Simultaneous mapping of nearshore bathymetry and waves based on physics-informed deep learning
COASTAL ENGINEERING(2023)
Abstract
This paper uses physics-informed neural networks (PINNs) to simultaneously determine nearshore water depths and wave height fields based on remote sensing of the ocean surface with limited or sparse measurements. Two methods that integrate the knowledge of water wave mechanics and fully connected neural networks are introduced. The first method utilizes observed wave celerity fields and scarce measurements of wave height as training data. The model performance was examined with linear waves over an alongshore varying barred beach and nonlinear waves over an alongshore uniform barred beach. The second method uses scarce wave height and water depth measurements as training points, and the model performance was investigated with water waves over a circular shoal and the alongshore varying barred beach. One advantage of applying PINNs to solve bathymetry inversion problems is that wave height and bathymetry can be simultaneously estimated by PINN models. Thus, the impact of wave amplitude dispersion on depth inversion in nonlinear wave systems can be considered without measuring the entire wave height field. Overall, this study demonstrates the potential of the inverse PINN model as a promising tool for estimating nearshore bathymetry and reconstructing wave fields using observations from different remote sensing platforms.
MoreTranslated text
Key words
nearshore bathymetry,waves,deep learning,simultaneous mapping,physics-informed
AI Read Science
Must-Reading Tree
Example
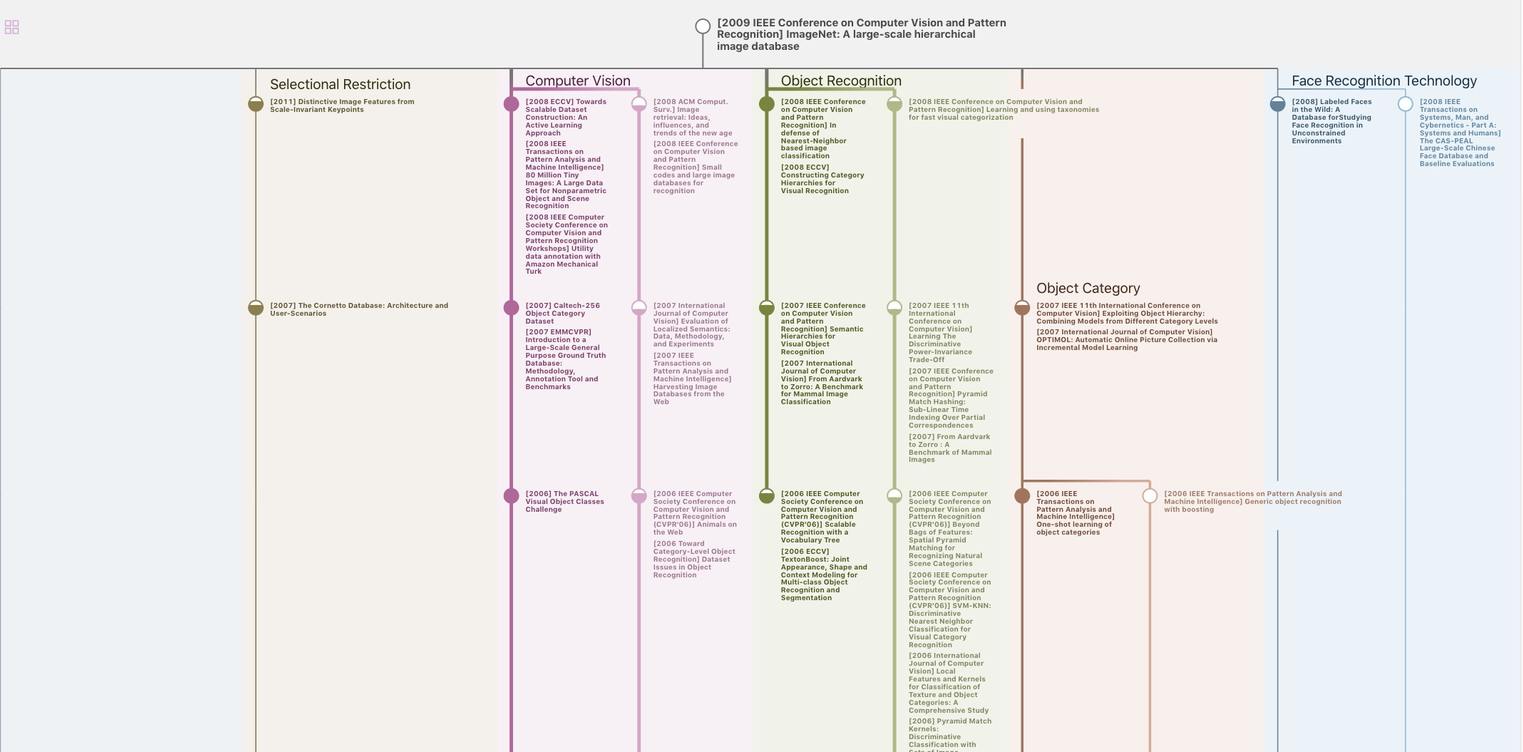
Generate MRT to find the research sequence of this paper
Chat Paper
Summary is being generated by the instructions you defined