Multimodule Deep Learning Scheme for Elastic Wave Inversion of Inhomogeneous Objects With High Contrasts
IEEE TRANSACTIONS ON GEOSCIENCE AND REMOTE SENSING(2023)
摘要
Fault detection is an essential component of seismic interpretation and plays a crucial role in industrial processes. However, it is also one of the main challenges, especially in delineating faults in 3-D seismic data. Recently, the rapidly developing technology, deep learning, has proven to be a powerful tool for this task. A number of neural networks have been proposed for this purpose by regarding 3-D fault detection as a semantic segmentation task. To further enhance the effectiveness of the deep learning methods, we propose a novel network architecture, named R2SE-Unet, to solve the 3-D segmentation problem. In the neural network, we design a recurrent residual-SE convolutional unit (RRCU-SE) that integrates the residual learning and squeeze-excitation module to store the information in 3-D seismic data. This component promotes the spread of 3-D volumetric information and aids in learning spatial dependencies in 3-D images. In addition, to reduce the impact of insufficient spatial resolution resulting from the base architecture of U-net, we add an attention unit between skip connection operations. These two new units enable our R2SE-Unet to exploit semantic information more accurately in the feature maps. After many experiments on region-based loss functions and distribution-based loss functions, we also propose a novel loss function, which takes the advantage of generalized dice (GDice) loss and balanced binary cross entropy (b-BCE) loss, named GDice-bce, to effectively train R2SE-Unet. Although only synthetic seismic data samples are used to train the network parameters, our R2SE-Unet could produce more reliable fault feature maps on field seismic data than two other conventional fault detection neural networks. Thus, the proposed neural network is easy to train and reliably works for seismic fault interpretation on field seismic data.
更多查看译文
关键词
Fault detection, Petroleum, Three-dimensional displays, Convolution, Task analysis, Deep learning, Neural networks, 3-D fault detection, compound loss function, deep learning, field data, recurrent network
AI 理解论文
溯源树
样例
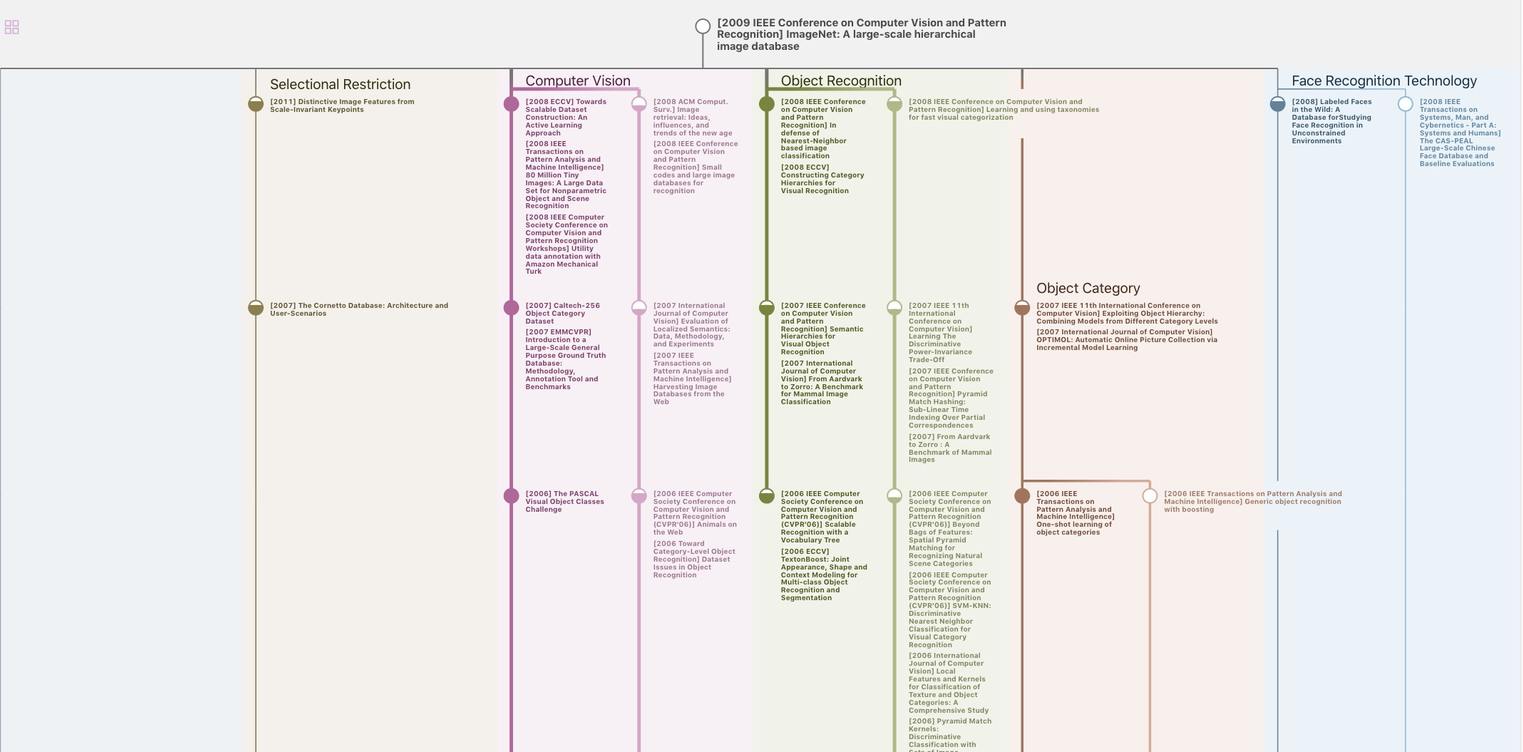
生成溯源树,研究论文发展脉络
Chat Paper
正在生成论文摘要