Rapid detection of diarrhetic shellfish poisoning toxins in Perna Viridis using NIS and D2-BO-SVM classifier
European Food Research and Technology(2023)
摘要
Perna Viridis has become a common dish in daily life. Consumption of Perna Viridis , which is contaminated with diarrhetic shellfish poisoning (DSP) toxins can cause food poisoning in humans. Therefore, there is an urgent need for a rapid and accurate method to identify Perna Viridis contaminated with DSP toxins. In this study, a non-destructive method based on near-infrared spectroscopy (NIS) is proposed to detect DSP toxin-laden Perna Viridis . Spectral data of Perna Viridis were collected in the range of 950–1700 nm for DSP toxin-laden and non-laden (non-DSP) samples. The second derivative (D2) spectral preprocessing method was used to reduce the influence of noise and light scattering. A support vector machine (SVM) model based on Bayesian optimization (BO-SVM) was constructed as a classifier for the detection of the DSP toxin-laden Perna Viridis . The average accuracy for the D2-BO-SVM classifier in detecting DSP toxin-laden Perna Viridis in 50 random tests reached 99.97%. The recognition performance of the D2-BO-SVM model was analyzed to solve the problems of different ratios of training and test datasets, small sample datasets, and unbalanced class datasets in practical applications. The experimental results show that the D2-BO-SVM model was found to be superior to BO-SVM, D2-Standard SVM, and Standard SVM. These results suggest that the combination of NIS and artificial intelligence models has great potential for the detection of the DSP toxin-laden Perna Viridis . This study provides a new method for detecting the quality and safety of shellfish, which is of great practical importance for ensuring the safety of shellfish for consumers.
更多查看译文
关键词
Non-destructive detection,Near-infrared spectroscopy,Diarrhetic shellfish poisoning toxins,Perna Viridis,Bayesian optimisation,SVM,Spectral preprocessing
AI 理解论文
溯源树
样例
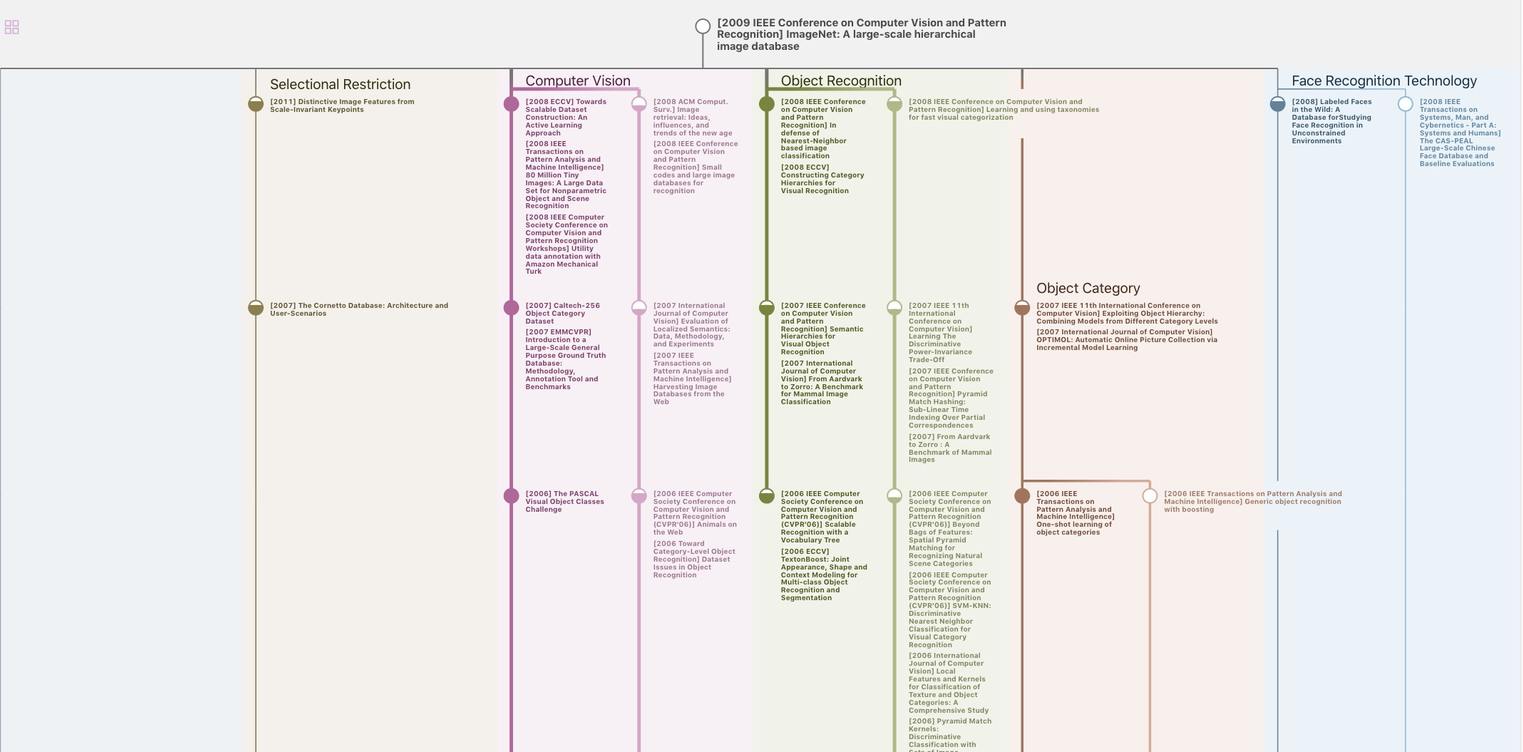
生成溯源树,研究论文发展脉络
Chat Paper
正在生成论文摘要