Uncovering Patterns in Dairy Cow Behaviour: A Deep Learning Approach with Tri-Axial Accelerometer Data.
ANIMALS(2023)
摘要
The accurate detection of behavioural changes represents a promising method of detecting the early onset of disease in dairy cows. This study assessed the performance of deep learning (DL) in classifying dairy cows' behaviour from accelerometry data acquired by single sensors on the cows' left flanks and compared the results with those obtained through classical machine learning (ML) from the same raw data. Twelve cows with a tri-axial accelerometer were observed for 136 ± 29 min each to detect five main behaviours: standing still, moving, feeding, ruminating and resting. For each 8 s time interval, 15 metrics were calculated, obtaining a dataset of 211,720 observation units and 15 columns. The entire dataset was randomly split into training (80%) and testing (20%) datasets. The DL accuracy, precision and sensitivity/recall were calculated and compared with the performance of classical ML models. The best predictive model was an 8-layer convolutional neural network (CNN) with an overall accuracy and F1 score equal to 0.96. The precision, sensitivity/recall and F1 score of single behaviours had the following ranges: 0.93-0.99. The CNN outperformed all the classical ML algorithms. The CNN used to monitor the cows' conditions showed an overall high performance in successfully predicting multiple behaviours using a single accelerometer.
更多查看译文
关键词
convolutional neural network, machine learning, precision livestock farming, animal welfare
AI 理解论文
溯源树
样例
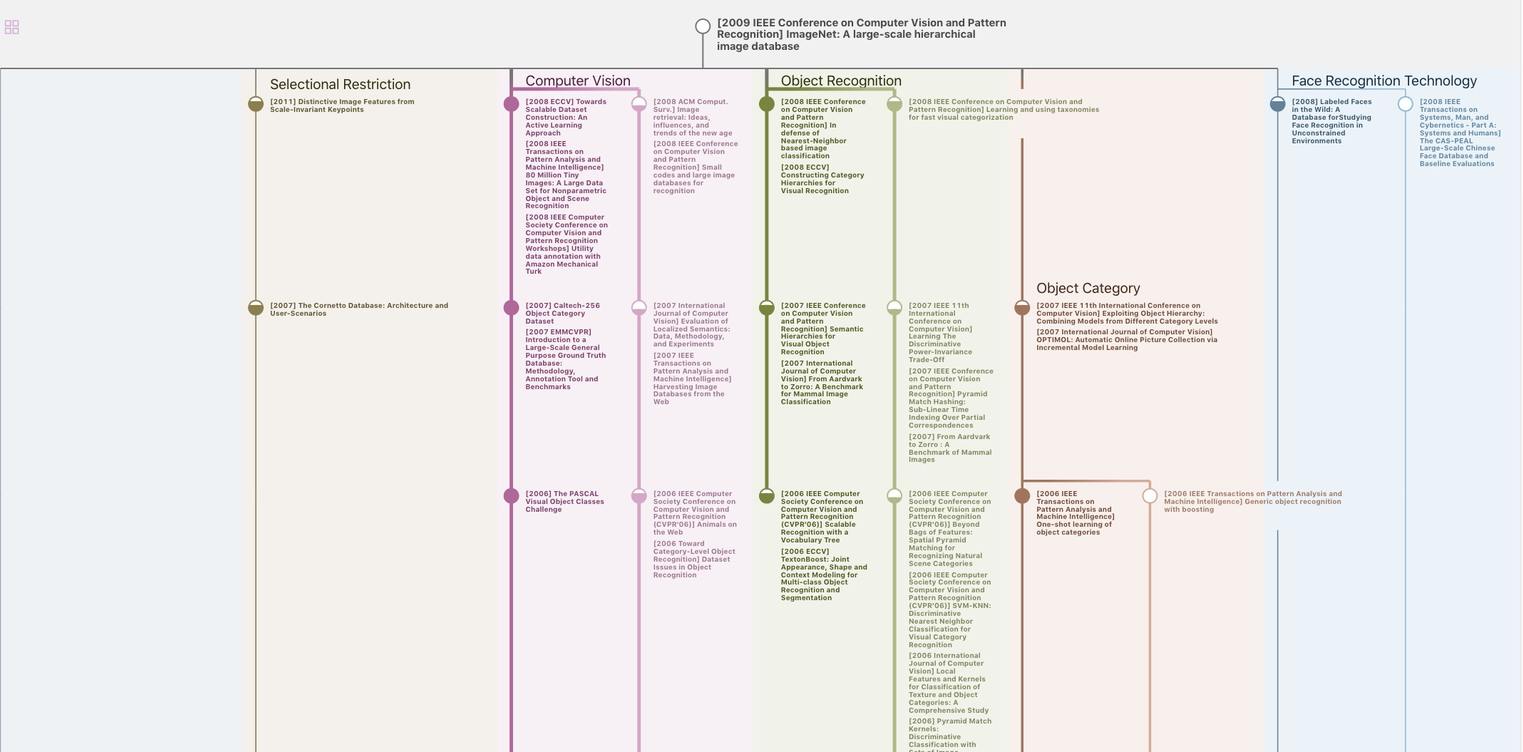
生成溯源树,研究论文发展脉络
Chat Paper
正在生成论文摘要