online Multi-Object Tracking (MOT) methods first obtain the detected objects in each frame and then establish associations between them in successive frames. However, it is difficult to obtain high-quality trajectories when camera motion, fast motion, and occlusion challenges occur. To address these problems, this paper proposes a transformer-based MOT system named Spatio-Temporal Memory Transformer (STMT), which focuses on time and history information. The proposed STMT consists of a Spatio-Temporal Enhancement Module (STEM) that uses 3D convolution to model the spatial and temporal interactions of objects and obtains rich features in spatio-temporal information. Moreover, a Dynamic Spatio-Temporal Memory (DSTM) is presented to associate detections with tracklets and contains three units: an Identity Aggregation Module (IAM), a Linear Dynamic Encoder (LD-Encoder) and a memory Decoder (Decoder). The IAM utilizes the geometric changes of objects to reduce the impact of deformation on tracking performance, the LD-Encoder is used to obtain the dependency between objects, and the Decoder generates appearance similarity scores. Furthermore, a Score Fusion Equilibrium Strategy (SFES) is employed to balance the similarity and position distance fusion scores. Extensive experiments demonstrate that the proposed STMT approach is generally superior to the state-of-the-art trackers on the MOT16 and MOT17 benchmarks.
更多查看译文
关键词
Deep learning,Multi-object tracking,Transformer,Memory,Spatio-temporal
AI 理解论文
溯源树
样例
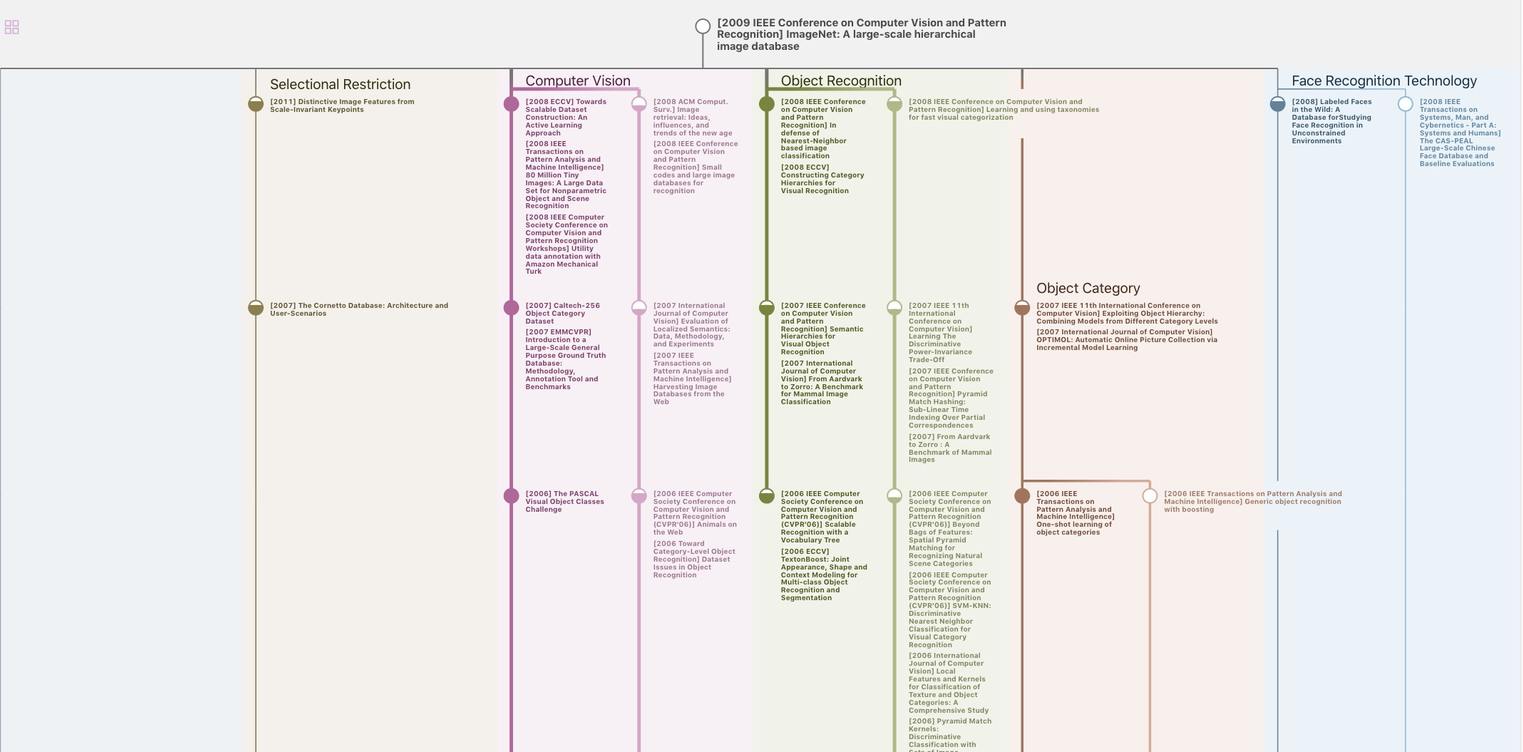
生成溯源树,研究论文发展脉络
Chat Paper
正在生成论文摘要