How Certain are Transformers in Image Classification: Uncertainty Analysis with Monte Carlo Dropout
FIFTEENTH INTERNATIONAL CONFERENCE ON MACHINE VISION, ICMV 2022(2023)
摘要
Researchers have been inspired to implement transformer models in solving machine vision problems after their tremendous success with natural language tasks. Using the straightforward architecture and swift performance of transformers, a variety of computer vision problems can be solved with more ease and effectiveness. However, a comparative evaluation of their uncertainty in prediction has not been done yet. As we know, real world applications require a measure of uncertainty to produce accurate predictions, which allows researchers to handle uncertain inputs and special cases, in order to successfully prevent overfitting. Our study approaches the unexplored issue of uncertainty estimation among three popular and effective transformer models employed in computer vision, such as Vision Transformers (ViT), Swin Transformers (SWT), and Compact Convolutional Transformers (CCT). We conduct a comparative experiment to determine which particular architecture is the most reliable in image classification. We use dropouts at the inference phase in order to measure the uncertainty of these transformer models. This approach, commonly known as Monte Carlo Dropout (MCD), works well as a low-complexity estimation to compute uncertainty. The MCD-based CCT model is the least uncertain architecture in this classification task. Our proposed MCD-infused CCT model also yields the best results with 78.4% accuracy, while the SWT model with embedded MCD exhibits Pmaximum performance gain where the accuracy increased by almost 3% with the final result being 71.4%.
更多查看译文
关键词
Vision Transformer, Uncertainty, Monte Carlo Dropout, Swin Transformer, Compact Convolutional Transformer
AI 理解论文
溯源树
样例
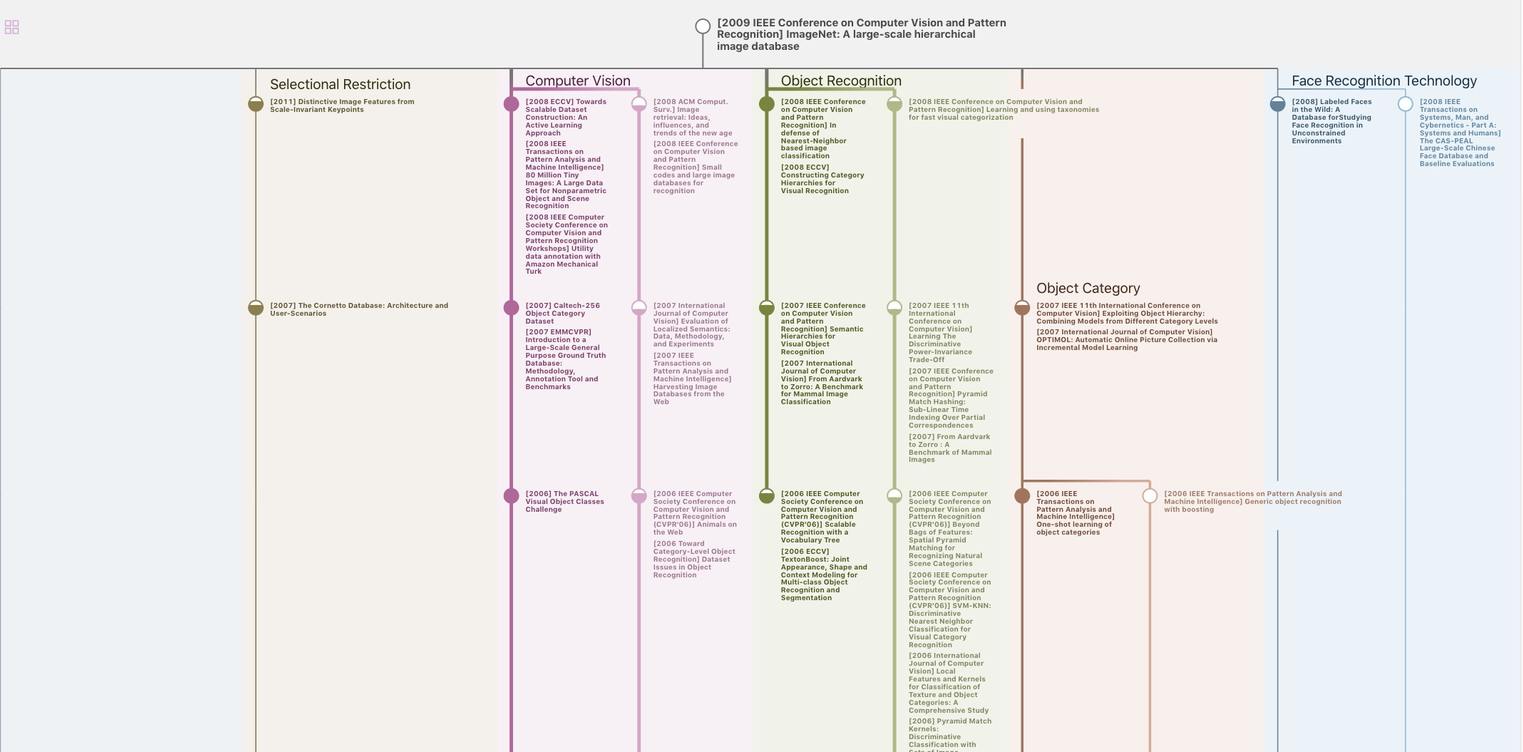
生成溯源树,研究论文发展脉络
Chat Paper
正在生成论文摘要