MetaCL: a semi-supervised meta learning architecture via contrastive learning
INTERNATIONAL JOURNAL OF MACHINE LEARNING AND CYBERNETICS(2024)
摘要
Meta learning aims to endow models with the ability to quickly learn new tasks based on existing knowledge. However, recent works have relied on complex structures and prior information to improve performance on few-shot tasks. To this end, we propose MetaCL, a meta learning architecture that uses only a traditional backbone without any priors. MetaCL takes distorted versions of an episode of samples as input and outputs predictions respectively. Besides, we introduce an unsupervised loss to minimize component redundancy and maximize variability, achieving soft-whitening and soft-alignment constraints. We evaluate MetaCL on few-shot tasks of image classification datasets CUB and miniImageNet, and experimetal results proves that MetaCL outperforms other meta-learning methods. MetaCL can be treated as a simple yet effective baseline and also be easily integrated into other few-shot models for additional performance gains.
更多查看译文
关键词
Meta learning,Few-shot learning,Contrastive learning,Semi-supervised learning,Image classification
AI 理解论文
溯源树
样例
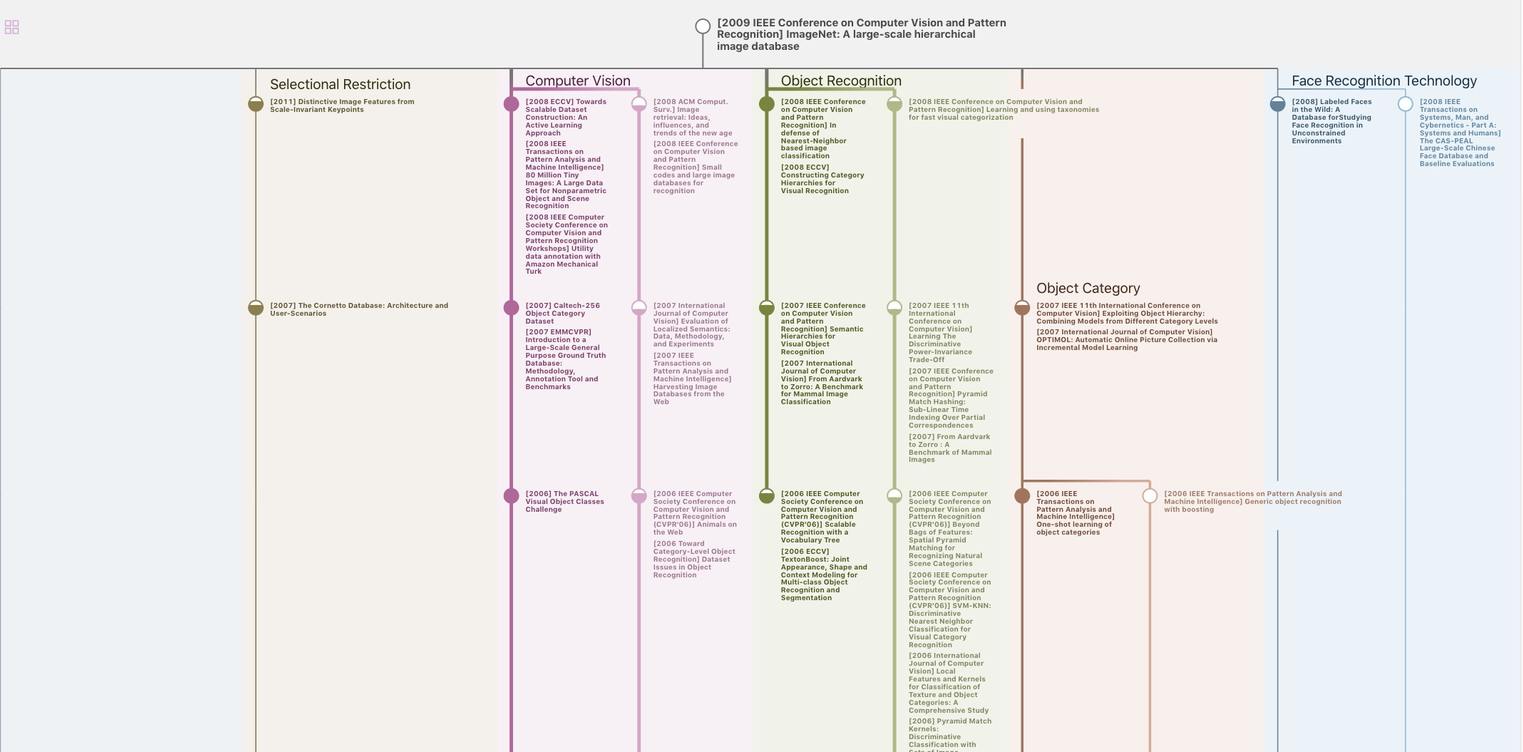
生成溯源树,研究论文发展脉络
Chat Paper
正在生成论文摘要